Boost Your Decision Skills with Analytics

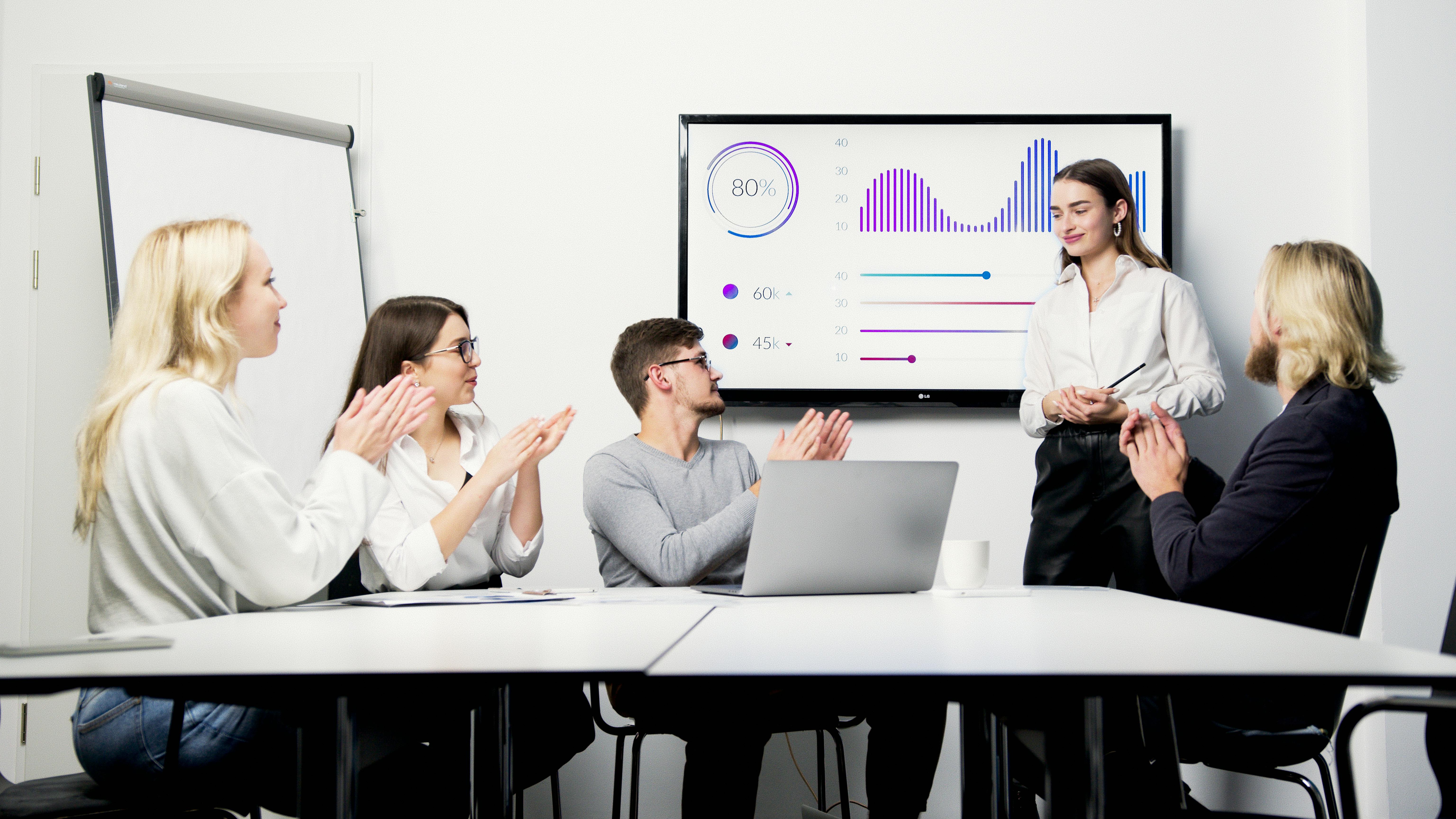
Making informed decisions has never been more crucial in a world driven by data. From businesses seeking competitive advantages to individuals navigating daily choices, analytics empowers us to uncover insights that shape outcomes. But analytics isn't just about crunching numbers—it's about understanding the stories data tells and using them to create meaningful change.
This article explores the four pillars of analytics—descriptive, diagnostic, predictive, and prescriptive—and how each helps decode the past, diagnose the present, and design the future. Whether you're an aspiring data professional or simply looking to sharpen your decision-making skills, this journey through analytics will inspire you to harness the full potential of data-driven thinking.
Types of Analytics: From Understanding the Past to Shaping the Future
In this overview, you can see the four main types of analytics: descriptive, diagnostic, predictive, and prescriptive analytics. Each type of analytics has its main focus.
