Understanding the Impact of Cross-Lingual Transfer on Asian Historical Language Processing
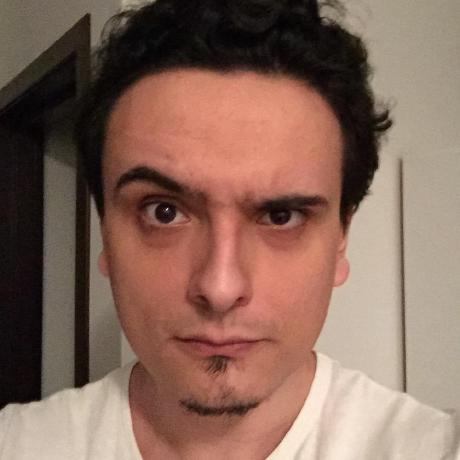

In a world of intertwined cultures and languages, understanding ancient scripts is vital for preserving history and enhancing linguistic studies. In this article, I break down the insights presented in a comprehensive study on processing historical East Asian languages using machine learning techniques, making it accessible to you.
- Arxiv: https://arxiv.org/abs/2411.04822v1
- PDF: https://arxiv.org/pdf/2411.04822v1.pdf
- Authors: Alice Oh, Kyunghyun Cho, Jiho Jin, Haneul Yoo, Seyoung Song
- Published: 2024-11-07
Main Claims of the Paper
The paper makes a bold claim: integrating Classical Chinese language data into language models for other East Asian scripts (such as Hanja used in Korea) doesn't significantly enhance performance across various tasks like machine translation (MT), named entity recognition (NER), and punctuation restoration (PR). The authors conducted a range of experiments across these tasks, discovering a marginal gain in machine translation and no significant improvements in NER or PR when Classical Chinese data was included.
New Proposals and Enhancements
The research introduces specialized corpora like the Korean Literary Collections (KLC) which encapsulate diverse Hanja writings. Exploring the intricacies of these texts suggests that selecting language data with similar writing styles may offer limited performance enhancements. Fine-tuning models using quantized low-rank adaptation (QLoRA) techniques for efficient parameter modification also highlights a modern approach for optimizing language models.
Leveraging the Paper for Business Opportunities
Despite the modest improvements reported, companies working with historical texts or multilingual content translation systems can leverage these insights in several ways:
Developing Multilingual Tools: Creating tools that offer better translations of under-represented languages. By understanding the limits of cross-lingual transfer, businesses can design more accurate software dedicated to specific language pairs.
Cultural Preservation Software: Companies can develop applications that help preserve and archive historical texts, providing efficient and user-friendly access to scholars and educators.
Text Analytics: Incorporating these findings into text analytics platforms can enhance understanding of customer sentiments and trends in multilingual data.
Custom Language Models: Building custom machine learning models for companies dealing with multilingual texts, focusing on fine-tuning on specific data to improve accuracy.
Hyperparameters and Model Training
The models in this study are trained using specific hyperparameters. The max sequence length is set to 512 with a batch size of 64 and an initial learning rate of 1e-4. A cosine scheduler with a warm-up ratio of 0.1 is used for optimizing this learning rate. These models are fine-tuned using state-of-the-art techniques that are still computationally feasible.
Hardware Requirements
Training such expansive models requires robust computational power. The experiments were conducted on servers with Intel Xeon processors and high-powered NVIDIA GPUs like the GeForce RTX 2080 Ti and Quadro RTX A6000. These specifications highlight the necessity of substantial hardware resources to efficiently handle model training and inference tasks.
Target Tasks and Datasets
The paper focuses on three primary tasks: machine translation (MT), named entity recognition (NER), and punctuation restoration (PR). Datasets specific to these tasks were developed to ensure comprehensive analysis, such as the AJD, KLC, GLNER, and WYWEB datasets, each contributing a unique dimension to the study.
Comparing with State-of-the-Art Alternatives
Compared to other advanced methods, the study shows that the use of Classical Chinese data offers no significant edge over existing models purely trained on the target language data. Though promising techniques like QLoRA were employed, it appears that the fundamental differences in languages within the same cultural sphere have a larger impact than anticipated.
Conclusions and Areas for Improvement
In conclusion, the paper reveals limited cross-lingual transfer effectiveness for historical languages within the Sinosphere. Improvements call for more precise data selection and validation experiments. The research suggests that empirical data validation is crucial over assumptions based on linguistic similarities.
As a takeaway for companies, this study encourages investment in exploring and validating language models tailored to specific language nuances, underscoring the importance of a customized approach for each unique language domain. As the field progresses, leveraging such insights can anchor cost-effective and culturally sensitive language technology solutions.
Subscribe to my newsletter
Read articles from Gabi Dobocan directly inside your inbox. Subscribe to the newsletter, and don't miss out.
Written by
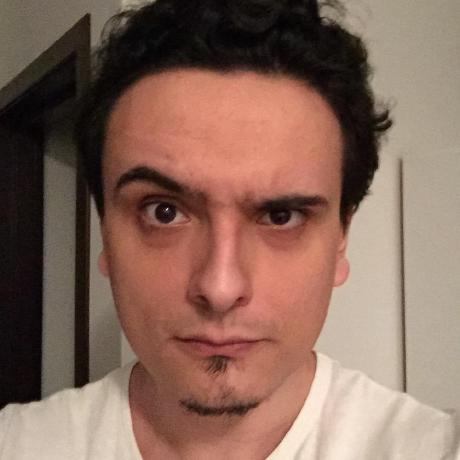
Gabi Dobocan
Gabi Dobocan
Coder, Founder, Builder. Angelpad & Techstars Alumnus. Forbes 30 Under 30.