Understanding the Contourlet Refinement Gate Framework for Infrared Image Super-Resolution
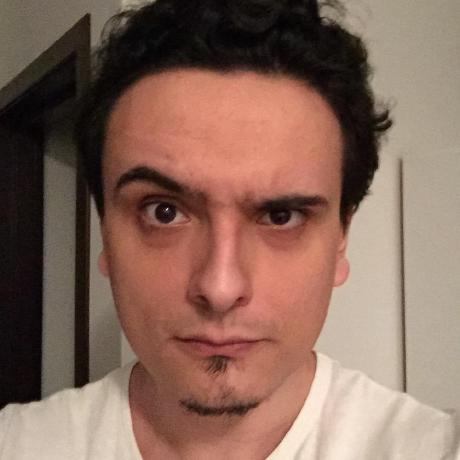

In the world of digital imaging, the challenge of transforming low-resolution images into their high-resolution counterparts, known as image super-resolution (SR), is a well-studied yet continually evolving problem. This becomes even more complex when dealing with infrared images, which are different from standard RGB images in both their function and degradation patterns. Today, we're digging deep into a new advanced methodology proposed by Yang Zou and colleagues: the Contourlet Refinement Gate Framework for Thermal Spectrum Distribution Regularized Infrared Image Super-Resolution.
- Arxiv: https://arxiv.org/abs/2411.12530v1
- PDF: https://arxiv.org/pdf/2411.12530v1.pdf
- Authors: Yanning Zhang, Peng Wang, JinYuan Liu, Long Ma, Xingyuan Li, Zhipeng Zhang, Zhixin Chen, Yang Zou
- Published: 2024-11-19
What Are the Main Claims?
The authors underscore significant claims about how traditional methods fall short when directly applied to infrared images. These techniques, often designed for visible light images, try to boost image clarity by leveraging features from RGB images. They miss out on the distinct characteristics inherent to infrared imaging. The standard approaches inadvertently alter the infrared spectral distribution, which is crucial for applications where machine perception, such as object detection and segmentation, is vital. The paper suggests a novel framework focused on preserving the spectral distribution fidelity of infrared-specific features, a game-changer for the task of super-resolution in this domain.
New Proposals and Enhancements
One innovative leap from this research is the adoption of what they call a Contourlet Refinement Gate Framework. This approach leverages the multi-scale and multi-directional decomposition capacities of the Contourlet Transform to address the unique high-frequency needs of infrared images. Also, a Spectral Fidelity Loss is introduced to ensure the preservation of essential infrared-specific features throughout the image enhancement process.
The framework doesn't stop there. It also incorporates a two-stage prompt learning strategy. This strategy allows the model to better understand the degradation pattern of infrared images, guiding it to more precisely learn the mapping from low-resolution to high-resolution infrareds. These combinations position this framework at the forefront of methodologies designed specifically with infrared images in mind.
Leveraging the Framework for Business
The potential applications for this advanced method are as varied as they are impactful. For companies in industries reliant on infrared imaging—such as security surveillance, medical diagnostics, and remote sensing—the advantages are twofold: enhanced image clarity and preservation of critical data. Enhanced infrared images mean more reliable security systems, improved diagnostic imaging in the medical field, and better data quality in geospatial and environmental monitoring tasks.
In commercial sectors, businesses can now offer products or services with superior quality of detail in infrared imagery, opening avenues for new revenue streams or enhancing existing ones. They could, for instance, develop more advanced camera technologies or improve image processing software used in various fields.
How Is the Model Trained?
The model employs a detailed architecture composed of modules like the Contourlet Transform, which separates images into low and high-frequency components, effectively extracting important features across scales and directions. Moreover, Spatial and Channel Attention Blocks work to refine these extracted features by enhancing spatial relationships and channel-specific details.
Training utilizes datasets like the M3FD, TNO, and RoadScene, which are specifically chosen to cater to infrared image properties. Each image is carefully processed, and the model learns through an intricate dance of losses, including the Spectral Fidelity and Degradation Losses, ensuring the high fidelity of super-resolved images.
Hardware Requirements
Running and training this highly specialized model would typically require robust hardware capabilities. Researchers trained their network on a GPU, specifically the GeForce RTX 4090. This high-end processing capability, in tandem with techniques like data augmentation and careful initialization of learning rates, allows the model to handle the demanding computational tasks inherent in super-resolution processes efficiently.
Comparison with Other State-of-the-Art Methods
Compared to alternatives in the field, the use of the Contourlet Transform offers a tangible edge over traditional wavelet transforms. The meticulous process of preserving both high and low-frequency components through this transform leads to better overall image fidelity and detail restoration.
The proposed framework demonstrates superior performance in a suite of visual and perceptual assessments, not only rivaling current state-of-the-art approaches such as GANs and Transformer models but surpassing them, particularly in the context of infrared applications.
Conclusions and Areas for Improvement
Through extensive testing and comparison, the authors reveal that their methodology significantly outperforms existing SR models, especially when infrared characteristics are paramount. However, while their approach opens new avenues, it isn't without potential areas for improvement. The system's complexity might prove a hurdle for some applications, and the need for powerful hardware may limit accessibility for broader uses.
In essence, what Yang Zou and his team have offered is not just a step forward in the realm of image super-resolution but a leap, particularly for infrared imaging. By focusing on the fidelity and preserving essential spectral details, they provide a blueprint for future advancements.
Final Thoughts
The Contourlet Refinement Gate Framework elevates the capabilities of super-resolution techniques in infrared imaging, showcasing how tailoring methodologies to specific imaging types can revolutionize applications. This is a breakthrough not only academically but also in practical, commercial senses across industries reliant on the nuanced performance of infrared imaging. As companies look to integrate such advancements, they're not just embracing cutting-edge technology but enhancing their ability to transform raw data into actionable insights, with all the precision and clarity that the future demands.
Subscribe to my newsletter
Read articles from Gabi Dobocan directly inside your inbox. Subscribe to the newsletter, and don't miss out.
Written by
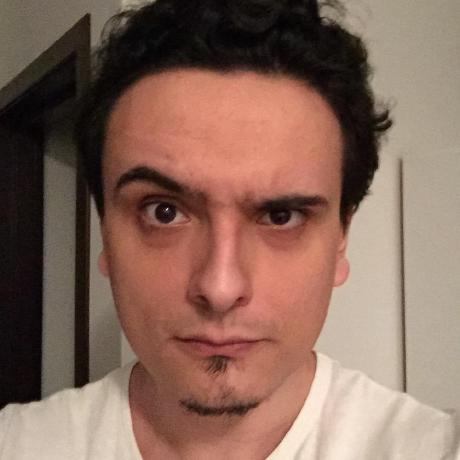
Gabi Dobocan
Gabi Dobocan
Coder, Founder, Builder. Angelpad & Techstars Alumnus. Forbes 30 Under 30.