Sliding Window Template and Important Pattern Problems

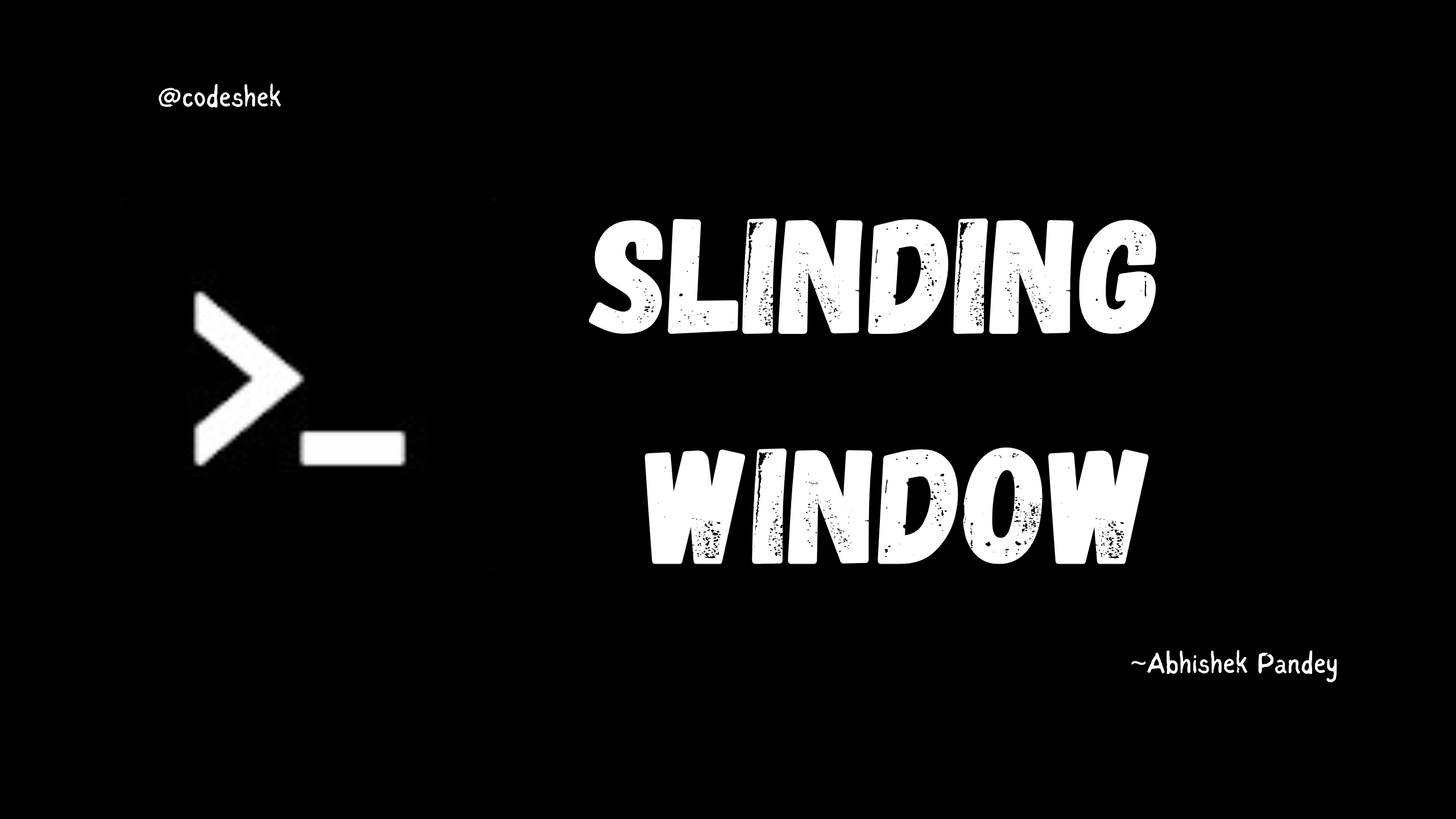
What is Sliding Window?
The sliding window is a commonly used technique in solving array and string problems. It involves creating a window (a range of indices) that slides over the data structure to analyze or compute specific properties, such as sums, counts, or substrings.
It is particularly effective for problems requiring a contiguous sequence of elements, as it avoids recalculating data redundantly, making it efficient with a time complexity of O(n) in many cases.
Types of Sliding Windows
Fixed-Size Sliding Window
A window with a fixed size that slides through the array or string.Used when the window size is predefined or constant.
Example: Maximum sum of subarrays of size k.
Dynamic-Size Sliding Window
A window whose size expands or shrinks based on conditions.Used when the window size depends on problem constraints.
Example: Longest substring without repeating characters.
