Efficient Transfer Learning For Video-Language Foundation Models Explained
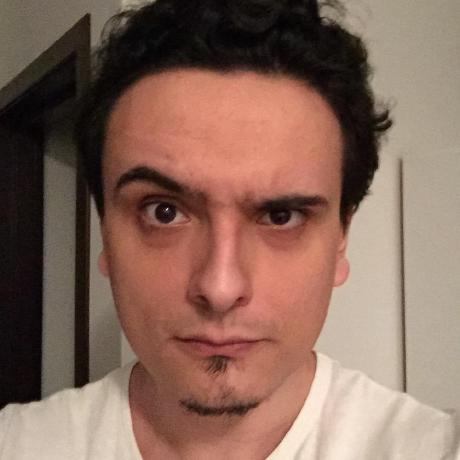
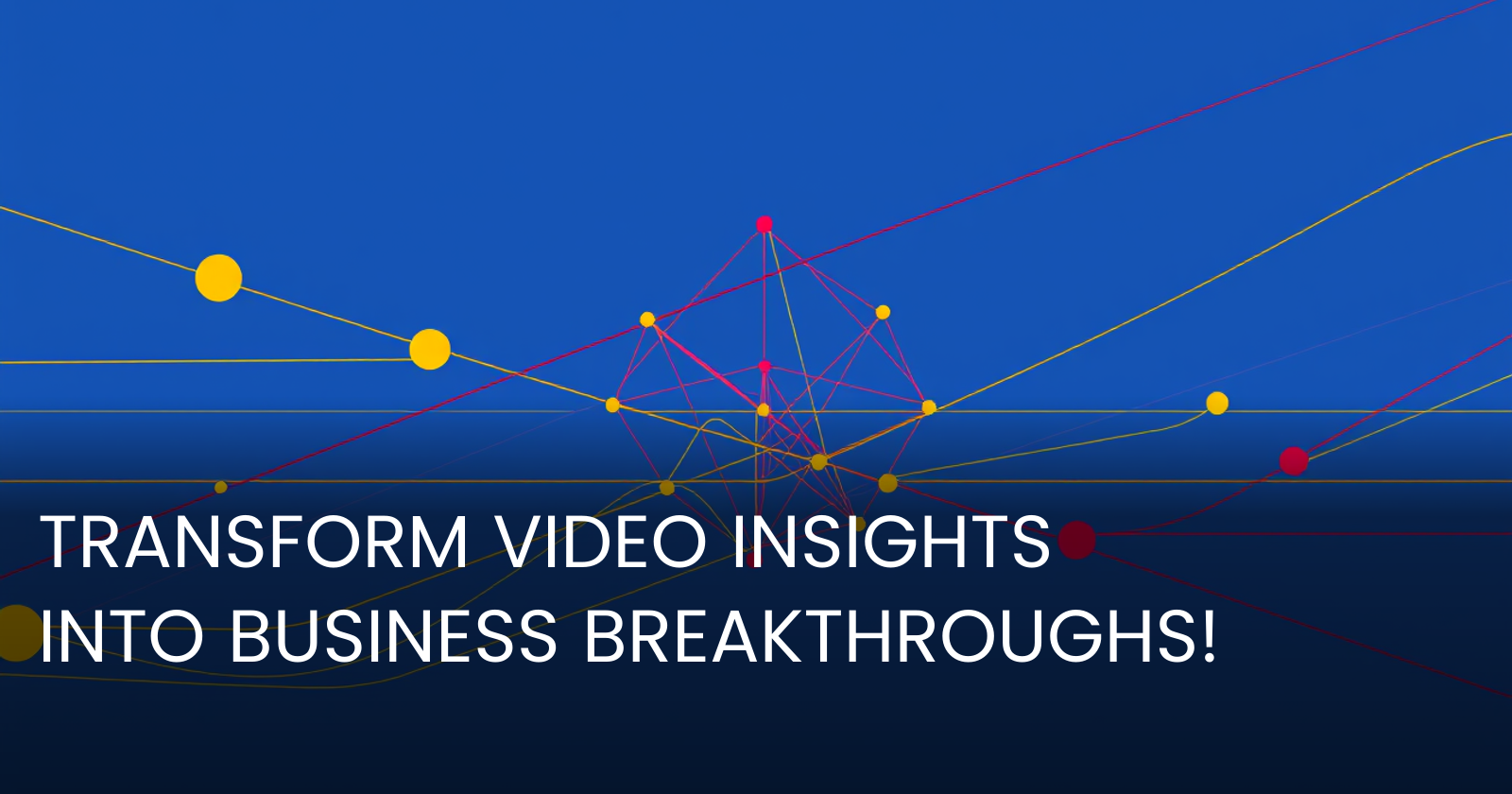
Welcome to our all-encompassing dive into a fascinating scientific paper: "Efficient Transfer Learning For Video-Language Foundation Models." Our goal is to break down the complex concepts from the paper into straightforward explanations. As we do this, we'll explore the possibilities this technology unveils for companies, from optimizing their processes to potentially unveiling new revenue streams.
