The 3 Myths of AI Literacy: Common Misconceptions Holding Back True Understanding

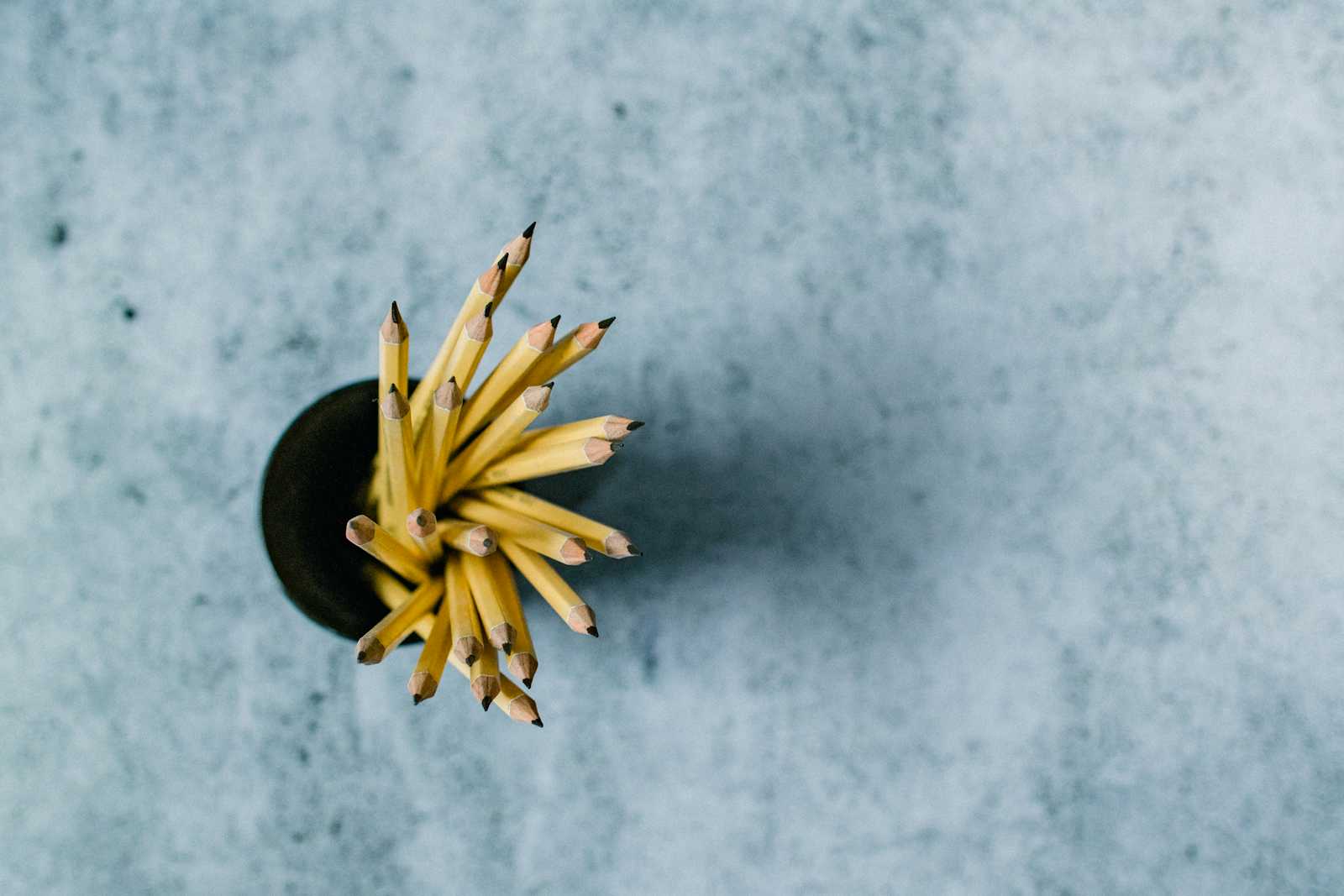
As artificial intelligence becomes increasingly integrated into our daily lives, a concerning pattern of misconceptions threatens to undermine our ability to effectively understand and utilise these powerful tools. Three particular myths stand out as especially problematic, creating barriers to genuine AI literacy and responsible deployment of AI systems.
Myth 1: The Anthropomorphism Trap
Perhaps the most pervasive and dangerous myth in AI literacy is our tendency to project human qualities onto AI systems. We casually describe AI as "thinking," "understanding," or possessing "intelligence" in ways that mirror human cognition. This anthropomorphization isn't just semantically incorrect – it fundamentally misshapes our understanding of AI capabilities and limitations.
When we attribute human-like reasoning to AI, we create false expectations about its decision-making process and capacity for genuine comprehension. An AI model doesn't "understand" context the way humans do; it processes patterns in data using statistical methods that, while sophisticated, are fundamentally different from human cognition.
Myth 2: The Prompt Engineering Adoration
The second myth centers on what I call "prompt evangelism" – the belief that mastering prompt engineering is the key to controlling and understanding AI behavior. While well-crafted prompts are undoubtedly important, this view drastically oversimplifies how AI systems actually work.
The reality is that an AI's responses are predominantly determined by its training data and architectural design, not just the prompts we feed it. Prompt engineering is more akin to learning the proper syntax for communication rather than fundamentally altering the AI's capabilities or knowledge base. This myth can lead to unrealistic expectations about what can be achieved through prompt optimization alone.
Myth 3: The Illusion of Knowledge Convergence
The third myth is particularly insidious: the assumption that AI outputs naturally align with human knowledge or truth. This "magical knowledge convergence" myth suggests that AI systems somehow distill objective truth from their training data, arriving at conclusions that reflect genuine human understanding.
This misconception ignores the fundamental nature of AI training: these systems are pattern-matching engines trained on human-created data, complete with all its biases, errors, and limitations. They don't "converge" on truth – they reproduce patterns found in their training data, regardless of whether those patterns represent accurate knowledge or common misconceptions.
Moving Forward: Building True AI Literacy
Dismantling these myths is crucial for developing genuine AI literacy. We need to understand AI systems for what they are: powerful pattern-matching tools that, while incredibly useful, operate in fundamentally different ways from human intelligence. This understanding allows us to better leverage AI's strengths while remaining cognizant of its limitations.
Real AI literacy requires moving beyond these comfortable but misleading anthropomorphic analogies and developing a more nuanced understanding of what AI actually is and does. Only then can we have meaningful discussions about AI deployment, safety, and governance that aren't clouded by misconception and myth.
The path forward involves education that emphasizes AI's actual mechanisms rather than relying on human-centric analogies. It requires us to be more precise in our language about AI capabilities and more realistic in our expectations of what these systems can achieve. Most importantly, it demands that we approach AI with a clear-eyed understanding of its nature as a technological tool rather than as a simulation of human intelligence.
Subscribe to my newsletter
Read articles from Gerard Sans directly inside your inbox. Subscribe to the newsletter, and don't miss out.
Written by

Gerard Sans
Gerard Sans
I help developers succeed in Artificial Intelligence and Web3; Former AWS Amplify Developer Advocate. I am very excited about the future of the Web and JavaScript. Always happy Computer Science Engineer and humble Google Developer Expert. I love sharing my knowledge by speaking, training and writing about cool technologies. I love running communities and meetups such as Web3 London, GraphQL London, GraphQL San Francisco, mentoring students and giving back to the community.