Sliding Window Template Code
1. Fixed-Size Sliding Window
vector<int> fixedSlidingWindow(vector<int>& nums, int k) {
int n = nums.size();
vector<int> result; // Store results
int sum = 0; // Current window sum
for (int i = 0; i < n; i++) {
sum += nums[i]; // Add current element to the window
if (i >= k - 1) { // Check if window size is k
result.push_back(sum); // Store the result for this window
sum -= nums[i - (k - 1)]; // Remove the element going out of the window
}
}
return result;
}
2. Dynamic-Size Sliding Window
int dynamicSlidingWindow(string s, int target) {
int n = s.size();
int i = 0; // Left pointer of the window
int maxLength = 0; // Result for the maximum length
unordered_map<char, int> charCount; // Store character frequencies
for (int j = 0; j < n; j++) { // Right pointer of the window
charCount[s[j]]++; // Include the current character
// Shrink the window until the condition is satisfied
while (charCount.size() > target) {
charCount[s[i]]--;
if (charCount[s[i]] == 0) {
charCount.erase(s[i]);
}
i++; // Move the left pointer
}
maxLength = max(maxLength, j - i + 1); // Update the result
}
return maxLength;
}
Fixed-Size Sliding Window Problems
1. Easy
Maximum/Minimum Subarray Sum { >_ }
Find the maximum sum of any subarray of size
k
in a given array.Approach:
1️⃣ Use a sliding window to calculate the sum of the firstk
elements.
2️⃣ Slide the window one element at a time:Add the next element to the sum.
Subtract the element leaving the window.
3️⃣ Track the maximum sum during each iteration.
Complexity:
🕒 Time: O(n) (Each element is added and removed from the sum once).
🧠 Space: O(1) (No additional data structures are used).
class Solution { public: int maximumSumSubarray(vector<int>& arr, int k) { int n = arr.size(); int sum = 0; int store = 0; for(int i = 0; i < n; i++) { sum += arr[i]; // Add the current element to the window sum // Once the window size reaches k, subtract the element going out of the window if(i >= k - 1) { store = max(store, sum); // Update the maximum sum sum -= arr[i - (k - 1)]; // Remove the element that goes out of the window } } return store; } };
2. Medium
Find All Anagrams in a String { >_ }
(LC 438)
Given stringss
andp
, find all start indices ofp
's anagrams ins
.Approach:
1️⃣ Count character frequencies inp
using a frequency array.
2️⃣ Use a sliding window of sizep.length()
overs
.
3️⃣ For each window, decrement frequency for the added character and check if all frequencies are zero.
4️⃣ If zero, the current window is an anagram; store the start index.
5️⃣ Slide the window by restoring the frequency of the removed character.Complexity:
🕒 Time: O(n) (Sliding window processes each character once, and frequency check is O(1)).
🧠 Space: O(1) (Fixed frequency array of size 26).
class Solution { public: bool allzero(vector<int>& array) { for (int &i : array) { if (i != 0) { return false; } } return true; } vector<int> findAnagrams(string s, string p) { int n = s.size(); vector<int> array(26, 0); // Count the frequency of each char in pattern `p` for (char ch : p) { array[ch - 'a']++; } // Sliding window int i = 0, j = 0; vector<int> res; int k = p.length(); // Window size while (j < n) { array[s[j] - 'a']--; // Check if window size matches `p` if (j - i + 1 == k) { if (allzero(array)) { res.push_back(i); // Store the start index } array[s[i] - 'a']++; // Restore the frequency for sliding i++; // Slide the window } j++; } return res; }
Fruit Into Baskets { >_ }
(LC 904)
You’re given a list of fruits where you can pick from at most 2 types of trees in a row. The goal is to find the maximum number of fruits you can collect.Approach:
1️⃣ Use a sliding window with two pointers (i
andj
).
2️⃣ Expand the window by adding fruits to a map and track their count.
3️⃣ Shrink the window when the map size exceeds 2 (more than two fruit types).
4️⃣ Update the maximum length during each iteration.Complexity:
🕒 Time: O(n) (Each fruit is processed at most twice).
🧠 Space: O(1) (Map size limited to 2).
class Solution { public: int totalFruit(vector<int>& fruits) { int n = fruits.size(); int count = 0; unordered_map<int,int>mp; for(int i=0,j=0;j<n;j++){ mp[fruits[j]]++; while(mp.size()>2){ mp[fruits[i]]--; if(mp[fruits[i]]==0) mp.erase(fruits[i]); i++; } count = max(count, j-i+1); } return count; } };
Dynamic-Size Sliding Window Problems
1. Easy
Longest Substring with K Distinct Characters { >_ }
Given a string and an integer
k
, find the length of the longest substring with at mostk
distinct characters.Approach:
1️⃣ Use a sliding window and a hash map to count character frequencies.
2️⃣ Expand the window by adding characters until the distinct count exceedsk
.
3️⃣ Shrink the window by removing characters until the distinct count is back tok
.
