What SAMURAI Can Do For Your Company: Unlocking New Potential in Visual Object Tracking
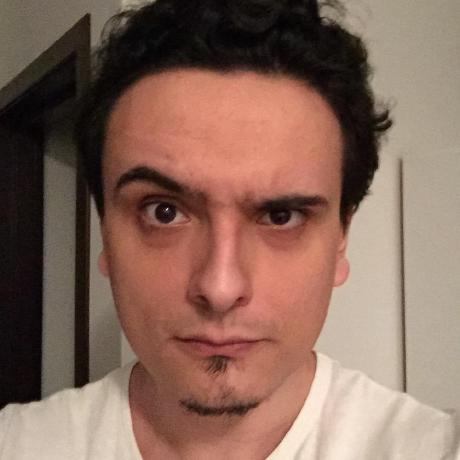

Welcome to our exploration of the research paper "SAMURAI: Adapting Segment Anything Model For Zero-Shot Visual Tracking With Motion-Aware Memory". This paper introduces an innovative way to tackle visual object tracking, a technology with vast potential for business applications. We'll break down the complex concepts, highlight potential business implications, and set the stage for leveraging these advancements in your own operations.
- Arxiv: https://arxiv.org/abs/2411.11922v1
- PDF: https://arxiv.org/pdf/2411.11922v1.pdf
- Authors: Jenq-Neng Hwang, Zhongyu Jiang, Wenhao Chai, Hsiang-Wei Huang, Cheng-Yen Yang
- Published: 2024-11-18
Main Claims of the Paper
The researchers present SAMURAI, an adaptation of the Segment Anything Model 2 (SAM 2), focusing particularly on visual object tracking—a scenario where objects are continuously monitored across a series of frames in a video. The paper claims that SAMURAI significantly enhances SAM 2's capabilities, overcoming hurdles like error propagation and inaccuracies in dense or complex scenes. The core breakthrough lies in SAMURAI's ability to provide zero-shot performance, meaning it can effectively track objects without needing additional training or fine-tuning, which in itself is a huge advantage for practical, real-time applications in dynamic environments.
New Proposals and Enhancements
SAMURAI introduces two primary advancements over SAM 2: motion modeling and a motion-aware memory selection mechanism.
Motion Modeling System: This addition enhances the predictability of an object's future position by taking into account historical movement data, minimizing confusion in scenarios where multiple objects share similar appearances. A Kalman Filter, a well-known algorithm for predicting system states over time, is incorporated to improve accuracy in selecting tracking masks.
Motion-Aware Memory Selection: This new mechanism selectively retains relevant past information, based on a mix of motion and affinity scores, instead of indiscriminately storing recent frames. This leads to fewer errors and better performance in crowded and complex scenes.
Leveraging SAMURAI: Potential Business Applications
By understanding how SAMURAI improves object tracking, businesses can harness this technology to optimize various processes and create new revenue streams. Some areas of potential application include:
Retail and Inventory Management: Implement real-time monitoring of inventory in warehouses via automated visual tracking, ensuring precise stock levels and reducing losses due to human error or theft.
Autonomous Vehicles: Enable better path planning and decision-making by accurately tracking other vehicles, pedestrians, and obstacles regardless of how crowded or dynamic the streets might be.
Surveillance and Security: Enhance security systems with real-time tracking capabilities that can differentiate between similar objects and track individuals or items across different cameras without manual supervision.
Sports Analytics: Provide detailed player and ball tracking for live sports analysis, offering insights into player movements and strategies without needing extensive pre-training of models.
Healthcare Monitoring Systems: Utilize non-invasive tracking of patients and hospital equipment, which can alert staff to movements that might indicate falls or unauthorized usage.
Training the Model: Dataset and Process
The SAMURAI model enhances visual tracking using SAM 2's framework but does not require additional training specific to SAMURAI itself. Instead, it uses existing datasets to tune the motion and memory modules. Key datasets include LaSOT, LaSOText, and GOT-10k, which provide diverse environments and object interactions for testing SAMURAI's robustness.
Hardware Requirements
For training and deploying visual tracking models like SAMURAI, robust hardware is vital due to the computational demands of processing video data. The experiments reported were conducted using high-performance GPUs, such as an NVIDIA RTX 4090, ensuring smooth operation even in real-time scenarios.
Comparison with State-of-the-Art Alternatives
SAMURAI shows significant improvements when benchmarked against other state-of-the-art models. Thanks to its innovative motion-aware mechanics, SAMURAI outperforms traditional models which often require retraining for each unique scenario:
- Performance: Clinches greater accuracy and reliability, surpassing other models even under challenging conditions of occlusions and rapid motion.
- Versatility: Goes beyond typical tracking systems by eliminating extensive pre-training needs, making it adaptable to various applications straight from the deployment phase.
Conclusions and Areas for Improvement
The SAMURAI model presents a breakthrough in zero-shot visual tracking by integrating motion awareness into the Segment Anything framework. It maintains competitive edge performance while operating efficiently without retraining, which is a game-changer for companies looking to implement cutting-edge machine learning solutions.
However, the paper also hints at possible future enhancements. They suggest further exploration into diverse motion modeling techniques or hybrid systems that combine heuristic and deep learning methodologies. As real-world applications rapidly evolve, these improvements could refine SAMURAI's adaptability even further.
In summary, SAMURAI offers a robust, efficient, and scalable solution for industries heavily reliant on accurate object tracking, with minimal adaptation costs and maximum flexibility. Staying ahead in these technological advancements can significantly position companies to unlock new efficiencies and revenue opportunities in their respective fields.
Subscribe to my newsletter
Read articles from Gabi Dobocan directly inside your inbox. Subscribe to the newsletter, and don't miss out.
Written by
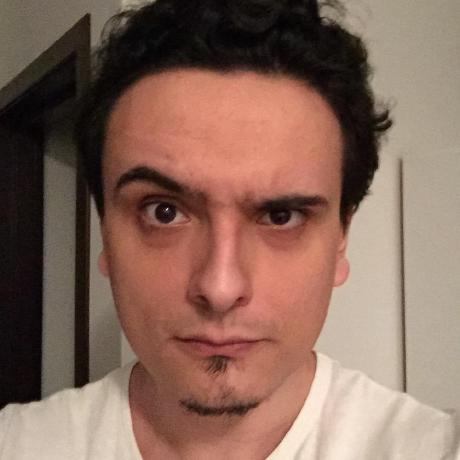
Gabi Dobocan
Gabi Dobocan
Coder, Founder, Builder. Angelpad & Techstars Alumnus. Forbes 30 Under 30.