Descriptive Analytics
Descriptive analytics focuses on summarizing and visualizing the data. This type of analytics can be used to get to know the data based on its variables. Variables are the characteristics of interest we have measured or observed—for example, temperature in a weather dataset. Descriptive analytics can also be used to investigate relationships or patterns in the data, for example, whether specific groupings occur or one variable is closely related to another. Lastly, descriptive analytics can be used to prepare for more advanced techniques, like building a model.
Common techniques
In its most basic form, a common technique of descriptive analytics consists of calculating descriptive statistics, like averages and maximum or minimum values of variables. These help us answer questions like 'What is the most common value?' or 'How spread out are the values?' Visualizing the data is also helpful in getting to know the characteristics of the data, in particular, in seeing patterns, groupings, or other relationships in the data. Outlier detection helps to find values that are very different from the majority, which can help to find errors. Exploratory data analysis, or EDA, is a more advanced and comprehensive form of descriptive analytics.
Exploratory data analysis
The focus of exploratory data analysis, or EDA, as the name implies, lies in exploring the data: assessing its main characteristics, finding relationships, patterns, or groups, and suggesting hypotheses for future analysis. Often, it is contrasted with hypothesis testing, which is more formal and specific, while EDA looks at data in a broad, open way to discover something unknown. It combines techniques to gain a comprehensive data picture, including basic descriptives, and more advanced techniques, such as cluster analysis, to find groupings. It also places a strong emphasis on visualization to gain additional insights. It can be used to prepare for further study and offer valuable insights, such as discovering data problems.
Case study: ice cream sales
Suppose you work for an ice cream company and are tasked with analyzing ice cream sales. You are particularly interested in which flavor sells best. Using descriptive analytics, you could count the number of sales per flavor and, if needed, separate the numbers per store or month. The insights gained from this can then be used to limit the number of flavors to the most popular or select new flavors to sell based on the characteristics of the already popular flavors.
2. Diagnostic analytics
Just like a doctor diagnosing a patient to find the underlying disease, diagnostic analytics aims to find the root causes of events. It is similar to descriptive analytics but focuses more on the 'why' question.
Why use diagnostic analytics?
Diagnostic analytics can be used to find potential causes of events or reasons for behaviors, investigate causal relationships, and, most importantly, the results of a diagnostic analysis can be used to suggest solutions based on the identified causes. While diagnostic analytics can provide evidence for possible causes, it can be challenging to prove that there is an actual causal relationship. This is because a third factor can cause a statistical relationship between variables we don't know yet or even by coincidence. This is why domain knowledge is a crucial asset in diagnostic analytics, to help assess whether the results are plausible. Additional analysis and setting up experiments can also help strengthen the case for causality.
Common techniques
A common technique for diagnostic analytics is drill-down analysis, which consists of moving from general summary data to examining underlying data, for instance, from a sales report showing rapid revenue growth and digging deeper to identify the stores and products that contributed most to the increase. Other standard techniques include correlation and regression analysis, which can tell us if variables are closely related; hypothesis testing, when the probable causes are theorized beforehand; and root cause analysis, which we'll discuss further in the next slide. Some of these techniques use similar methods to descriptive analytics, and you will often see similar descriptive statistics and visualizations. The difference is that diagnostic analysis is more directed at underlying causes, while descriptive analytics offers a broader view of an event.
Root cause analysis (RCA)
Root Cause Analysis or RCA is a formal set of steps used to look beyond superficial causes that have a direct effect, named 'contributing factors,' by investigating these factors further to identify their causes. This drill-down process continues until the 'root causes' are identified. How far this process goes is typically determined by domain knowledge. An RCA process consists of five steps: first, define the specific event under consideration. Second, all relevant data related to this event must be collected. Third, the contributing factors are determined based on an analysis of the data. Fourth, drill down to root causes. Finally, recommend solutions based on the identified root causes.
Case study: Airline customer satisfaction
Suppose you are working for an airline and are tasked with determining why the customer satisfaction rate is declining. Using diagnostic analytics, you could identify some potential causes by finding and grouping keywords in complaints and ranking the most common categories. You could also investigate connections between the complaint categories and other data you might have, such as waiting times or delays. This is typically based on domain knowledge. The results of this analysis can then be used to suggest potential solutions for the most common categories of complaints.
3. Predictive analytics
After learning all about descriptive and diagnostic analytics, it is time to look at predictive analytics.
Descriptive and diagnostic analytics are concerned with the past or, at most, the present; predictive and prescriptive analytics look at the future. Predictive analytics focuses on identifying outcomes and their probability, helping us investigate what will most likely happen based on what we know from the data.
Why use predictive analytics?
Predictive analytics can assist in anticipating the most likely outcomes of an event, such as predicting whether a machine will soon fail based on maintenance data so that the company can organize repairs in advance. It is also often used to forecast how a specific trend will continue, for example, to predict stock prices or the evolution of a pandemic. Usually linked to future events, predictive analytics can also be used to indicate something that is not yet known in the present. For example, based on symptoms, predict which disease a patient most likely has or indicate whether it is fraudulent based on the characteristics of a transaction. Either way, predictions are always associated with a degree of uncertainty, even more so if we look further away from the data we have.