4️⃣ Track the maximum length of valid substrings during each iteration.Complexity:
🕒 Time: O(n) (Each character is processed at most twice).
🧠 Space: O(k) (Hash map stores at most
k
characters).
#include <unordered_map>
#include <string>
#include <algorithm>
using namespace std;
int kDistinctChars(int k, string &str) {
int n = str.size();
unordered_map<char, int> charCount;
int i = 0, maxLength = 0;
for (int j = 0; j < n; j++) {
// Add the current character to the hash map
charCount[str[j]]++;
// Shrink the window until we have at most K distinct characters
while (charCount.size() > k) {
charCount[str[i]]--;
if (charCount[str[i]] == 0) {
charCount.erase(str[i]);
}
i++; // Move the start pointer
}
// Update the maximum length of the valid substring
maxLength = max(maxLength, j - i + 1);
}
return maxLength;
}
2. Medium
Subarray with Product Less Than K { >_ }
(LC 713)
Given an array of integersnums
and an integerk
, find the number of contiguous subarrays where the product of all elements is strictly less thank
.Approach:
1️⃣ Use a sliding window with two pointers (i
andj
).
2️⃣ Expand the window by multiplying the current element into the product.
3️⃣ If the product becomes ≥k
, shrink the window by dividing elements until it's <k
.
4️⃣ Count all subarrays within the window using the formulai - j + 1
.Complexity:
🕒 Time: O(n) (Each element is processed at most twice).
🧠 Space: O(1) (No additional data structures).
class Solution {
public:
int numSubarrayProductLessThanK(vector<int>& nums, int k) {
if(k<=1) return 0;
int n = nums.size();
int count =0;
int product = 1;
for(int i=0,j=0;i<n;i++){
product *= nums[i];
while(product >=k){
product /= nums[j++];
}
count += i-j+1;
}
return count;
}
};
3. Hard
Longest Repeating Characters Replacement { >_ }
Given a string
s
and an integerk
, find the length of the longest substring you can obtain by replacing at mostk
characters with any other character.Approach:
1️⃣ Use a sliding window with two pointers (i
andj
).
2️⃣ Track the frequency of characters in the window using a hash map.
3️⃣ Calculate the most frequent character in the current window.
4️⃣ If the window size minus the most frequent character count exceedsk
, shrink the window by movingi
.
5️⃣ Track the maximum window size that satisfies the condition.Complexity:
🕒 Time: O(n) (Each character is processed at most twice).
🧠 Space: O(1) (Hash map stores a fixed number of characters).
class Solution { public: int characterReplacement(string s, int k) { int n = s.size(); int i=0,j=0; int count =0; unordered_map<char,int>mp; int ans =-1; while(j<n){ mp[s[j]]++; count = max(count,mp[s[j]]); if((j-i+1) -count > k){ mp[s[i]]--; i++; } ans =max(ans,(j-i+1)); j++; } return ans; } };
Minimum Window Substring { >_ }
(LC 76)
Given stringss
andt
, find the minimum window ins
that contains all the characters oft
.Approach:
1️⃣ Count the frequency of each character int
using a hash map (dictT
).
2️⃣ Use two pointers (l
andr
) to create a sliding window.
3️⃣ Expand the window by movingr
and update the count of characters in the window.
4️⃣ When the window contains all characters fromt
, try to shrink it from the left by movingl
.
5️⃣ Track the minimum window size during each iteration.Complexity:
🕒 Time: O(n + m) (where
n
is the length ofs
andm
is the length oft
).🧠 Space: O(n) (for the hash maps to store character counts).
class Solution {
public:
string minWindow(string s, string t) {
if (s.empty() || t.empty()) {
return "";
}
unordered_map<char, int> dictT;
for (char c : t) {
int count = dictT[c];
dictT[c] = count + 1;
}
int required = dictT.size();
int l = 0, r = 0;
int formed = 0;
unordered_map<char, int> windowCounts;
int ans[3] = { -1, 0, 0 };
while (r < s.length()) {
char c = s[r];
int count = windowCounts[c];
windowCounts[c] = count + 1;
if (dictT.find(c) != dictT.end() && windowCounts[c] == dictT[c]) {
formed++;
}
while (l <= r && formed == required) {
c = s[l];
if (ans[0] == -1 || r - l + 1 < ans[0]) {
ans[0] = r - l + 1;
ans[1] = l;
ans[2] = r;
}
windowCounts[c]--;
if (dictT.find(c) != dictT.end() && windowCounts[c] < dictT[c]) {
formed--;
}
l++;
}
r++;
}
return ans[0] == -1 ? "" : s.substr(ans[1], ans[0]);
}
};
Subscribe to my newsletter
Read articles from Abhishek Pandey directly inside your inbox. Subscribe to the newsletter, and don't miss out.
Written by
