Unlocking Medical Image Segmentation: A Deep Dive into SAM-Driven Pseudo Label Refinement
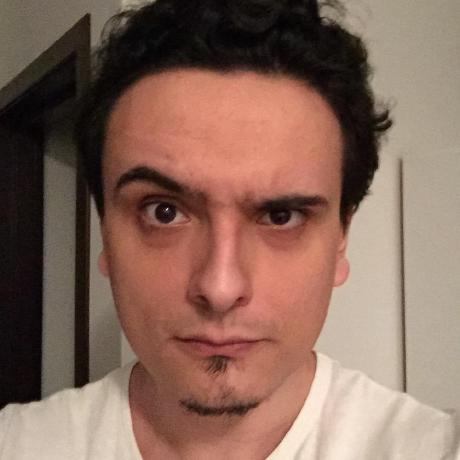

Modern technology has transformed many aspects of healthcare, with medical imaging being one zone experiencing remarkable advancements. Yet, accurate segmentation of medical images remains a challenging task. Often, it hinges on vast datasets full of precision-annotated images—pretty tricky and expensive, given they require expert knowledge to label. A recent paper titled "Sam Carries The Burden: A Semi-Supervised Approach Refining Pseudo Labels For Medical Segmentation" offers a fresh perspective on this challenge. What follows is an exploration of this innovative approach, what it proposes, and how it could reshape healthcare industries.
- Arxiv: https://arxiv.org/abs/2411.12602v1
- PDF: https://arxiv.org/pdf/2411.12602v1.pdf
- Authors: Mattias Heinrich, Ludger Tüshaus, Anne-Nele Schröder, Ronja Jäger, Maren Balks, Lasse Hansen, Ron Keuth
- Published: 2024-11-19
What the Paper Claims: Breaking It Down
The heart of the paper revolves around enhancing medical image segmentation by leveraging the Segment Anything Model (SAM). This novel method aims to mitigate the heavy reliance on large, annotated datasets by utilizing semi-supervised learning techniques. Here's a breakdown of the paper’s key claims:
Reduction in Demand for Annotated Data: By utilizing SAM's ability to understand abstract objects, the approach allows for the creation of pseudo labels. These are essentially improved segmentation guesses that can be used like real labels during training.
Quality Improvement: The method refines initial segmentations from a small set of annotated data using SAM. This refinement results in improved segmentation masks for unlabelled data. Remarkable improvements were recorded, bumping up effectiveness metrics (like the Dice score) significantly.
Comparison with Other Methods: The proposed method outperforms state-of-the-art supervised learning methods such as nnU-Net and semi-supervised models like the Mean Teacher. It shows that fewer precise initial data can still yield high segmentation performance.
Applicability in Different Medical Domains: This technique works across various domains, exemplified by its application in segmenting the bones of pediatric wrists and dental radiographs (teeth in X-ray images).
New Proposals and Enhancements: The Core Idea
The paper leverages the Segment Anything Model (SAM) to significantly enhance pseudo labeling in a semi-supervised setting. Here’s how:
Prompt-Based Segmentation: SAM can generate segmentation maps using prompts like bounding boxes and seed points. The proposed method refines initial segmentation derived from limited annotated cases by using these prompts. Essentially, it uses what it knows to improve guesses for what it doesn't know.
Pseudo Labeling Routine: The workflow involves training an initial model on limited data, generating initial pseudo labels, and then using SAM to refine these predictions to create more accurate pseudo labels for unlabelled data.
Automatic Prompt Extraction: This includes mechanisms for cleaning and processing predicted segmentation masks to generate effective prompts for SAM, making the process more automatic and less reliant on manual input.
Leveraging the Paper in Real-World Scenarios: Application and Business Ideas
Translating from concept to real-world application, the implications of this work are vast. Companies in the healthcare and medical imaging space can benefit significantly:
Streamlined Annotation Process: With reduced reliance on accurate annotations, companies can expedite the dataset generation stage for segmentation tasks, cutting costs and resource requirements.
