Understanding the Role of MRI Image Quality in Brain Analysis
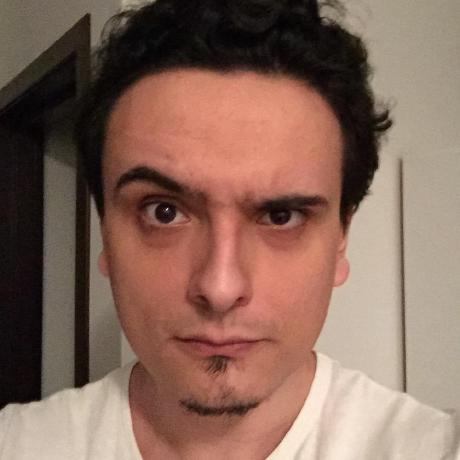
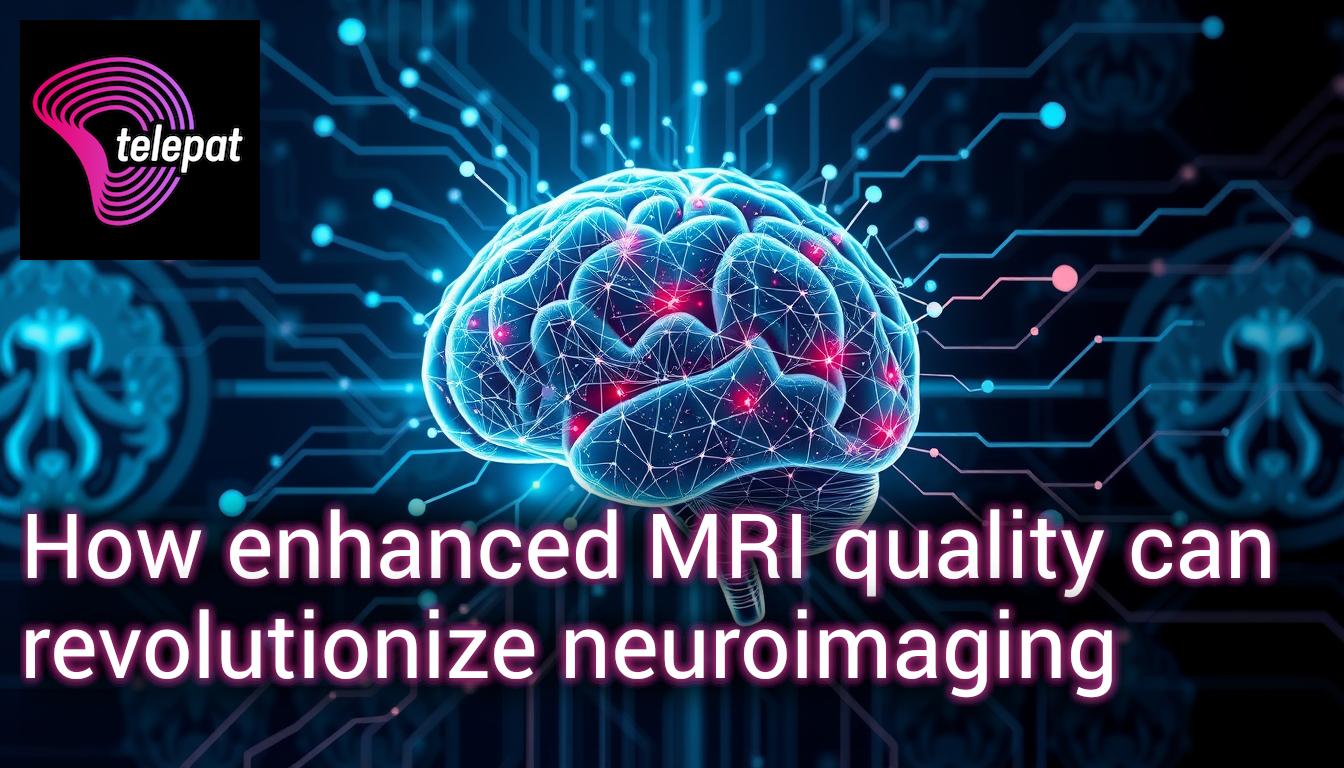
Introduction to MRI and Voxel-Based Morphology
Magnetic Resonance Imaging (MRI) is a pivotal tool in neuroscience for visualizing the human brain's structure. It provides insights into brain morphology, which involves analyzing features like local volumes and cortical thickness. This information is crucial for understanding various neurological conditions and conducting research on brain functionality.
However, the quality of MRI images plays a significant role in the accuracy of the data derived from them. Artifacts from within-scanner head movements, for instance, can distort measurements, impacting studies that rely on this data. The paper titled "The Impact Of MRI Image Quality On Statistical And Predictive Analysis Of Voxel Based Morphology" delves into how image quality affects scientific interpretations and predictive analytic outcomes in voxel-based morphology (VBM).
This piece provides simplified insights from the paper, aimed at a broader audience. It explores how enhanced MRI image quality can unlock new opportunities for companies and improve the accuracy of neuroimaging research.
- Arxiv: https://arxiv.org/abs/2411.01268v1
- PDF: https://arxiv.org/pdf/2411.01268v1.pdf
- Authors: Kaustubh R. Patil, Simon B. Eickhoff, Nicolás Nieto, Felix Hoffstaedter
- Published: 2024-11-02
Main Claims
1. Image Quality Significantly Affects Data Analysis
The study reveals that poor quality MRI images significantly degrade the sensitivity of statistical analyses when identifying gender differences within brain features. This effect is more pronounced in studies with smaller sample sizes.
