Revolutionizing Image Matting: Zim's Zero-Shot Capabilities
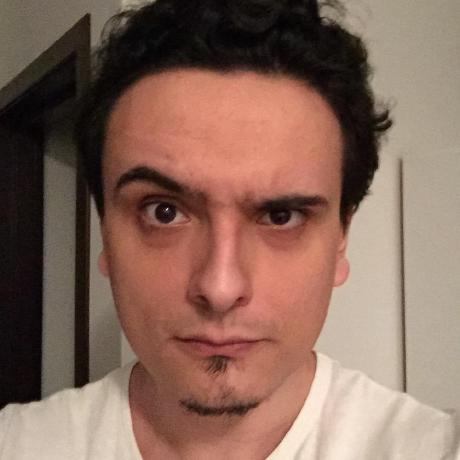

Zero-Shot Image Matting: Time for a New Perspective
In this article, we're taking a deep dive into "Zim: Zero-Shot Image Matting For Anything," a groundbreaking approach presented by a group of researchers from NAVER Cloud and various institutions. This work introduces ZIM, a model that extends the capabilities of the Segment Anything Model (SAM) by addressing its limitations in fine-grained precision and proposing a robust solution for zero-shot image matting. Let's explore how this innovation transforms image processing and its potential business applications.
- Arxiv: https://arxiv.org/abs/2411.00626v1
- PDF: https://arxiv.org/pdf/2411.00626v1.pdf
- Authors: Joonsang Yu, Dong-Hyun Hwang, Sewhan Chun, Se-Yun Lee, Hyungsik Jung, JoonHyun Jeong, Chanyong Shin, Beomyoung Kim
- Published: 2024-11-01
The Main Claims of the Paper
The paper primarily argues that while the Segment Anything Model (SAM) exhibits strong zero-shot segmentation capabilities, it falls short in generating precise and detailed matte masks. SAM's limitations become evident when it deals with the intricate boundaries needed for tasks like image inpainting or 3D reconstruction. To tackle this, the researchers propose ZIM, which can produce high-quality, fine-grained matting results while maintaining zero-shot capabilities.
ZIM's strength lies in its innovative approach to transforming segmentation labels into precise matte labels using a newly constructed dataset named SA1B-Matte. The model incorporates a hierarchical pixel decoder and a prompt-aware masked attention mechanism to enhance mask representation and precision. The new dataset, along with these architectural improvements, enables ZIM to outperform existing models in generating detailed matte masks.
Innovative Proposals and Enhancements
ZIM makes two major contributions:
Label Conversion Method: Instead of relying on expensive manual annotations to obtain detailed matting datasets, ZIM introduces a label converter that transforms existing segmentation labels into high-fidelity matte labels. This is achieved through methods like Spatial Generalization Augmentation and Selective Transformation Learning, which ensure the conversion yields minimal noise and maximum accuracy. These innovations culminate in the creation of the SA1B-Matte dataset, a large collection of micro-level detailed matte labels.
Improved Network Architecture: The researchers propose a hierarchical pixel decoder that enhances feature representation, reducing checkerboard artifacts typical in SAM's outputs. Additionally, the prompt-aware masked attention mechanism allows for dynamic focusing on user-specified regions, leading to better matting performance in interactive scenarios. Together, these enhancements make ZIM capable of generating more accurate and robust masks.
Leveraging ZIM for Business Opportunities
Businesses can leverage ZIM's advancements in several ways to boost revenue and optimize processes:
Photography and Media: Precision in image matting is crucial for creative endeavors involving complex image edits, such as background removal, portrait enhancement, and image manipulation. ZIM can streamline such tasks, saving time and reducing costs for companies in photography and digital content creation.
Augmented and Virtual Reality: ZIM's zero-shot matting capabilities can enhance AR and VR experiences by providing detailed environmental masks, improving object interaction and background integration in virtual spaces.
E-commerce and Marketing: ZIM enables quick and precise image matting for product showcases, facilitating better visual presentation without labor-intensive edits. This can significantly enhance online retail aesthetics and immersive marketing campaigns.
Medical Imaging: In medical fields, where precision is critical, ZIM can assist in segmenting anatomical structures from medical images, aiding diagnostics and research.
Film and Animation: The entertainment industry can utilize ZIM for special effects and post-production work, offering a new level of detail and efficiency without extensive manual efforts.
Training and Dataset Utilization
Training ZIM involves transforming the SA1B dataset's segmentation labels into matte labels using the innovative label converter. This process creates the extensive SA1B-Matte dataset. The model is then fine-tuned on this dataset using ViT-B as the backbone, leveraging SAM's pre-trained weights to ensure rapid model convergence and high matting accuracy.
Hardware Requirements
Running and training ZIM demands significant computational resources typical of modern AI models. It requires GPU capabilities at least similar to NVIDIA's V100 for efficient training and inference. Given the large-scale dataset and complex computations involved in running hierarchical decoders and attention mechanisms, robust GPU infrastructure is essential to deploy ZIM effectively.
Comparison with State-of-the-Art Alternatives
ZIM outperforms existing models like SAM, HQ-SAM, Matte-Any, Matting-Any, and SMat regarding mask precision for intricate, micro-level tasks. While these models offer strong segmentations, ZIM's enhanced architecture and dataset lead to more nuanced and accurate matting, addressing the shortcomings in detail fidelity apparent in alternatives.
Conclusions and Potential Improvements
The introduction of ZIM marks an advanced step in zero-shot matting. It effectively combines innovations in label conversion and architectural enhancements to achieve high-quality, intricate masks needed for various downstream applications. However, further work could make ZIM even more adaptable, such as broadening its dataset to include more contextual scenarios or optimizing its performance on computationally lighter infrastructures.
In conclusion, ZIM represents a promising leap in image matting, providing valuable insights and practical solutions across multiple domains. By harnessing ZIM's capabilities, industries can unlock new creative opportunities and operational efficiencies, bridging the gap between AI potential and real-world applications.
Subscribe to my newsletter
Read articles from Gabi Dobocan directly inside your inbox. Subscribe to the newsletter, and don't miss out.
Written by
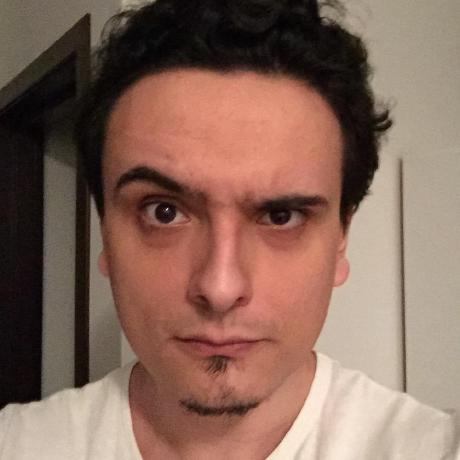
Gabi Dobocan
Gabi Dobocan
Coder, Founder, Builder. Angelpad & Techstars Alumnus. Forbes 30 Under 30.