Unveiling DomainGallery: Innovating Few-Shot Domain-Driven Image Generation
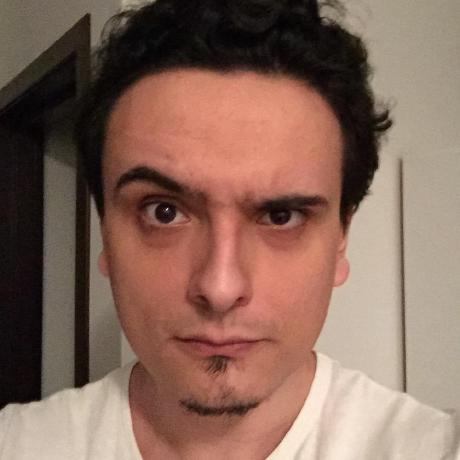
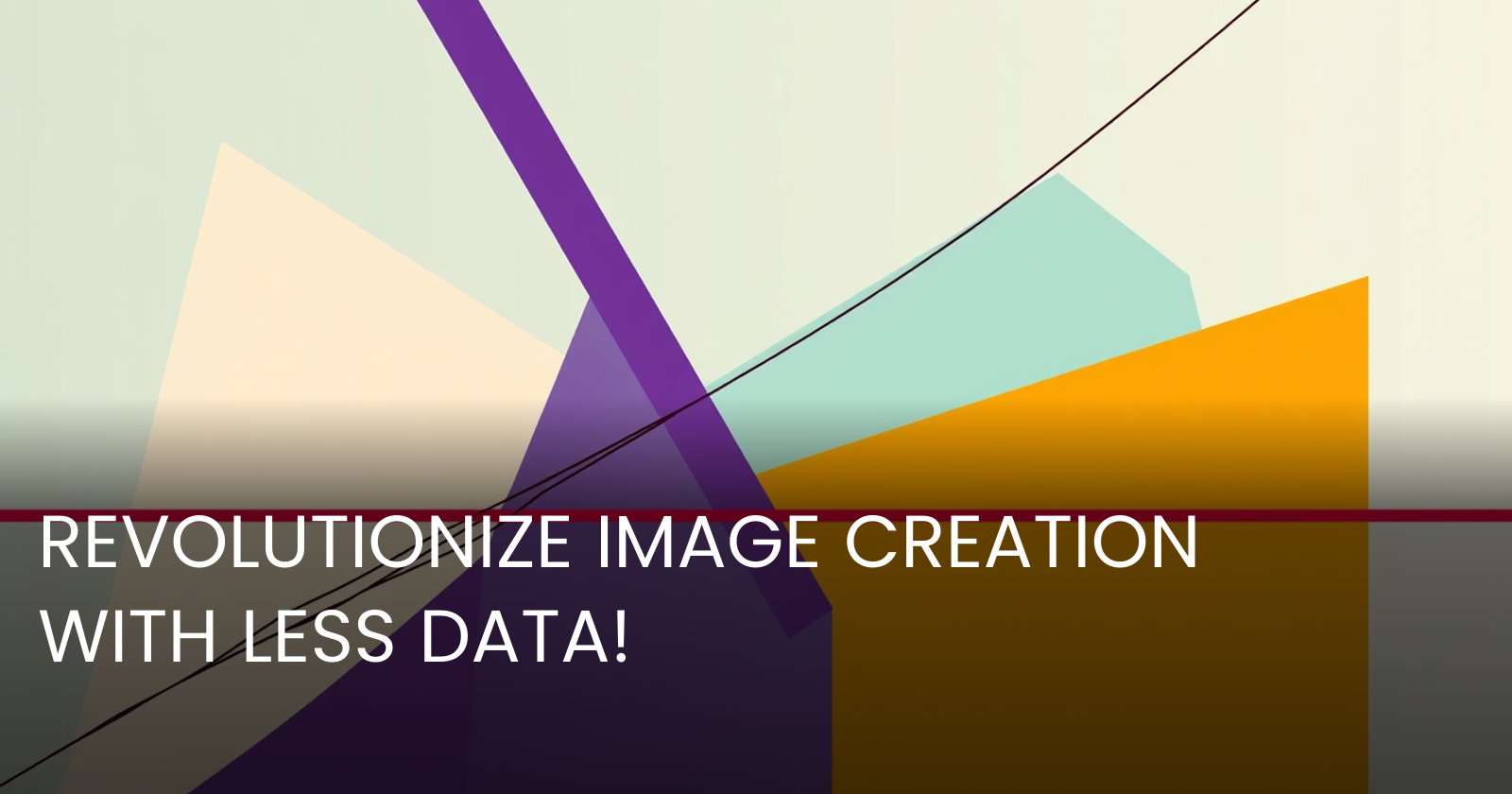
In this article, let's explore how the recent advancements embodied in DomainGallery, a few-shot domain-driven image generation method, can revolutionize business processes and product development. The paper we dissect delves into refining image generation techniques using minimal data while retaining quality and specificity. This is a game-changer, especially for industries creatively inclined or reliant on personalized digital content.
