Exploring the Role of Machine Learning in Rainfall Prediction
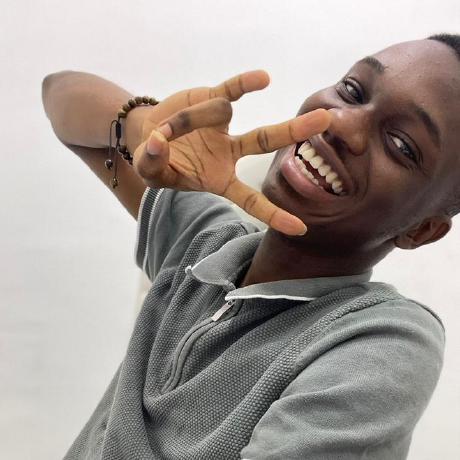
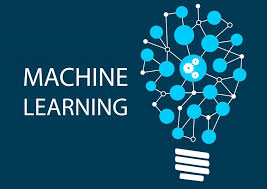
A Lagos, Nigeria Case Study
Rainfall prediction has always been a cornerstone of effective planning in agriculture, water resource management, and disaster preparedness. However, in regions like Lagos, Nigeria, where rainfall patterns are marked by extreme variability and complex interactions, traditional forecasting methods often fall short. This is where machine learning (ML) steps in as a game-changer. In this blog, we explore how ML techniques can revolutionize rainfall prediction for Lagos, shedding light on their immense potential and critical applications.
The Significance of Rainfall Prediction in Lagos
Lagos, a bustling coastal city, is heavily influenced by the West African Monsoon and the Intertropical Discontinuity (ITD). These climatic factors result in high variability in rainfall patterns, impacting agriculture, water management, and urban planning. Accurate rainfall forecasting in Lagos is essential for:
Agriculture: Guiding planting schedules and irrigation.
Flood Mitigation: Enabling early warnings and preventive measures.
Urban Planning: Informing infrastructure development and disaster management.
Challenges in Traditional Rainfall Forecasting
Conventional methods, such as statistical models and numerical weather prediction (NWP), have been widely used but struggle with:
Nonlinear Dynamics: Difficulty in modeling complex interactions in meteorological data.
Localized Events: Poor accuracy in predicting microclimatic conditions.
Data Limitations: Dependence on sparse or inconsistent observational data.
Machine Learning to the Rescue
Machine learning leverages historical data and computational algorithms to uncover patterns and improve predictive accuracy. This study analyzed ML's role in rainfall prediction, focusing on Lagos, using data spanning 2002 to 2013. Here’s how it works:
1. Data Preparation
Meteorological variables like precipitation, temperature, humidity, and wind speed were collected from weather stations and satellite sources. The data underwent preprocessing, including cleaning, handling missing values, and feature engineering to create meaningful predictors.
2. Model Selection
Three ML models—Random Forest, Gradient Boosting, and Neural Networks—were evaluated for their ability to predict rainfall:
Random Forest: Emerged as the most effective, excelling in managing nonlinear relationships and feature interactions.
Gradient Boosting: Performed well with sequential optimization of errors.
Neural Networks: Captured temporal and spatial patterns but were sensitive to data preprocessing and hyperparameter tuning.
3. Performance Metrics
The models were assessed using:
Mean Absolute Error (MAE)
Root Mean Squared Error (RMSE)
R-squared values
Among the three, Random Forest achieved the highest accuracy, demonstrating the robustness required for practical applications.
Real-World Applications
The benefits of integrating ML into rainfall forecasting for Lagos are vast:
Agriculture: Farmers gain precise information for crop cycles, improving yields and minimizing losses.
Flood Preparedness: Enhanced predictions enable proactive measures to mitigate flooding impacts.
Urban Infrastructure: Accurate forecasts inform city planners, reducing risks associated with extreme weather events.
Future Directions
To fully harness ML's potential, the study recommends:
Data Quality Enhancement: Investing in automated weather stations and integrating diverse data sources like satellite imagery.
Hybrid Models: Combining ML with traditional NWP for greater accuracy.
Real-Time Systems: Deploying cloud-based platforms for dynamic updates and predictions.
Explainable AI: Implementing interpretability tools like SHAP and LIME to increase trust in ML predictions.
Conclusion
Machine learning holds transformative potential for operational meteorology in Lagos. By addressing the limitations of traditional methods and offering unparalleled predictive accuracy, ML can significantly impact sectors reliant on weather forecasting. As climate variability intensifies, integrating adaptive, data-driven techniques like ML into rainfall prediction systems will be crucial for resilience and sustainable development.
How do you see ML transforming weather predictions in your region? Share your thoughts below!
Subscribe to my newsletter
Read articles from Samuel Damilola Akinmarin directly inside your inbox. Subscribe to the newsletter, and don't miss out.
Written by
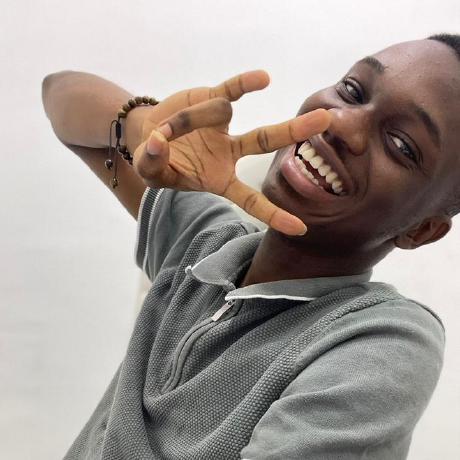
Samuel Damilola Akinmarin
Samuel Damilola Akinmarin
I'm a Software Engineer, Meteorologist, Graphic design, Content Creator, and Community Engineer. I'm passionate about sharing knowledge, documentation, web engineering, Jamstack, headless commerce, and others. I create technical content (on my YouTube), build useful open-source projects, speak at some meetups/conferences, build technical communities... Here's a quick summary about me: 😊 Pronouns: He/him 💡 Fun fact: I'm currently studying at AltSchool Africa School of Software Engineering Class of 2022. 🌱 I’m currently learning JavaScript, Reactjs, and Python. 😊 I’m looking for help with open source projects, hackathons, internships, and entry-level opportunities. 💼 Job interests: Software Engineer, Front Engineer, or UI Engineer (Intern or Junior level). 📫 You can view my resume and contact me by emailing Youngsamsy.1@gmail.com.