Peri-midFormer: A Revolutionary Approach to Time Series Analysis
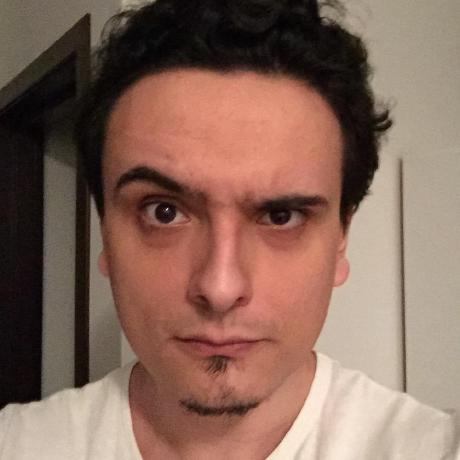
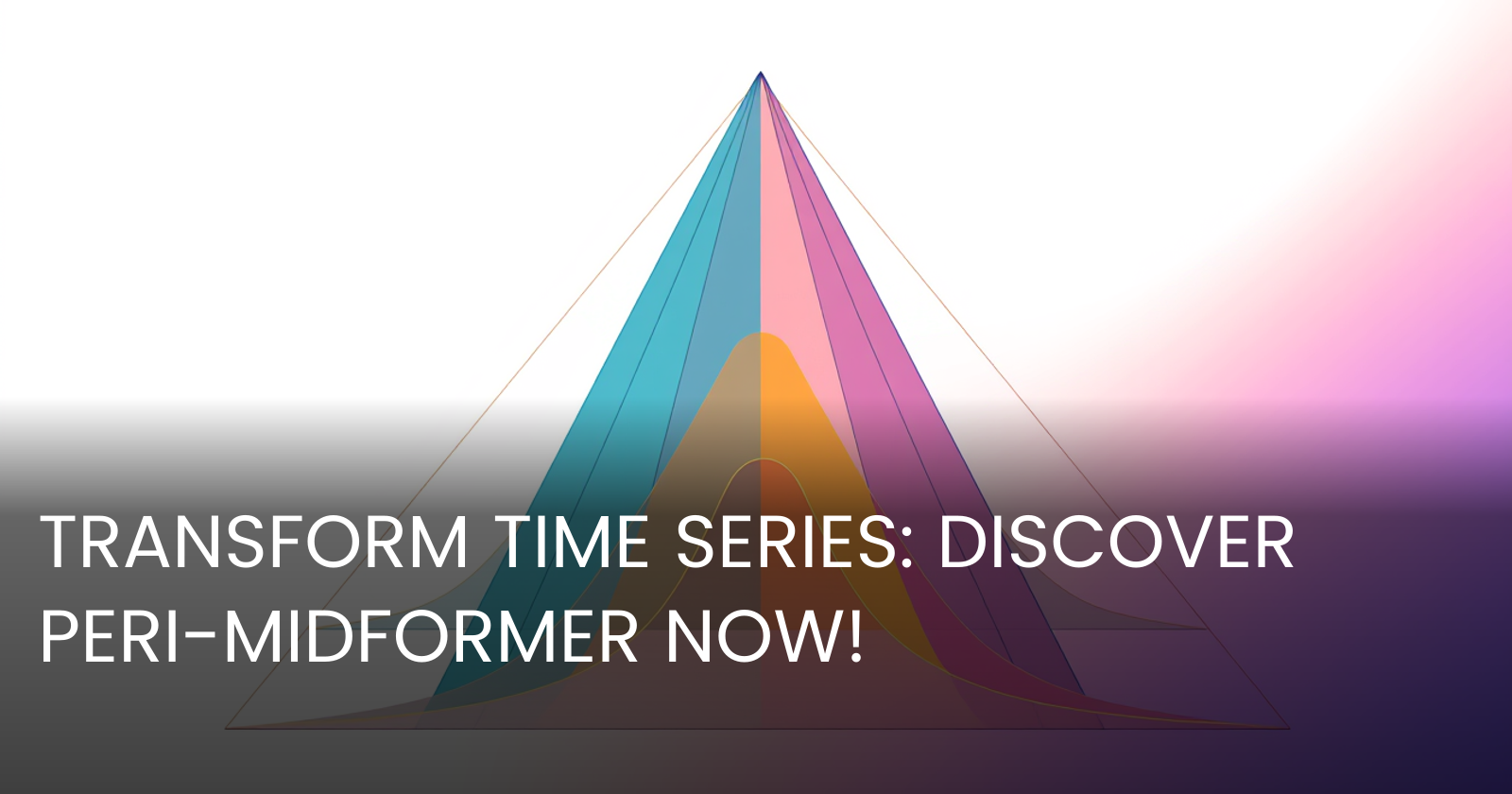
Time series analysis plays a pivotal role in many industries, ranging from finance to weather forecasting, anomaly detection, and more. A recently published scientific paper delves into an innovative method called Peri-midFormer which aims to revolutionize how time series data is analyzed by harnessing its periodic components. This article breaks down the paper's complex ideas into simple concepts for you, explaining its potential applications for businesses and industries.
