Data as a Product, the DataChef way

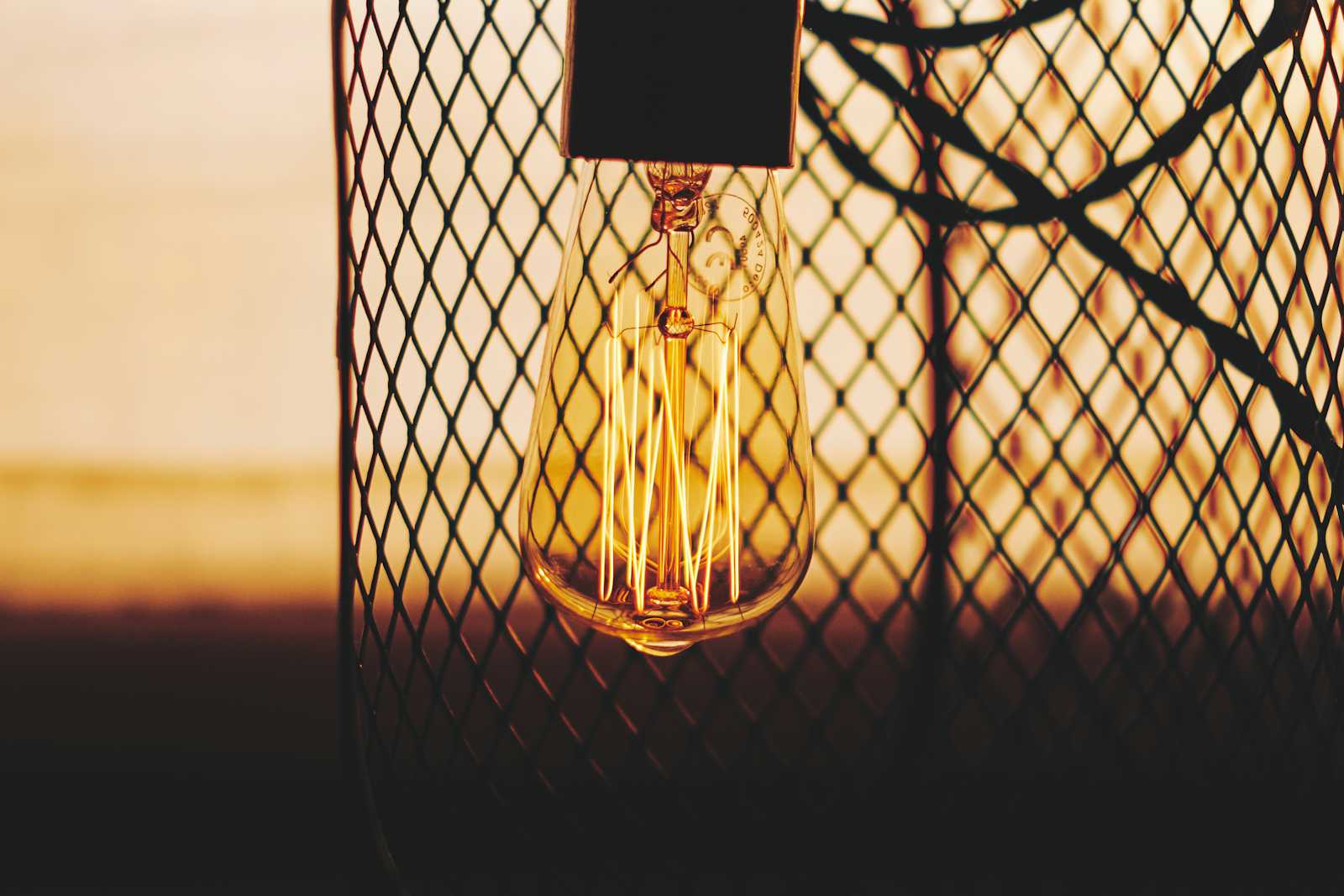
At DataChef, we know that treating data as a product can be a powerful principle, whether your organization is embracing the Data Mesh architecture or not. We also feel that, while it’s very easy to talk about ‘Data as a Product’, it’s actually quite challenging to navigate its implementation.
