Unlocking the Power of Invariant Shape Representation in Image Classification
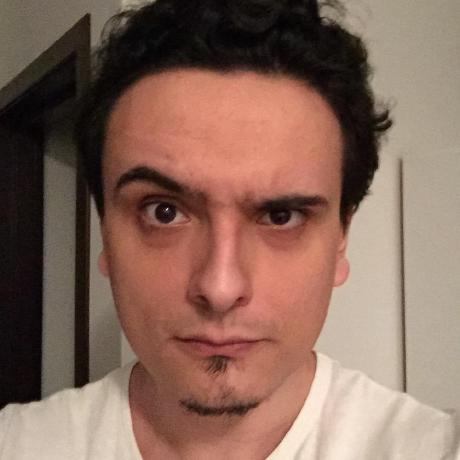

In the realm of machine learning and artificial intelligence, the exploration and development of new methodologies in image classification have been pivotal for advancing technology. One such groundbreaking development is the introduction of Invariant Shape Representation Learning (ISRL) as proposed by researchers Tonmoy Hossain, Jing Ma, Jundong Li, and Miaomiao Zhang. This article breaks down their scientific paper "Invariant Shape Representation Learning For Image Classification," illuminating its main claims, methodologies, and applicability, particularly for businesses and organizations seeking to leverage AI to boost revenue or refine processes.
- Arxiv: https://arxiv.org/abs/2411.12201v1
- PDF: https://arxiv.org/pdf/2411.12201v1.pdf
- Authors: Miaomiao Zhang, Jundong Li, Jing Ma, Tonmoy Hossain
- Published: 2024-11-19
Understanding the Main Claims of the Paper
The crux of Hossain and colleagues' research lies in the enhancement of image classification accuracy by focusing on invariant shape features, rather than relying on potentially misleading statistical correlations. Traditional deep neural networks (DNNs) often exploit these statistical correlations between shape features and target labels, but these correlations can be spurious and unstable across varying environments, resulting in biased or inaccurate predictions.
The authors introduce the concept of ISRL to target this issue, proposing a framework that learns invariant representations of shapes and images. By utilizing invariant risk minimization (IRM) techniques, the ISRL framework aims to identify features that have stable relationships with target labels, regardless of environmental changes. This robust approach ensures more accurate and reliable predictions across different scenarios, improving the performance of image classifiers.
New Proposals and Enhancements
The paper presents several novel enhancements:
Joint Learning Paradigm: ISRL combines image and shape representations, learning invariant features across multiple environmental distributions. This creates a more integrated and stable framework for image classification.
Efficiency and Adaptability: By effectively eliminating spurious correlations, ISRL increases the adaptability of image classifiers when faced with unseen data or environments.
Causal Representation Learning: The method opens avenues for learning representations that capture the true causal features associated with target labels, going beyond mere statistical association.
These advancements position ISRL as a significant step forward in the domain of robust image classification, particularly in complex environments where conventional models may falter due to confounding factors.
Leveraging ISRL in Business: New Products and Ideas
The applicability of ISRL goes far beyond academia. Businesses can harness this technology to revolutionize several key areas:
Healthcare Imaging: Medical imaging can be particularly challenging due to the variability in patient data. By using ISRL, healthcare providers could vastly improve the accuracy of disease detection systems, such as those for neurodegenerative diseases or cardiac conditions, leading to earlier and more reliable diagnoses.
Retail and Fashion: Image classification is a crucial component in automated inventory management and personalized shopping experiences. ISRL can enhance these systems by accurately identifying products and understanding consumer preferences across diverse shopping environments.
Autonomous Vehicles: Precision in identifying objects and interpreting surroundings is vital for the safety and efficiency of autonomous vehicles. ISRL can provide high-fidelity classification despite changing weather conditions or terrains.
Security and Surveillance: In security-focused applications, the ability to correctly identify and classify shapes and objects in various lighting and environmental contexts is crucial. ISRL-enhanced systems could reduce false positives/negatives in threat detection.
Potential for New Business Models
AI-as-a-Service (AIaaS): Companies can offer ISRL-based solutions as a service, providing robust classification tools to other businesses needing adaptable and reliable image classification methods.
Custom AI Solutions: Businesses could develop tailored applications using ISRL for specific industries, such as agriculture (for crop monitoring) or logistics (for package sorting).
Training the Model and Dataset Utilization
ISRL is trained using a variety of datasets, including simulated 2D images, 3D brain MRIs, and cardiac MRI videos. The methodology involves learning from deformable shape representations, which capture detailed geometric and structural information crucial for classification tasks.
The training process is divided into two parts: unsupervised learning for geometric deformations and an invariant classification network. Together these modules help in minimizing empirical risk across different environments, ensuring that the extracted features are truly invariant.
Hardware Requirements
Running and training ISRL can be resource-intensive, typically requiring GPUs for efficient computation due to the complexity of operations involved in deformation-based shape representation and the processing of large, high-dimensional medical datasets.
Comparisons with State-of-the-Art Alternatives
When compared to other state-of-the-art models, ISRL demonstrates superior performance, especially in scenarios involving environmental shifts or data distribution changes. Benchmarked against models like ERM (Empirical Risk Minimization) and other IRM-based models, ISRL consistently shows higher accuracy and robustness.
Conclusions and Areas for Improvement
The outcomes of the research underline ISRL's capability to substantially enhance classification accuracy and reliability. However, there are still areas for potential improvement:
Scalability: While ISRL is effective, scaling the model for extremely large datasets or real-time applications might pose challenges that require further optimization strategies.
Applicability Across Modalities: Exploring ISRL's ability to generalize across various modalities (e.g., combining video data with static images) could expand its utility.
Integration with Other AI Techniques: Combining ISRL with other machine learning paradigms, such as reinforcement learning or generative adversarial networks, could unlock deeper insights and capabilities.
In summary, the paper presents a significant stride towards more reliable and accurate image classification systems by focusing on invariant shape features. For businesses, ISRL offers a pathway to develop cutting-edge applications that can greatly enhance operational efficiency and create new revenue streams. As the technology matures, further exploration and integration with other AI methodologies could amplify its impact across sectors.
Subscribe to my newsletter
Read articles from Gabi Dobocan directly inside your inbox. Subscribe to the newsletter, and don't miss out.
Written by
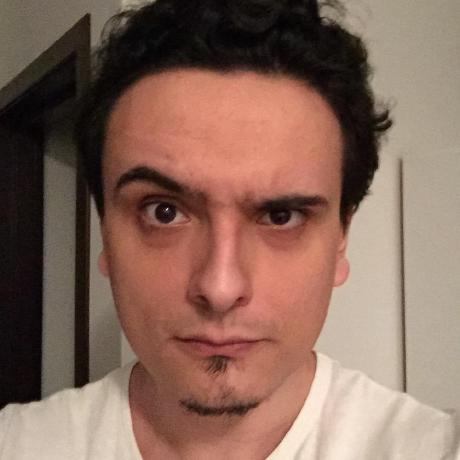
Gabi Dobocan
Gabi Dobocan
Coder, Founder, Builder. Angelpad & Techstars Alumnus. Forbes 30 Under 30.