2. Machine Learning Applications Show Slight Variability
For machine learning applications, while sample size and image quality influence outcomes, the effect is less pronounced than in classical statistical methods. Machine learning models show resilience to variability in image quality, though higher quality data can enhance classification accuracy.
3. Quality Control is Essential
For univariate analyses, stringent quality control measures are more beneficial than in multivariate or machine learning contexts. The paper suggests that better image quality increases the likelihood of detecting significant effects, especially with smaller datasets.
New Proposals and Enhancements
Automated Quality Assurance
The paper highlights the feasibility of automated quality assurance models. These models aim to substitute labor-intensive manual inspections, which are prone to error, especially with large datasets. Implementing automated systems could revolutionize data processing in neuroimaging by providing more consistent and reliable quality assessments.
Leveraging Image Quality Metrics
The paper proposes using quantitative image quality metrics, like the Image Quality Rating (IQR), to systematize filtering processes in MRI datasets. Such metrics provide a standardized approach to evaluating and deciding the usability of MRI scans, potentially making manual assessments obsolete.
Business Opportunities and Applications
The implications of this study extend beyond academic interest, offering commercial opportunities for companies specializing in medical imaging, AI, and healthcare solutions.
Enhanced Diagnostic Tools
Companies could create advanced diagnostic tools that rely on high-quality MRI images. These tools can more accurately detect neurological conditions and provide clearer insights into brain abnormalities.
Improved AI Models
Developing AI models equipped with mechanisms to handle varying image quality could lead to more reliable predictions and analyses in healthcare. These models would be invaluable in developing personalized medical treatments and therapies based on brain morphology data.
Streamlined Quality Assessment Services
There is a potential market for services offering automated MRI image quality assessments, which could be marketed to hospitals and research institutions. These services could make processing large datasets faster and more reliable, saving time and reducing errors inherent in human assessment.
Training the Model: Datasets and Techniques
Datasets Utilized
The paper analyzed data from three significant public datasets:
- Southwest University Adult Lifespan Dataset (SALD) with 494 participants.
- Enhanced Nathan Kline Institute Rockland Sample (eNKI) with 818 participants.
- Cambridge Center for Ageing and Neuroscience (CamCAN) with 651 participants.
These datasets span a wide age range (18 to 80 years) and represent diverse populations, providing a robust foundation for testing the effects of image quality.
Methodology
Data preprocessing was conducted using CAT 12.8.1 software, which provided both image quality ratings (IQR) and voxel-wise measurements. The study stratified participants into different subgroups based on image quality (low, high, and random) and balanced samples across age and gender.
Statistical analysis applied Bonferroni correction to identify significant features, while logistic regression was employed for machine learning tests to predict gender differences from gray matter volume.
Hardware Requirements
While the paper does not specify detailed hardware requirements, analyzing and processing MRI images typically demands substantial computing power. Researchers and companies engaged in similar work can expect to utilize:
- High-performance computing clusters or powerful GPUs for data processing and machine learning tasks.
- Sufficient storage capacity to handle large datasets, as MRI data is both voluminous and complex.
- Comprehensive data governance solutions to meet compliance requirements for medical data handling.
State-of-the-Art Comparisons
Resilience to Image Quality Variations
Compared to other state-of-the-art alternatives, traditional volumetric approaches showed more resilience to variances in image quality. Surface modeling approaches, however, were more susceptible to variability, impacting their reliability in studies using lower-quality images.
Advancements in Automated Tools
The mention of tools like CAT12, which facilitate the automated assessment of MRI quality, illustrates the ongoing advancement in the field. Such tools stand at the frontier of replacing manual evaluations, marking a significant difference from older methodologies relying solely on human experts.
Conclusions and Areas for Improvement
Key Takeaways
Image quality is crucial: For traditional statistical analyses, high image quality significantly enhances the accuracy of detected effects. This is notably vital for studies operating with limited sample sizes.
Machine learning offers flexibility: While machine learning applications are somewhat less affected by image quality variations, maintaining high data quality still promises improvements in predictive performance.
Areas for Future Research
The study acknowledges that the images analyzed were largely of acceptable quality. Exploring a wider spectrum of image quality, including extremely poor samples, could provide deeper insights into how far machine learning models can stretch the boundaries of data quality.
Also, further development and refinement of automated image quality assessment tools would benefit the field and potentially expand into broader healthcare applications.
In essence, enhancing MRI image quality not only improves scientific studies but also fosters innovation in the medical field, creating pathways for new technologies and products that could help diagnose and treat neurological conditions more effectively. This research illuminates the critical role of data quality and opens up numerous possibilities for advancing the neuroscience domain.
Subscribe to my newsletter
Read articles from Gabi Dobocan directly inside your inbox. Subscribe to the newsletter, and don't miss out.
Written by
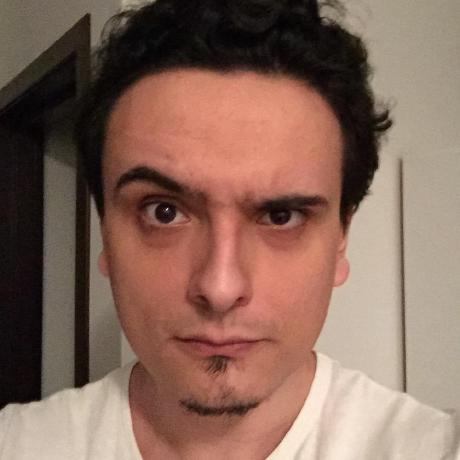
Gabi Dobocan
Gabi Dobocan
Coder, Founder, Builder. Angelpad & Techstars Alumnus. Forbes 30 Under 30.