Enhancing Investment Analysis with AI: Optimizing Multi-Agent Collaboration
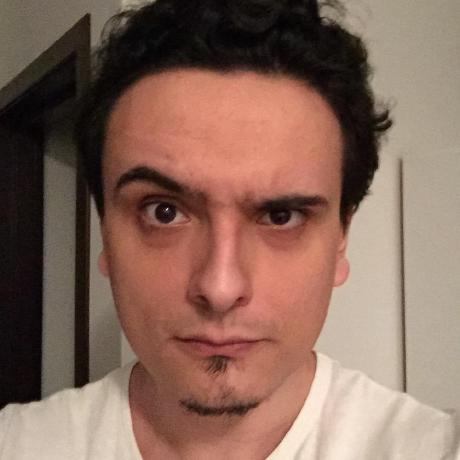

In the realm of financial analysis and investment decision-making, the integration of artificial intelligence (AI) is reshaping the landscape. A recent scientific paper provides a deep dive into a novel multi-agent collaboration system tailored for financial research. This system leverages the collective capabilities of AI agents to enhance decision-making processes in complex financial environments. In this blog post, we’ll dissect the key findings and proposals outlined in the paper, and explore how businesses can harness these advancements to optimize their operations and unlock new revenue streams.
- Arxiv: https://arxiv.org/abs/2411.04788v1
- PDF: https://arxiv.org/pdf/2411.04788v1.pdf
- Authors: Sean Xin Xu, Kunpeng Zhang, Hongyang Yang, Shangkun Che, Neng Wang, Xuewen Han
- Published: 2024-11-07
Main Claims of the Paper
The paper's central thesis is the effectiveness of a multi-agent AI system over traditional single-agent models in financial investment research. The research advocates that a collaborative approach among AI agents enhances accuracy, efficiency, and adaptability. The study focuses on three core financial tasks: fundamental analysis, market sentiment analysis, and risk analysis, utilizing data from the 2023 SEC 10-K forms of companies listed on the Dow Jones Index.
New Proposals and Enhancements
Innovative Collaboration Frameworks
The paper proposes several innovative multi-agent architectures, including vertical, horizontal, and hybrid collaboration structures. These frameworks are distinguished by varying implementations of leadership and cooperation among agents. For simpler tasks like fundamental and sentiment analysis, horizontal and hybrid structures, which promote inter-agent communication, perform better. For more complex risk analysis tasks, a vertical structure with a clear leader is advantageous.
Information Flow Analysis
A detailed analysis of information flow between agents is conducted, highlighting how different collaboration models produce varied outcomes. This understanding aids in selecting the optimal structure for given financial tasks.
Optimal Agent Groups
The study identifies the optimal multi-agent configurations for diverse sub-tasks. Ensembles of agent groups were shown to outperform single-agent setups in investment research tasks, proving particularly effective in target price prediction and decision-making.
Leveraging the Paper for Business Growth
New Product and Business Ideas
Businesses can utilize this multi-agent AI system to enhance financial analysis capabilities. By integrating these systems, companies can offer more accurate and comprehensive financial insights, potentially leading to smarter investment strategies and better risk management.
Revenue Opportunities
The ability to automate complex analysis tasks allows financial service providers to manage a larger portfolio with fewer human resources, increasing potential revenue without scaling staff proportionately. Companies can also sell these insights or tools as services to interested clients or financial analysts.
Technical Insights: Hyperparameters, Model Training, and Hardware Requirements
The study utilized Large Language Models (LLMs) like GPT-4, leveraging a variety of tools for data retrieval and analysis. Specific hyperparameters or training protocols are not detailed in the summary, but the use of such advanced models typically requires substantial computational resources. For training, efficient hardware setups involving GPUs or TPUs are crucial to managing the computational demands of LLMs.
Target Tasks and Datasets
The target tasks consist of fundamental, sentiment, and risk analysis, centered on data extracted from the 2023 SEC 10-K forms of 30 Dow Jones companies. Each task utilizes different AI agent configurations to assess which performs best under various circumstances.
Comparison with SOTA Alternatives
Compared to State Of The Art (SOTA) alternatives, the proposed multi-agent system shows superior performance in tasks like price prediction and decision-making accuracy. Single-agent models fall short in flexibility and collaborative analysis capabilities.
Conclusions and Areas for Improvement
The paper concludes with a strong recommendation for the adoption of multi-agent AI systems in financial analysis for comprehensive insights. However, it acknowledges the need for further improvements, such as ensuring continuous information flow and exploring debates in group structures to enhance performance. The sub-optimal structure selection strategy could also be refined for global optimization of tasks.
In conclusion, the adoption of multi-agent AI systems represents a significant opportunity for businesses in the financial sector. By leveraging these technologies, companies can streamline operations, enhance decision-making processes, and open new avenues for innovation and revenue growth. As AI continues to evolve, its role in transforming industries will only become more pronounced, making early adoption a crucial step for forward-thinking businesses.
Subscribe to my newsletter
Read articles from Gabi Dobocan directly inside your inbox. Subscribe to the newsletter, and don't miss out.
Written by
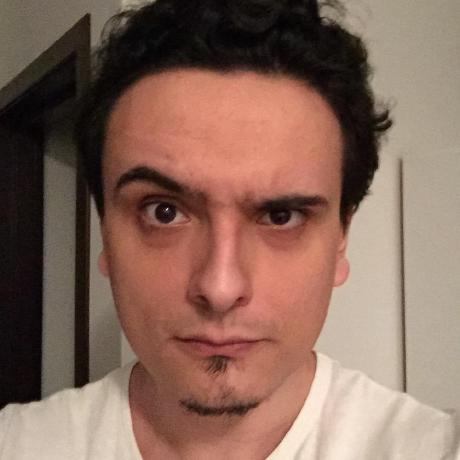
Gabi Dobocan
Gabi Dobocan
Coder, Founder, Builder. Angelpad & Techstars Alumnus. Forbes 30 Under 30.