- Arxiv: https://arxiv.org/abs/2411.04571v1
- PDF: https://arxiv.org/pdf/2411.04571v1.pdf
- Authors: Liqing Zhang, Li Niu, Jianfu Zhang, Weiqiang Wang, Huijia Zhu, Jun Lan, Bo Zhang, Yan Hong, Yuxuan Duan
- Published: 2024-11-07
Main Claims
DomainGallery addresses the limitations of existing text-to-image (T2I) models that struggle when rendering images from niche, sparsely populated domains. The proposed solution fine-tunes pre-trained Stable Diffusion models by focusing on domain attributes—characteristics inherent to the dataset yet often ignored by broad-spectrum models.
Innovative Enhancements
This method introduces four attribute-centric enhancements:
- Prior Attribute Erasure: It cleans a domain’s prior attributes, preventing unwanted features from contaminating the generated image.
- Attribute Disentanglement: By separating domain and categorical attributes, it ensures individual elements are preserved in cross-category image production without leakage.
- Attribute Regularization: Aims to minimize overfitting through strategic loss implementation, maintaining model versatility despite limited data inputs.
- Attribute Enhancement: Increases the versatility, allowing subtle adjustments to existing attributes during the synthesis .
Business Applications and Opportunities
Revenue Generation and Optimization
- Creative Industries: Film and game developers can harness the power of DomainGallery to generate unique conceptual art with minimal data inputs, saving time and resources typically spent on manual creation.
- Marketing Innovations: Advertisers can customize campaigns based on intricate consumer attributes by producing personalized visuals that cater to specific tastes and preferences .
- E-commerce: Companies can build dynamic product catalogers where users visualize products in personalized settings, potentially boosting engagement and conversion rates.
New Products and Ideas
- Customized Visual Content Platforms: Platforms could allow users to create content using their unique style with ease, democratizing artistic expression.
- Virtual Reality (VR) and Augmented Reality (AR): Enhance immersion with auto-generated, themed environments based on user preferences, facilitating a more personalized experience .
Hyperparameters and Training Insights
The process involves setting up hyperparameters suitable for attribute-centric training phases. Prior attribute erasure applies LoRA configurations on precise UNet layers while preserving text encoder integrity. Parameters include:
- Training Steps: 500 for attribute erasure, enhanced with successive 1000 steps for finetuning.
- Learning Rates: Adopted rates are set to 1×10⁻⁴ and 5×10⁻⁵ for successive phases .
Hardware Requirements
DomainGallery experiments utilize a single NVIDIA RTX 4090 GPU with 24GB VRAM. The implementation uses memory-efficient techniques like gradient checkpointing and 8-bit Adam to streamline usage, making it accessible without extensive hardware resources .
Target Tasks and Datasets
The methodology optimizes generation across distinct domains, including:
- CUFS Sketches
- Van Gogh Houses
- Watercolor Dogs and FFHQ Sunglasses
- Wrecked Cars
These datasets broaden the application from style-centric generative tasks to content or theme-related demands .
Comparison with Other State-of-the-Art Models
Compared to techniques like DreamBooth and DomainStudio, DomainGallery consistently surpasses in both fidelity and diversity across domain-specific tasks. Its careful attribute handling allows it to outshine counterparts that falter due to overfitting or attribute misalignment .
Conclusions and Future Directions
DomainGallery demonstrates significant advancements in niche domain-driven image generation, addressing limitations observed in prior works with robust attribute management. Future explorations might address handling multi-category datasets simultaneously or refining attribute disentanglement for more complex domains.
By streamlining image generation processes with fewer resources yet achieving high-quality outputs, DomainGallery proves instrumental in reshaping industries reliant on personalized and dynamic visual content.
Subscribe to my newsletter
Read articles from Gabi Dobocan directly inside your inbox. Subscribe to the newsletter, and don't miss out.
Written by
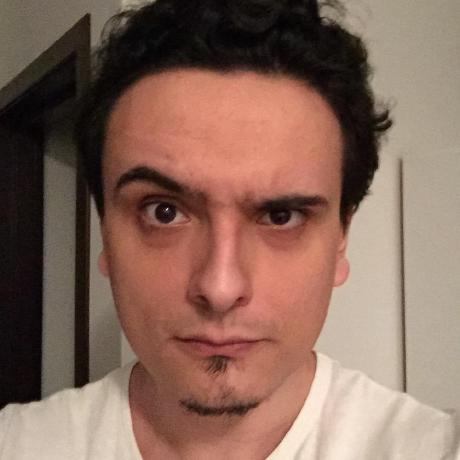
Gabi Dobocan
Gabi Dobocan
Coder, Founder, Builder. Angelpad & Techstars Alumnus. Forbes 30 Under 30.