Data as a Product typically requires big changes in order to be implemented successfully. Teams must rethink how they handle data, starting from a different governance and ownership model. Also, balancing access and security adds complexity to the picture.
One of our customers had the ambition to transform their data ecosystem into a real Data Marketplace, where internal and external users can find and access the data products they need. To support this vision, they wanted to embrace the principle of Data as a Product, and asked DataChef for help. Here’s how we crafted a tailored path to discover and describe data products.
Understand the value flow
A good product is valuable to its users, and the same applies to datasets. It is important to start this exercise by identifying the end users of data as a product, and working backwards from the problems they are trying to solve. This ensures you remain connected with the value flow you are enabling through your data products.
We conducted initial interviews with a curated list of key representatives in the organization, representing the different roles that need access to datasets. This initial exploration was useful to understand the overall sentiment towards data, such as a perceived lack of trust in data-powered solutions, and the high friction when it comes to usability and accessibility of the data. Also, it allowed us to have a clear picture of the interaction patterns between producers, consumers, and platform teams.
Having now a high-level overview of the goals of data users, we were ready to go to the next step and work with a larger group of people.
Involve the right people
We spent a large portion of the effort profiling and segmenting our audience. Leveraging frameworks such as Jobs To Be Done, we grouped our users together, breaking team and role boundaries.
Most importantly, we didn’t just ask their team lead or PO, “What does your team need?” - we made sure we were actually involving the people on the ground.
The activities were tailored with a collaborative approach in mind. Instead of a traditional PowerPoint presentation, we positioned ourselves as facilitators, asking the right questions and then letting the audience craft an answer together in small groups and present it to the rest of the people involved.
This ensured that all voices would be heard, and that the real pain points would emerge. Also, the audience became invested in the solution to their problems, because it was not mandated on them: they owned it in the first place.
Choose winning properties
By now, we knew what the biggest data-related pain points were. It was then a simple exercise to connect them to key properties of Data as a Product.
For example, being able to discover the datasets available at any given point in time was a big struggle for every user, regardless whether they are a Data Scientist, a ML Engineer, or a Data Analyst. And the biggest item in the wishlist for Product Owners was the ability to spot issues with the data and be notified before their consumers are affected in production.
From there, we were able to design the core properties for this implementation of Data as a Product, for example discoverability and observability, and we worked with engineering teams and architects to translate these properties into a repeatable framework.
To recap, here’s what we have achieved so far:
Identified the biggest pain points for teams who build data-enabled solutions.
Crafted a set of properties for the implementation of Data as a Product that effectively solved those pain points.
Promoted such properties to a new standard for the development of data products.
The Data Marketplace didn’t even exist yet, but it could now be built on solid foundations.
Design the interactions between teams
For a successful implementation of the Data as a Product principles, technology and organization must evolve hand in hand. For data to be treated as a product, you need dedicated product managers who own the ROI (return on investment) and the business case for building a new product. These product managers should treat their consumers as customers, and monitor metrics to measure adoption, satisfaction, and accessibility of their assets.
Zooming out, it’s important to design clear interaction patterns and engagement models between teams. A great inspiration for this is the Team Topologies framework. Make sure that your data product managers are aligned to the business domains, and don’t break down their scope into multiple teams just based on the technology they are using. Stream-aligned teams need complete ownership over their scope, and their interactions with other teams, such as platform teams or enabling teams, need to be designed in order to accelerate their development, and not to put more obstacles in their way.
If you want to read more on how to design data platforms for success, you can read our blog post.
Subscribe to my newsletter
Read articles from Davide Rovati directly inside your inbox. Subscribe to the newsletter, and don't miss out.
Written by