Enhanced AI-Driven Diagnostics: By refining pseudo labels with improved accuracy, diagnostic solutions can become even more precise, pivotal for healthcare services that rely on accurate segmentation for patient assessments.
Development of New Tools and Services: Enterprises can innovate with new products for hospitals and medical research institutions. These include tools that offer quick semi-automatic segmentation, helping medical professionals save time when processing imaging data.
Contributing to Remote and Global Healthcare: Entities can apply these advancements in developing healthcare markets, enabling access to advanced diagnostic capabilities without extensive local expertise.
Understanding the Training and Data Sets: The Technical Cockpit
The process to achieve the outcomes described involves meticulous training with a mix of labeled and unlabelled datasets:
Datasets Used: The study used two datasets - GRaZPEDWRI-DX for pediatric wrist segmentation and a dental radiograph dataset for teeth segmentation. These datasets included both labelled and vast amounts of unlabelled images, suitable for semi-supervised learning.
Training Methodology: Training starts on a small labelled dataset. The model learns basic segmentation patterns, generating preliminary predictions. SAM then takes over to refine these predictions to train a more comprehensive model. This results in using both initial and refined labels as pseudo labels for unlabelled training data.
Tech Stack and Hardware: The training employs U-Net models optimized with Adam's optimizer. Computationally, it’s relatively lightweight, requiring around 11GB of VRAM and performed on a standard NVIDIA RTX 2080 Ti.
How Does It Compare? A Look at State-of-the-Art Alternatives
In the fast-paced realm of AI-driven medical imaging, standing out requires competitive performance:
Comparison with SOTA Models: The SAM-enabled approach outperformed the intensive nnU-Net and the semi-supervised Mean Teacher method, especially when reducing the annotation burden.
Dice Score Improvements: For specific tasks (e.g., dental and pediatric wrist), the method showed 6-9% improvement in Dice scores, benchmarking it higher than others in the space.
Versatility Across Domains: Unlike some models that require significant tailoring to new domains, SAM’s generalization capability makes it more versatile for different medical settings.
Conclusions and Areas for Future Exploration
To round up, the pseudo-labeling approach using SAM holds potential for redefining how medical imaging tasks are tackled. Here are the paper’s conclusions and some spaces for future tweaks:
Semi-Supervised Success: The work demonstrates that harnessing abstract object understanding capabilities via SAM can significantly bridge the gap between fully supervised and unsupervised setups.
Incorporating More Objects Per Class: Currently, the method is limited to one instance per class per image. Exploring ways to scale beyond that could unlock further benefits.
General Method vs. Specific Tools: While this study shows SAM’s prowess without extensive domain adaptation, further refinement or creation of domain-specific adaptations could enhance specific segmentation tasks.
In summary, these insights and advancements present not just a technological step forward, but open practical avenues for businesses globally. They signal a promising landscape where AI can advance healthcare solutions, drive efficiency, and ultimately provide improved patient outcomes.
Subscribe to my newsletter
Read articles from Gabi Dobocan directly inside your inbox. Subscribe to the newsletter, and don't miss out.
Written by
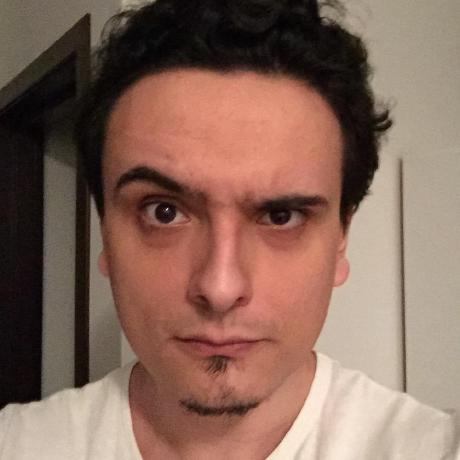
Gabi Dobocan
Gabi Dobocan
Coder, Founder, Builder. Angelpad & Techstars Alumnus. Forbes 30 Under 30.