Simplifying Progressive Generalization Risk Reduction for Causal Effect Estimation
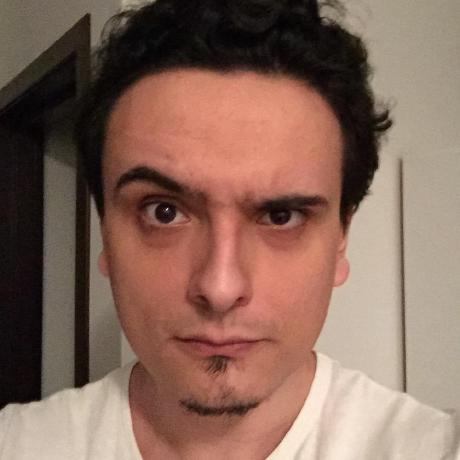

Imagine you are embarking on a journey into the complex world of predicting the effects of actions without having a perfect "what if" scenario at hand. This blog post breaks down a dense piece of research from the University of Queensland that proposes a new way to improve how we estimate these effects using machine learning, even when data is limited and expensive to gather. Whether you're a healthcare institution, a business analyst, or a curious machine learning enthusiast, understanding this innovation could open new doors to more accurate decision-making and better financial outcomes.
- Arxiv: https://arxiv.org/abs/2411.11256v1
- PDF: https://arxiv.org/pdf/2411.11256v1.pdf
- Authors: Hongzhi Yin, Shazia Sadiq, Li Kheng Chai, Guanhua Ye, Tong Chen, Hechuan Wen
- Published: 2024-11-18
Unpacking the Research
When researchers talk about "Causal Effect Estimation" (CEE), they mean the ability to look at data and predict what would happen if we could change a particular factor, like switching a patient's medication. Typically, this kind of prediction demands tons of data that's perfectly aligned, which is rarely available, especially in critical fields like healthcare or finance. That's where this paper steps in.
Key Claims and Solutions
The researchers identify a problem: Despite the promise of CEE, the scarcity of well-labeled data due to costs, ethics, and time makes its benefits hard to harness. They propose a novel approach to acquire better data incrementally, called "Model Agnostic Causal Active Learning" (MACAL). This method is designed to smartly select which data to label in a way that minimizes risks and maximizes the reliability of CEE, all while working within budget constraints.
Practical Benefits for Companies
Whether you're in healthcare estimating treatment results, or in retail evaluating the effects of marketing strategies, MACAL could transform your operations. The method allows you to make informed decisions faster and with less data, reducing costs and ethical concerns linked to vast data collection. Early adopters could use this to improve service efficiency, enhance customer satisfaction by tailoring experiences, and ultimately, boost revenue.
Diving Into the Methodology
Training and Datasets
Training a CEE model with MACAL starts small, with a limited set of labeled data, which grows wisely as more data becomes labeled over time. The paper mentions using a mix of real-world and synthetic datasets for testing, like IHDP, IBM, and CMNIST, each offering a unique challenge and helping validate the proposed method's reliability.
Hardware Requirements
The experiments leveraged GPUs like the NVIDIA A40, pointing to the need for considerable processing power. For companies, this means ensuring access to robust computational resources if they plan to implement techniques similar to MACAL at scale.
Innovations and Comparisons
Compared to existing methods, MACAL shines in its dual focus: it not only reduces the model's uncertainty but also ensures the even representation of treatment groups in its data, respecting the crucial assumption of positivity in causal analysis. Other approaches tend to neglect one of these aspects, leading to less reliable predictions.
Conclusions and Future Directions
MACAL outperformed current state-of-the-art methods across several datasets, proving more consistent in providing accurate causal estimates. However, like any pioneering research, there's room for refinement. For instance, the computational demands are still relatively high, and the method's full potential across diverse application domains has yet to be unlocked.
Open Avenues for Improvement
Future work could focus on reducing the computational complexity further, facilitating easier adoption by smaller businesses with limited access to high-end computing resources. Also, exploring synergies with other emerging AI techniques could enhance its adaptability and effectiveness across different fields.
Wrapping It Up
In simple terms, this research offers a new, smarter way to predict critical outcomes without needing endless amounts of perfect data, balancing both practicality and innovative machine learning strategies. As businesses strive to make data-driven decisions in complex environments, employing methodologies like MACAL might just be the key to staying ahead of the curve, ensuring both economic and ethical success.
Subscribe to my newsletter
Read articles from Gabi Dobocan directly inside your inbox. Subscribe to the newsletter, and don't miss out.
Written by
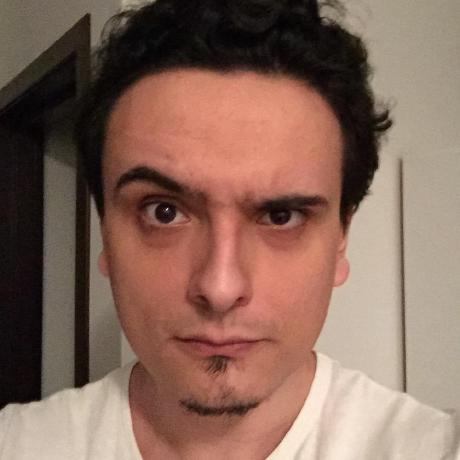
Gabi Dobocan
Gabi Dobocan
Coder, Founder, Builder. Angelpad & Techstars Alumnus. Forbes 30 Under 30.