- Arxiv: https://arxiv.org/abs/2411.04554v1
- PDF: https://arxiv.org/pdf/2411.04554v1.pdf
- Authors: Shuyuan Yang, Zhixi Feng, Gechang Yao, Qiang Wu
- Published: 2024-11-07
What Are the Main Claims of the Paper?
The main claim of the paper is that previous methods of time series analysis, which largely treat time series as linear sequences, are limited in their ability to capture the complex, multidimensional relationships inherent in actual data. To address this, the authors propose a novel framework called the Periodic Pyramid. This structure transforms one-dimensional time series data into a multi-level pyramid, capturing the varied periodic relationships within the dataset.
What are the New Proposals or Enhancements?
Introduction of Peri-midFormer
The Peri-midFormer model incorporates a new mechanism called the Periodic Pyramid Attention Mechanism. This allows the model to automatically capture dependencies across different and similar periods within the data, addressing both temporal variations and dependencies.
Rich Temporal Feature Extraction
In addition to the Pyramid structure, the paper discusses Periodic Feature Flows, which help decode complex temporal variations. These features provide robust periodic information for various analytical tasks, ensuring the model's adaptability to different applications [4:0†source].
How Can Companies Leverage the Paper?
Unlocking New Business Opportunities
With its ability to efficiently analyze complex time series data, companies in sectors like finance, healthcare, and utilities can leverage Peri-midFormer to improve forecasts and predictions. This could help in budgeting, resource allocation, and even customer demand prediction.
Enhancing Existing Processes
Businesses can use Peri-midFormer to enhance anomaly detection in industrial IoT data, enabling preemptive maintenance and reducing downtime. For weather-dependent sectors like agriculture, the model could provide more accurate long-term forecasts, optimizing planning and operations.
What are the Hyperparameters? How is the Model Trained?
The Peri-midFormer model employs various hyperparameters, such as k
, which influences how many levels of periodicity are considered within the time series pyramid. It also uses a flexible number of layers and model dimensions (from 16 to 768) depending on the task.
The training involves capturing temporal dependencies using a multi-level decomposition of the data, where periodic layers are involved. Training optimization is conducted using the ADAM optimizer with different learning rates tailored for specific tasks.
What are the Hardware Requirements to Run and Train?
Training Peri-midFormer is relatively computationally efficient compared to some state-of-the-art models. In comparison to models like Time-LLM that require extensive resources due, Peri-midFormer manages to deliver near-optimal performance without such demands.
What are the Target Tasks and Datasets?
The paper demonstrates the model's potential using tasks like forecasting, classification, imputation, anomaly detection, and others. It uses widely recognized datasets such as ETTh2 and UEA Heartbeat to showcase its performance.
How Do the Proposed Updates Compare to Other SOTA Alternatives?
Comparison with Previous Models
Peri-midFormer delivers state-of-the-art performance across multiple benchmarks, outperforming contemporaries such as GPT4TS and PatchTST. While some models like Time-LLM have a slight edge in certain tasks, their computational requirements make Peri-midFormer a more practical choice.
What are the Conclusions and Potential Improvements?
The authors conclude that while Peri-midFormer shows exceptional performance in many scenarios, it could struggle with datasets lacking clear periodic characteristics. Future research will focus on broadening its applicability by refining its ability to manage non-periodic datasets.
In summary, the Peri-midFormer model offers a game-changing approach to time series analysis by efficiently modeling complex periodic components. Its adaptability, efficiency, and performance in comparison to existing methodologies mark it as a promising tool for a multitude of industries aiming to leverage advanced data analytics for business advancement.
Subscribe to my newsletter
Read articles from Gabi Dobocan directly inside your inbox. Subscribe to the newsletter, and don't miss out.
Written by
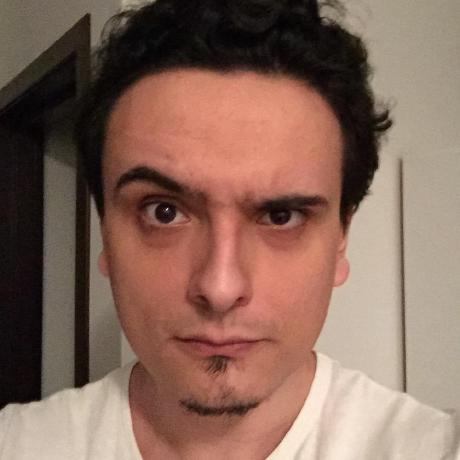
Gabi Dobocan
Gabi Dobocan
Coder, Founder, Builder. Angelpad & Techstars Alumnus. Forbes 30 Under 30.