Unraveling the Complexity of BEND-VLM: Enhancing Fairness in Vision-Language Models
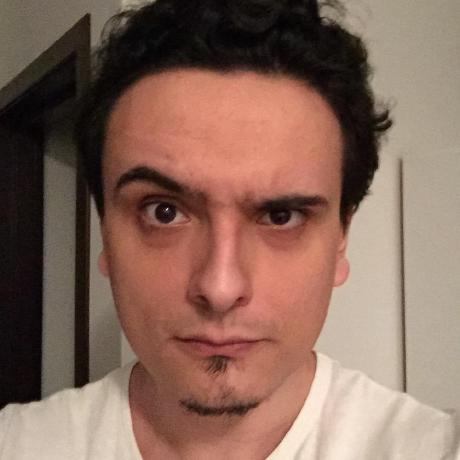
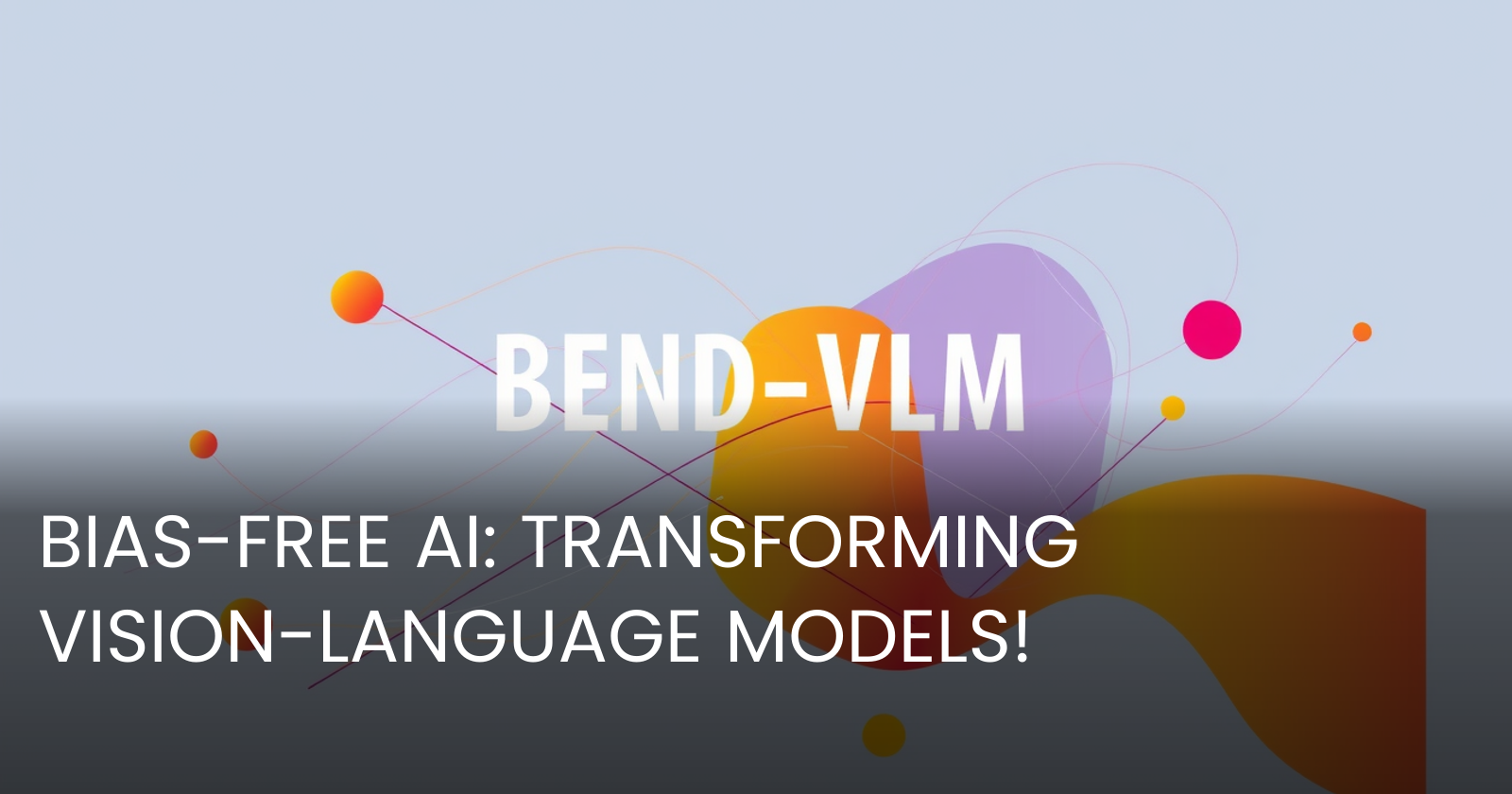
Scientific strides often entail wading through a sea of technical complexity to unveil innovations with the potential to transform industries. One such advancement comes from a notable paper that introduces BEND-VLM, a method designed to improve fairness in vision-language models (VLMs). This intriguing method holds promising applications for companies looking to fine-tune their AI-driven processes, ensuring inclusivity and fairness while maintaining efficiency and accuracy.
