Unleashing a New Frontier in Medical Question-Answering: Rationale-Guided Retrieval Augmented Generation
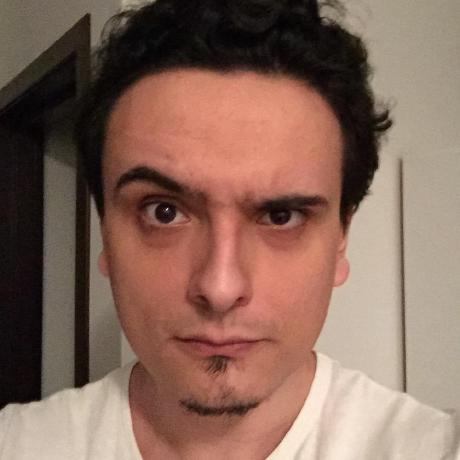

- Arxiv: https://arxiv.org/abs/2411.00300v1
- PDF: https://arxiv.org/pdf/2411.00300v1.pdf
- Authors: Jaewoo Kang, Hyunjae Kim, Mujeen Sung, Hyeon Hwang, Sihyeon Park, Chanwoong Yoon, Yein Park, Jiwoong Sohn
- Published: 2024-11-01
Introduction
In a groundbreaking paper, researchers from Korea University introduce a novel framework, called RAG2 (Rationale-Guided Retrieval Augmented Generation), to address the limitations of current large language models (LLMs) in biomedical applications. LLMs hold promise for tasks like medical question-answering (QA), but frequently grapple with issues like hallucinations—producing plausible but inaccurate information—and struggles to maintain up-to-date medical knowledge. RAG2 aims to solve these challenges by refining the retrieval and generation process within LLMs to ensure more reliable and accurate outputs.
Main Claims
The core claims of the paper are twofold: first, that RAG2 effectively improves the reliability and accuracy of existing LLMs in the biomedical domain. Second, RAG2 does so in a manner that outperforms current State-of-the-Art (SOTA) methods by reducing biases and enhancing the retrieval of pertinent information from various biomedical sources.
Innovative Enhancements
Rationale-Guided Filtering
A key innovation in RAG2 is the introduction of rationale-guided filtering. A smaller, dedicated model assesses whether integrating retrieved snippets with the LLM’s prompt would increase the model's confidence and accuracy. This filtering model is trained using perplexity-based labeling, which measures the change in a model's perplexity—a statistical measure of how well a probability model predicts a sample—when particular documents are introduced.
Rationale-Based Queries
Instead of relying solely on the initial query, RAG2 uses LLM-generated rationales to reformulate the query, thereby improving the relevance and utility of the retrieval process. This enables the model to pinpoint critical diagnostic clues more effectively, enhancing the retrieval system's performance.
Balanced Retrieval Strategy
RAG2 employs a balanced retrieval approach, drawing snippets equally from multiple biomedical corpora—such as PubMed, PMC, textbooks, and clinical guidelines—to mitigate the traditional retriever bias that tends to favor larger, more extensively trained corpora.
Applicability for Businesses
Leveraging RAG2 in Healthcare
For companies in the healthcare sector, RAG2 offers a potent tool for medical information retrieval and decision-making systems. Hospitals and clinics can deploy more reliable virtual assistants for supporting clinical diagnostics, benefiting both clinicians and patients by ensuring decisions are informed by comprehensive and relevant data.
Potential Products and Business Models
Telemedicine services can integrate RAG2 to enhance the accuracy of automated patient consultations. Pharmaceutical companies might employ this framework to streamline research by retrieving pertinent scientific literature and reducing time spent navigating vast repositories of medical information.
Training and Datasets
RAG2 builds upon existing LLMs, augmenting them with its retrieval and filtering framework. The training employs three well-established medical QA datasets: MedQA, MedMCQA, and MMLU-Med, each encompassing diverse medical topics and providing robust training and evaluation grounds.
Hardware Requirements
Training and implementing RAG2 requires substantial computational resources, but its filtering model is especially noteworthy for its efficiency. It is trainable on widely available GPUs like an RTX 3090, making it accessible for organizations with moderate computational infrastructure.
Comparison with State-of-the-Art Methods
RAG2 showcases an improvement in performance over traditional methods such as MedRAG and Adaptive-RAG by up to 6.1% in accuracy across multiple medical QA benchmarks. This significant gain highlights the effectiveness of RAG2’s rationale-based approach compared to models that lack sophisticated retrieval and filtering mechanisms.
Conclusions and Areas for Improvement
RAG2 marks a significant advancement in making LLMs more reliable and effective for critical biomedical QA tasks, positioning AI to contribute actively to medical decision-making processes. However, certain areas remain open for enhancement, including exploring RAG2's application across non-medical domains and testing varied model architectures. Addressing limitations like dependency on accurate rationales and further refining multi-snippet evaluation will also be pivotal in advancing the framework's robustness.
By pushing the envelope of what is possible with AI in medicine, RAG2 represents not just a technical innovation but a meaningful step toward integrating AI into the fabric of healthcare and wellness. This groundbreaking approach opens up a new avenue for businesses in health technology to create more reliable, informative, and beneficial AI applications.
Subscribe to my newsletter
Read articles from Gabi Dobocan directly inside your inbox. Subscribe to the newsletter, and don't miss out.
Written by
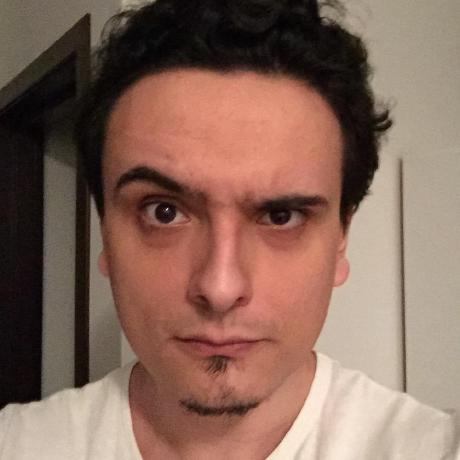
Gabi Dobocan
Gabi Dobocan
Coder, Founder, Builder. Angelpad & Techstars Alumnus. Forbes 30 Under 30.