It's Time for AI Research to Embrace Evidence Over Speculation

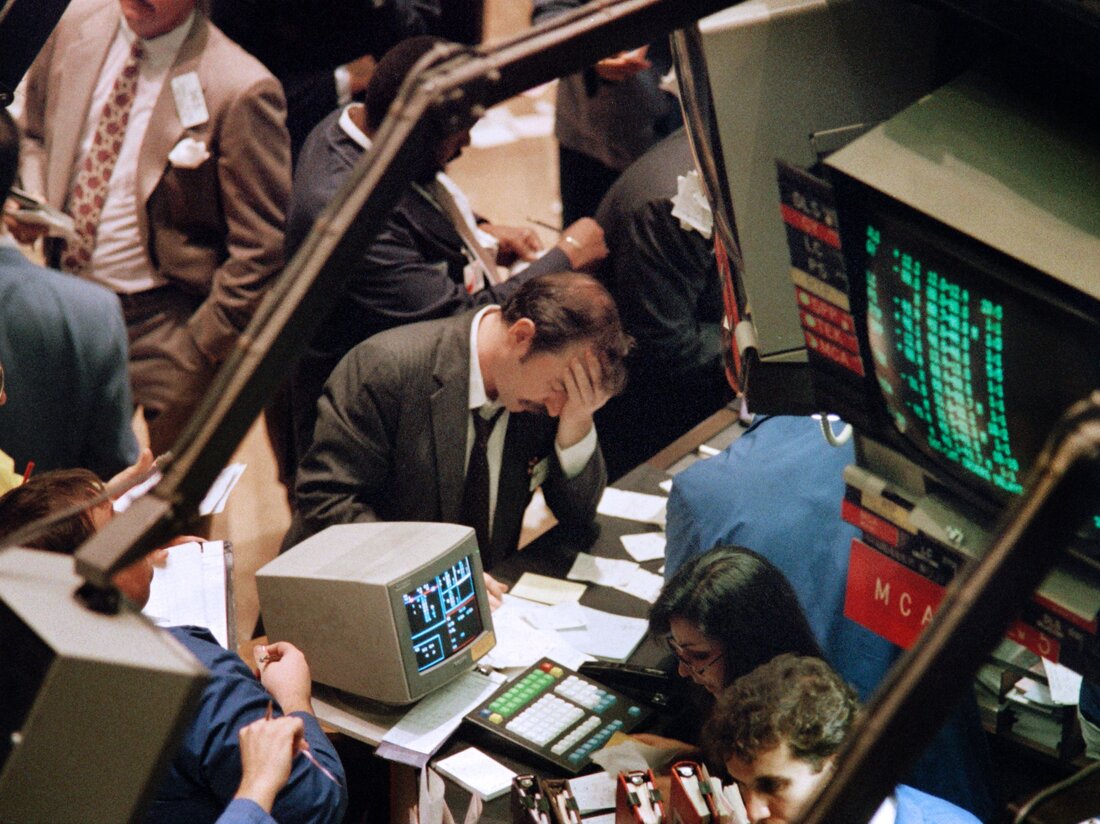
The current trajectory of AI research risks being undermined by a troubling pattern: the rush to adopt speculative methods and terminology without demanding the evidence to back them up. Techniques like in-context learning (ICL) and chain-of-thought (CoT) reasoning have garnered significant attention, but their underlying assumptions remain unfalsified and, in some cases, easily disproven. This complacency threatens the integrity of the field and undermines its progress.
It is time for the AI community to pause, reflect, and recalibrate its priorities toward rigor, transparency, and principled methodologies.
The Problem: Speculation Without Evidence
Flawed Assumptions Persist Unchallenged
Many popular methods operate under assumptions that can be tested—and disproven—using simple, transparent setups. For example:
A model with random, untrained weights will fail at both ICL and CoT reasoning, proving that these techniques rely on latent patterns shaped by pre-training data
Despite this, researchers continue to promote these techniques as if they independently generate reasoning capabilities, ignoring the dependency on pre-training
Unfalsifiable Claims Dominate
Techniques like CoT and ICL remain shielded from rigorous critique because their effectiveness is conflated with the underlying model's latent capabilities:
Researchers rarely isolate whether performance gains stem from genuine improvements or pre-existing knowledge embedded during pre-training
Cherry-picked successes obscure broader limitations, creating a distorted view of these techniques' efficacy
Short-Termism in AI Research
The obsession with outperforming benchmarks fuels a culture of overfitting and superficial gains:
Reinforcement learning (RL) applied to CoT reasoning risks over-optimizing for specific tasks without addressing generalization
Techniques like test-time training add computational inefficiency and further overfit to narrow benchmarks rather than addressing core deficiencies in model design
Missed Opportunities: A Call for Evidence-Based Claims
The rush to adopt speculative methods comes at a cost. By failing to prioritize falsifiable, evidence-based approaches, the community overlooks opportunities to deepen our understanding of large language models (LLMs) and their limitations.
Latent Space Over Inputs
The primary determinant of LLM behavior is the pre-trained latent space, not the inputs themselves. Techniques like CoT and ICL merely exploit these pre-existing patterns, amplifying their utility. Misattributing their success to the prompts alone diverts attention from the more meaningful question: How do we better construct, interrogate, and refine latent spaces during pre-training?
Transparency and Reproducibility
Simple, transparent setups—like comparing trained and untrained models—can easily test claims about prompting techniques. Yet, such tests are often omitted in research, allowing speculation to proliferate unchecked. By demanding such comparisons, the community can bring clarity to debates and ground assumptions in evidence.
The Path Forward: A Call to Action
The AI community must adopt a more principled approach, prioritizing rigor and transparency over speculative claims. Here are key steps to achieve this:
1. Rethink Evaluation Metrics
Move beyond static benchmarks that reward narrow, task-specific gains. Develop dynamic, open-ended evaluation frameworks that prioritize generalization, robustness, and adaptability.
2. Embrace Falsifiability
Encourage research that rigorously tests the assumptions behind popular techniques. Require baseline comparisons using untrained models to distinguish between inherent model capabilities and the effects of input techniques.
3. Reward Negative Results
Shift the incentive structure to value transparency and critique. Celebrate researchers who identify limitations in popular methods, fostering a culture that values truth-seeking over performance hacking.
4. Reclaim the Narrative
Demand precision in terminology and rigor in claims. Techniques like CoT and ICL should not be described as independent reasoning methods until they are definitively proven to generate new capabilities, rather than leveraging pre-trained patterns.
A Time for Reflection
The AI research community stands at a crossroads. It can continue down the path of speculative methods, superficial benchmarks, and short-termism—or it can recommit to the principles of scientific inquiry: transparency, falsifiability, and a focus on meaningful progress. The choice we make will define not just the future of AI, but also its impact on society as a whole.
Now is the time to pause, reflect, and demand better. Let's build a field rooted in evidence, one that values understanding over speculation and rigor over hype. The integrity of AI research—and its transformative potential—depends on it.
Subscribe to my newsletter
Read articles from Gerard Sans directly inside your inbox. Subscribe to the newsletter, and don't miss out.
Written by

Gerard Sans
Gerard Sans
I help developers succeed in Artificial Intelligence and Web3; Former AWS Amplify Developer Advocate. I am very excited about the future of the Web and JavaScript. Always happy Computer Science Engineer and humble Google Developer Expert. I love sharing my knowledge by speaking, training and writing about cool technologies. I love running communities and meetups such as Web3 London, GraphQL London, GraphQL San Francisco, mentoring students and giving back to the community.