- Arxiv: https://arxiv.org/abs/2411.04420v1
- PDF: https://arxiv.org/pdf/2411.04420v1.pdf
- Authors: Marzyeh Ghassemi, Thomas Hartvigsen, Maanas Sharma, Eileen Pan, Kimia Hamidieh, Haoran Zhang, Walter Gerych
- Published: 2024-11-07
Key Insights from the Paper: Tackling Bias in AI
What are the Main Claims in the Paper?
The paper's primary claim centers on the issue of biases inherent in vision-language models, which are often reflective of societal stereotypes present in the training data. These biases can lead to misclassification and negative stereotypes, which the authors aim to mitigate using BEND-VLM, a novel debiasing method.
What are the New Proposals/Enhancements?
BEND-VLM stands out as a test-time debiasing approach that does not require finetuning the VLM itself, thereby avoiding the pitfalls of accuracy degradation commonly seen with such processes. The method is innovative in its ability to apply debiasing in a flexible manner tailored to each specific input, which enhances its applicability in online, open-set environments.
How Can Companies Leverage This Paper?
Companies can harness BEND-VLM to deploy AI systems free from embedded biases, ensuring fairness in automated decision-making processes. For instance, organizations in sectors like healthcare, recruitment, and law can use this method to mitigate risks associated with algorithmic bias. Additionally, the technique can be adapted to improve consumer experience in personalized advertising, shopping assistants, and customer service—industries where bias-free interaction can drive customer loyalty and brand reputation.
What are the Hyperparameters? How is the Model Trained?
The BEND-VLM approach utilizes a two-step debiasing process, emphasizing query-specific nonlinear transformations. However, specific hyperparameters such as projections and embeddings are adjusted without altering the VLM's weights, thus maintaining the model's usability across diverse tasks without extensive retraining.
What are the Hardware Requirements to Run and Train?
The process described does not require hefty hardware resources for retuning the models, as it hinges on existing VLMs and operates effectively without needing to adjust the model’s existing weight parameters. This ensures scalability and adaptability across different platforms equipped with standard computational capabilities.
What are the Target Tasks and Datasets?
BEND-VLM targets various vision-language tasks including classification, retrieval, and image captioning. It utilizes datasets with diverse attribute representations—such as gender or race—to derive fairer representations across these dimensions.
How Do the Proposed Updates Compare to Other SOTA Alternatives?
The method significantly reduces bias compared to existing approaches like Orth-Proj and DebiasCLIP. It was evaluated using various datasets, showing marked improvements in fairness metrics such as KL divergence and max skew, while maintaining competitive accuracy levels.
What Are the Conclusions? What Can Be Improved?
The study concludes by highlighting BEND-VLM’s efficiency in reducing bias while consistently delivering robust performance across tasks. Yet, the challenge remains to further enhance this approach to accommodate expanding scenarios in VLM applications, leading to even greater inclusivity and accuracy in future implementations.
Conclusion
As businesses increasingly rely on AI-driven insights, the imperative to ensure fairness and eliminate bias grows stronger. BEND-VLM opens a window to new possibilities, allowing companies to innovate responsibly while unlocking revenue and optimizing processes. This pioneering approach is a step forward in creating more equitable AI systems that serve diverse communities with respect and integrity.
Subscribe to my newsletter
Read articles from Gabi Dobocan directly inside your inbox. Subscribe to the newsletter, and don't miss out.
Written by
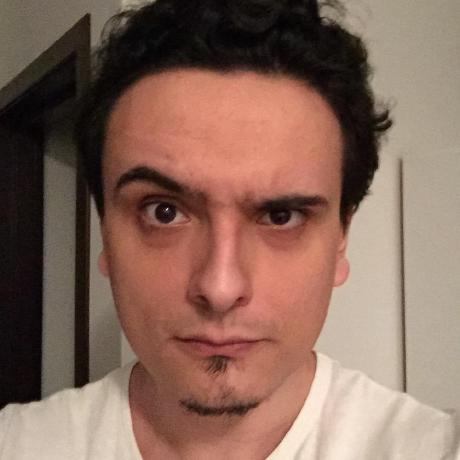
Gabi Dobocan
Gabi Dobocan
Coder, Founder, Builder. Angelpad & Techstars Alumnus. Forbes 30 Under 30.