- Arxiv: https://arxiv.org/abs/2411.11223v1
- PDF: https://arxiv.org/pdf/2411.11223v1.pdf
- Authors: Zhangxuan Gu, Jun Lan, Zhuoer Xu, Zhongcai Lyu, Yanshuo Wang, Yan Hong, Zizheng Huang, Haoxing Chen
- Published: 2024-11-18
Main Claims Summarized
The paper proposes a way to effectively fine-tune pre-trained video-language models to excel at video-specific tasks without compromising their general usability. The challenge addressed is catastrophic forgetting: While adding more parameters helps adapt these models to video tasks, it often erases the broad, transferable knowledge they come with. Enter the Multi-modal Spatio-Temporal Adapter (MSTA) – the proposed solution that balances this trade-off, preserving both general and task-specific knowledge.
New Proposals and Enhancements
The paper introduces MSTA, which aims to enhance the alignment of visual and textual representations in models like CLIP and ViCLIP. Alongside MSTA, a spatio-temporal description-guided consistency constraint is proposed—essentially a way to guide learning with richer and more descriptive narratives about videos. This consistency mechanism mitigates overfitting and bolsters the model's ability to generalize from a small dataset.
Ways Companies Can Use This Technology
For businesses operating in fields that require video recognition, this research has direct product and process optimization implications. Let's consider some ideas:
Enhanced Customer Interaction Tools: Platforms that offer video-based customer service could implement these robust video-language capabilities to better understand and respond to customer actions and moods in real-time.
Smart Surveillance and Security: Security companies can develop systems that automatically recognize suspicious activities or specific actions, enhancing surveillance efficiency without human intervention.
Sport and Performance Analysis: Sports analytics companies can offer tools that automatically generate detailed analytics of player performance using spatio-temporal models.
Content Moderation at Scale: Social media platforms and content providers can leverage such technologies for moderating video content, identifying undesirable activities with high accuracy.
Content Recommendation: Streaming services can utilize these models to understand the narrative of videos better, enhancing their recommendation systems with content similarity insights grounded in contextual understanding.
Training the Model: Datasets and Methodology
The robust pre-training of these models takes place on datasets like InternVid-10M, containing vast amounts of video-text pairs. The pre-trained models are further fine-tuned on widely-known datasets like Kinetics-400, Kinetics-600, UCF-101, and more. These datasets encompass varied video actions, helping the model develop a nuanced understanding across diverse scenarios.
Hardware Required: What Does it Take?
To train these sophisticated models and adapters, considerable hardware is necessary—especially GPUs like the Tesla A100-80G, known for their computational prowess. These are crucial given the model's reliance on processing large datasets. The setup described includes parallel training across multiple GPUs to handle the immense computations efficiently.
Comparing to Other State-of-the-Art Approaches
MSTA stands as a refined approach in the landscape of transfer learning for video-language tasks. Unlike its predecessors, which often overemphasize flexibility at the cost of generalizability, MSTA offers a balanced path. It achieves superior performance in both zero-shot and few-shot settings, outshining state-of-the-art methods like XCLIP or ActionCLIP which typically employ stronger modification of network structures at the risk of losing foundational knowledge.
Conclusions and Areas for Improvement
In summary, the method promises an optimized middle ground for video recognition tasks, with the aptness of using minimal parameters. Yet, there are still areas that can be honed further. Future directions could involve refining the training processes involved, ensuring every gradient shared between the visual and textual branches augments performance optimally. There's also the aspect of description quality for training—improving the automated generation of video descriptions to bolster consistency constraints could achieve better results.
With these insights, businesses can derive strong foundational technology enhancements and innovate in ways aligned with the demands of processing video content, applying the principles explored herein to unleash novel opportunities for growth and efficiency.
Subscribe to my newsletter
Read articles from Gabi Dobocan directly inside your inbox. Subscribe to the newsletter, and don't miss out.
Written by
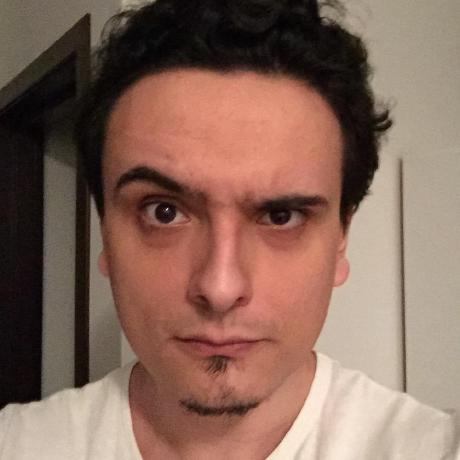
Gabi Dobocan
Gabi Dobocan
Coder, Founder, Builder. Angelpad & Techstars Alumnus. Forbes 30 Under 30.