Breaking Down the M3D Framework: A Leap in Single-View 3D Reconstruction
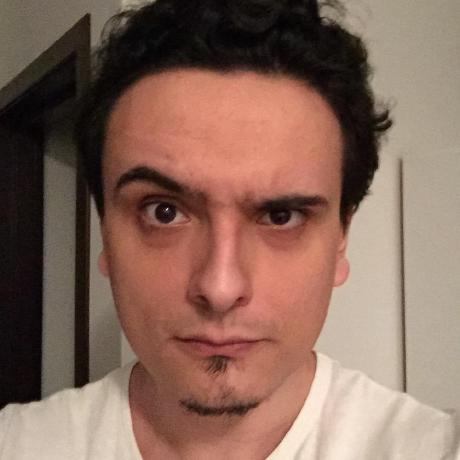

If you've ever marveled at a CAD model spun out of a single photo or felt the thrill of a VR experience crafted meticulously from simple inputs, you're witnessing the pinnacle of cutting-edge technological advancements. Single-view 3D reconstruction—turning a flat image into a 3D model—is a tricky challenge that is being addressed increasingly well by smart people doing smart stuff. The latest attempt to push the boundaries further comes in the form of a scientific exploration titled "M3D: Dual-Stream Selective State Spaces and Depth-Driven Framework for High-Fidelity Single-View 3D Reconstruction." This article offers a dig into this complex topic, breaking down what these advances mean for businesses and tech enthusiasts alike.
- Arxiv: https://arxiv.org/abs/2411.12635v1
- PDF: https://arxiv.org/pdf/2411.12635v1.pdf
- Authors: Itaru Kitahara, Chun Xie, Yu Zhou, Pragyan Shrestha, Luoxi Zhang
- Published: 2024-11-19
Unpacking M3D's Core Claims
The core of the paper revolves around improving how machines infer the detailed 3D structure of objects from just one RGB image. Recognized as a daunting task due to the absence of depth information and inherent ambiguities, single-view 3D reconstruction is ripe for new approaches. Existing models face trade-offs in capturing intricate details and broader contextual information; essentially, they're great at spotting the bark but not so hot at comprehending the forest. M3D proposes a method that skillfully balances the extraction of global and local features. The authors suggest that by integrating selective geometric features and adopting a depth attribute alongside RGB inputs, their method outshines current standards in reconstructive precision and quality.
The Magic Sauce: New Proposals and Enhancements
The proprietary enhancements proposed in this paper include a dual-stream architecture within the M3D framework that fosters better feature extraction. To tackle the shortcomings of existing convolutional and transformer-based models, which either lag in detail or context, the M3D framework incorporates:
Dual-Stream Feature Extraction Strategy: This clever technique divides the process into two separate paths—one for RGB features and another solely for depth information. By doing this, the framework captures both local details and wider contextual features for a more holistic object reconstruction.
Selective State Space Model (SSM): This model adeptly combines CNN layers for nuanced, shallow features and transformer elements for broader contextual insights. It brings optimized global and local information retrieval capabilities to the table.
Depth Estimation Module: Tackling depth via a specifically trained module that supplements RGB data gives precision to the geometrical comprehension that mere color and texture can't grasp alone. This addition notably enhances the object's depth and surface detail, leading to remarkably accurate 3D reconstructions.
Transforming Business and Industry Applications
For businesses, the ability to translate 2D images into detailed 3D models opens a treasure trove of possibilities. Here's how companies stand to gain:
Virtual Reality (VR) and Augmented Reality (AR): From real estate to video games, the capacity to create immersive environments from a single image can reduce costs and time, accelerating content creation without sacrificing detail.
E-commerce: Retailers can allow consumers to visualize products in their environment, leading to higher engagement and conversion rates. Technology that streamlines product uploads into 3D with limited resources is invaluable.
Industrial Design and Manufacturing: Engineers and designers can rapidly prototype and iterate designs. By creating models from simple sketches or photographs, the process from concept to production can be vastly accelerated.
Autonomous Driving: By accurately mapping 3D environments from single snapshots, vehicular systems can interpret complex scenes, improving navigation and safety measures.
The Training Process: Datasets and Methodologies
Model training in this research was predominantly based on the FRONT3D dataset. This dataset contains richly annotated 3D scenes suitable for the model's high expectations of scene understanding. The training processes employed computationally intense convolutional layers (ResNet), advanced transformer mechanisms, and a custom depth estimation model to ensure the reconstruction tasks were comprehensive and accurate. Through rigorous epochs performed on a robust NVIDIA H100 GPU infrastructure, the model was honed over extensively staged training sessions that prioritized reducing Chamfer Distance among other metrics to achieve significant accuracy benchmarks.
Hardware Requirements
For businesses looking to implement something akin to the M3D framework, having access to significant computational power is crucial. The training and operation of such a model involve processing high volumes of data with complex calculations; therefore, an industrial-level GPU, like NVIDIA's H100, is required. This processing power ensures that both RGB and supplementary depth data are handled without lag, yielding real-time applicable outputs in high-fidelity scenarios.
Standing Up to Other SOTA (State-of-the-Art) Alternatives
When evaluated against contemporary methods like Zero-1-to-3 and Shape-E, both of which use sizeable pretrained models and similarity-driven diffusion for depth assessment, the M3D framework surpasses in terms of reconstruction fidelity across key measurements. While other techniques may falter, especially in geometrically complex scenes with occlusions, M3D's use of dual data streams and SSM leads to robust and intricate final 3D forms.
Wrapping Up: Conclusions and Future Directions
In summary, the M3D framework stands out in its ability to achieve high-fidelity 3D reconstruction by efficiently integrating depth perception into its processing using a dual-stream model. The direct application of these technologies can revolutionize industries by automating and enriching processes that previously consumed much time and resources.
However, there's always room for enhancement. Future research directions proposed include reducing data dependencies by venturing into semi-supervised learning, potentially refining how models learn with minimal labeled datasets. This can democratize high-quality 3D reconstruction capabilities, making them accessible to more varied applications.
The authors of the paper have not only furthered the technological frontier of 3D reconstruction but have built a bridge that businesses can now walk over to innovate and improve offerings, embrace new opportunities, and lead in an increasingly digital landscape.
Subscribe to my newsletter
Read articles from Gabi Dobocan directly inside your inbox. Subscribe to the newsletter, and don't miss out.
Written by
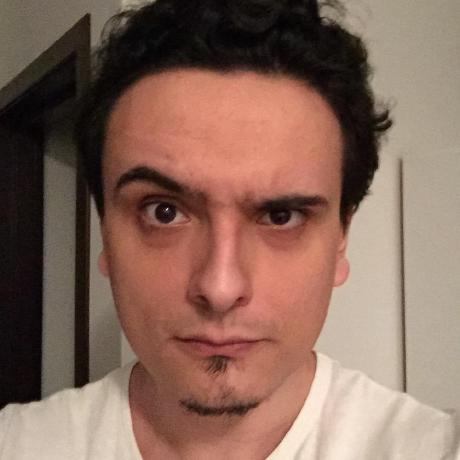
Gabi Dobocan
Gabi Dobocan
Coder, Founder, Builder. Angelpad & Techstars Alumnus. Forbes 30 Under 30.