Common techniques
One of the most common techniques used in predictive analytics is machine learning, a set of techniques where computers learn from existing patterns in data to make predictions on new data. A distinction can be made between classification and regression. Classification predicts categories or membership to a group, like predicting whether a customer will cancel their subscription shortly. In contrast, regression predicts values, such as predicting housing prices based on the characteristics of a neighborhood. Time series forecasting focuses on predicting future values over time, like predicting sales revenue for the next quarter. Predictive text analytics is a sub-group of predictive techniques that concentrate on text, typically used to predict to which category a text belongs, for example, whether an email is spam or not. Regardless of the exact technique used, there are some common steps all predictive models use, which we'll discuss in more detail on the following slide.
Predictive modeling
The first step consists of defining what we want to predict. Second, collect and prepare the data about what we want to predict. Typically, the data is split into a training set for building the model and a minor test set for evaluation. The data is then used in the third step to build and train the model until it provides accurate predictions on the training data. When the model is ready, the projections are interpreted and evaluated on the test data using pre-determined metrics like accuracy and the percentage of correct predictions. Usually, multiple metrics are used to evaluate a predictive model thoroughly. Finally, the model can be further fine-tuned if necessary.
Case study: World Cup winner
Before any great sports event, many try to predict the eventual winner, from animals with predictive ability to supercomputers. The computer models use data like team ratings, player ratings, current rankings, estimated difficulty of the matches, and so on to predict who will most likely win and other things like how likely a team will reach the knockout phase or the chances of getting to the final.
4. Prescriptive analytics
Now that you've learned about descriptive, diagnostic, and predictive analytics, it is time to learn about the fourth type of analytics: prescriptive analytics.
Analytics overview
Prescriptive analytics builds on the results of predictive analytics. While predictive analytics allows us to identify potential outcomes and their probabilities, prescriptive analytics aims to take this further and take action on the predicted result. As can be deduced from the name, with prescriptive analytics, we are trying to answer the question, "What is the 'prescribed' or recommended course of action?" and, by doing so, select the best action given the outcome we want to achieve and taking into account related benefits and drawbacks.
Why use prescriptive analytics?
The primary purpose of prescriptive analytics is to help us decide what best to do. In doing so, it provides the following benefits: the ability to make informed, data-driven decisions, optimization of processes, and mitigation of risks associated with particular outcomes.
Common techniques
Standard techniques used in prescriptive analytics include rule-based systems, which generate a set of rules or decision logic to get the best outcome. Domain knowledge, machine learning models, or combinations generate these rules. In reinforcement learning, an algorithm learns to achieve a particular objective or optimize an outcome by receiving positive and negative feedback when running through a set of actions. Scenario analysis and simulation consist of running through a set of pre-determined scenarios or simulating multiple outcomes to help select the decision that leads to the best result. And lastly, the recommendation engine is one of the most well-known techniques. We'll discuss this in further detail on the next slide.
Recommendation engine
You've probably seen this often: when shopping online or watching a movie on Netflix, you're recommended what to buy or watch next. Behind these recommendations is a recommendation engine. It first looks at the products you've viewed or purchased in the past and uses this as data to predict what other products you would be interested in purchasing. Then, it provides specific recommendations based on these predicted interests.
Case study: fraud detection
Suppose you work in a bank and transactions on a particular account have been flagged as possibly fraudulent. How should we proceed? Block the account? Call the customer to double-check the transactions. Have a fraud analyst do a manual check. Each of these options comes with its risks and benefits. We want to avoid fraud losses but avoid the negative impact of completely blocking a client's account. We may also have limited resources. For example, the fraud analyst can only check a limited number of cases daily. Prescriptive analytics can assist in these kinds of considerations and decisions.
Mastering the Analyst's Lens
The journey through analytics—descriptive, diagnostic, predictive, and prescriptive—reveals its transformative potential to turn raw data into actionable insights. Each type of analytics builds upon the last, offering a progressive understanding of events, their causes, and their probable outcomes while empowering organizations and individuals to act decisively.
By mastering the analyst's lens, you gain technical skills and a strategic edge in decision-making. Whether uncovering hidden patterns, predicting future trends, or prescribing optimal actions, analytics equips you to navigate complexity with clarity and confidence. The future belongs to those who can see beyond the numbers and tell compelling stories through data.
Subscribe to my newsletter
Read articles from Queen Carine directly inside your inbox. Subscribe to the newsletter, and don't miss out.
Written by

Queen Carine
Queen Carine
As a Data Analyst, I'm passionate about turning data📊📈 into actionable insights that drive smarter decisions. On this journey, I share my experiences, challenges, and the tools I’m learning along the way. Join me as I explore the world of data analytics and uncover new ways to make data work for you.