Streamlining AI: Fast Video Diffusion Revolution
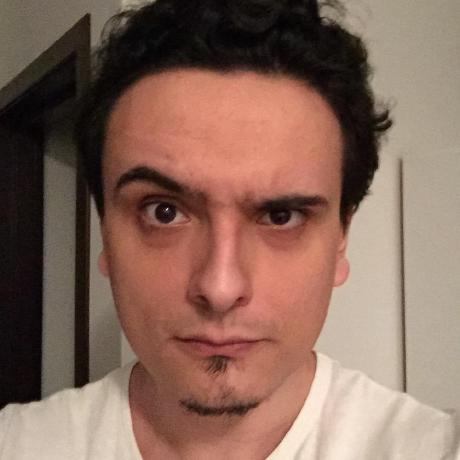

Introduction
In the realm of AI-driven creativity, transforming text prompts into vivid video content has become the latest frontier. This transformation owes much to video diffusion models, which offer a bridge between imaginative concepts and their visual portrayal. Yet, these models have hit a snag; their thirst for computational power and massive amounts of memory can be daunting, barricading many potential applications behind a wall of technical limitations.
Enter Streamlined Inference, an innovative framework that whispers promises of laying this barrier to rest. Designed to reduce the heavyweight needs of video diffusion models, this approach eliminates the need for additional training, cleverly harnessing the temporal and spatial elements within video generation itself. With its prowess, it makes the creation of high-resolution, long-duration videos via standard consumer GPUs a feasible reality. But how exactly does it work its magic, and why should companies eagerly observe?
- Arxiv: https://arxiv.org/abs/2411.01171v1
- PDF: https://arxiv.org/pdf/2411.01171v1.pdf
- Authors: Yanzhi Wang, Wei Niu, Pu Zhao, Geng Yuan, Changdi Yang, Zhenglun Kong, Zichong Meng, Yifan Gong, Yushu Wu, Zheng Zhan
- Published: 2024-11-02
Breaking Down the Paper's Claims
The paper under scrutiny, “Fast And Memory-Efficient Video Diffusion Using Streamlined Inference,” embarks on confronting the hurdles faced by current video diffusion models. When diving into AI-generated content, these models notably push the envelope concerning graphical beauty and realism. Yet, they do so while attracting computational and memory-heavy criticisms, often insurmountable for the everyday tech user.
The core challenge identified is the intense resource requirement, primarily stemming from needing to output multiple video frames together and the computationally extensive denoising steps inherent in video processing. To counteract this, the authors propose their novel "Streamlined Inference" framework, aimed at significantly easing memory loads without compromising video quality or speed.
Innovations Unveiled: What's New?
At the heart of this framework lie three cutting-edge techniques: Feature Slicer, Operator Grouping, and Step Rehash. Each serves a distinct purpose while complementing the others to produce an efficient inference process.
Feature Slicer
The first innovation, Feature Slicer, acts like a meticulous chef with a precision knife, slicing input features in both spatial and temporal layers. By breaking down these features into smaller parts, the framework manages to diminish the peak memory usage significantly. However, true efficiency isn't just about minimizing storage but about optimizing how these parts are subsequently processed — this brings us to the next innovation.
Operator Grouping
Operator Grouping steps in as the thoughtful organizer. By bundling homogeneous operators together, it allows intermediate results from sub-features to proceed through subsequent operators without requiring the typically needed consolidation step that consumes memory space. This method not only diminishes space usage but also boosts parallel processing capabilities, a cocktail that results in faster computations.
Step Rehash
Finally, Step Rehash capitalizes on the observed similarity between steps in the video diffusion process. By recognizing the likeness between consecutive processing steps, it smartly skips redundant computations, speeding up the process while keeping memory usage stable.
Practicality for Businesses: Reducing Costs, Amplifying Capability
For companies seeking to leverage AI for video content creation, the implications of this research are exciting. It potentially democratizes access to high-quality video generation by reducing the computational and financial barriers often linked with heavy AI models.
Enabling New Business Models
Affordable Video Transformation Platforms: Businesses can create platforms that allow users to transform script-based input into full-fledged videos, capturing market territory previously inaccessible due to prohibitive costs tied to high-end GPUs.
Creative Toolkits for Filmmakers: Streamlined Inference can catalyze a practical toolkit for filmmakers, allowing them to test scene ideas visually, without costly shootings or tech setups.
Advertising Innovations: Rapidly generate high-resolution ad content tailored to the messaging of specific audiences, allowing for hyper-targeted ad campaigns at a fraction of traditional costs.
Optimizing Existing Processes
Content Delivery and Streaming: Utilizing streamlined video diffusion could minimize the computational burden on servers, enhancing the quality and reducing latency in content streaming platforms.
In-House Digital Content Development: Companies can more feasibly employ AI to generate internal content, ranging from training videos to customer engagement materials, ensuring cohesion with current branding efforts without straining technical resources.
Training and Running: The Technical Side
Training details are a lower priority here, as the streamlined framework praises itself for being training-free during inference. Instead, the focus turns to its compatibility and practicality on commonly available hardware, like NVIDIA’s 2080Ti.
Datasets and Model Training
The models assessed within this framework were not retrained but evaluated on datasets such as UCF-101 and MSR-VTT. These datasets are well-regarded benchmarks in video content generation, ensuring that results obtain consistent, credible comparisons to industry standards.
Hardware Requirements
By drastically cutting peak memory usage from models like AnimateDiff’s 41.7GB to a palatable 11GB, the framework makes high-quality video generation viable on single consumer-grade GPUs. This accessibility matters tremendously, inviting more creators and companies to explore AI video generation.
Benchmark Comparisons: Leading the Pack
In testing, Streamlined Inference holds its ground against existing models, achieving comparable quality metrics like CLIP-Score and FVD with markedly reduced computational requirements. While alternatives like DeepCache share a similar premise of enhancing efficiency, Streamlined Inference demonstrates superior memory and speed optimizations without compromising video quality.
Conclusions and the Road Ahead
The paper’s results are promising, showcasing that Streamlined Inference opens up exciting opportunities for AI-driven video content creation at scale. Yet, there's always room for improvement. Future work might explore even more aggressive memory reduction strategies or delve deeper into optimizing specific video generation scenarios, including particular genres or lengths.
In closing, this novel approach offers a beacon of hope for harnessing AI to its full potential without succumbing to the demands of extensive computing and memory. Companies eyeing a future where video content rules supreme may very well find their path paved more smoothly than anticipated. It’s a testament to the profound impact streamlined, efficient inference can have across industries, providing everyone the creative latitude AI has long promised.
Subscribe to my newsletter
Read articles from Gabi Dobocan directly inside your inbox. Subscribe to the newsletter, and don't miss out.
Written by
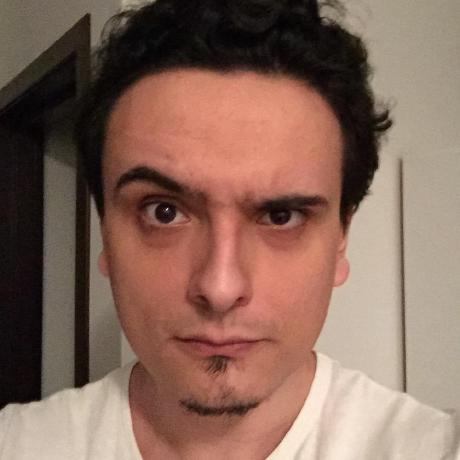
Gabi Dobocan
Gabi Dobocan
Coder, Founder, Builder. Angelpad & Techstars Alumnus. Forbes 30 Under 30.