Breaking Down Real-Time Talking Portraits: AI's Leap Forward in Training Scenarios
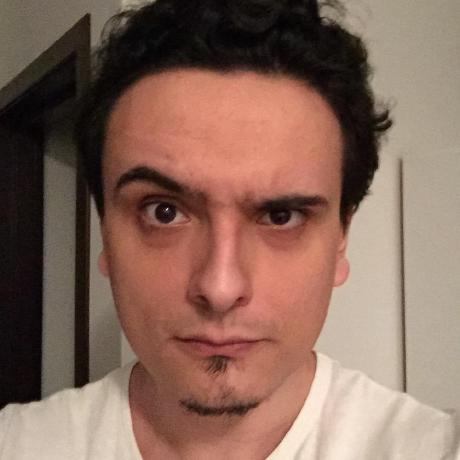
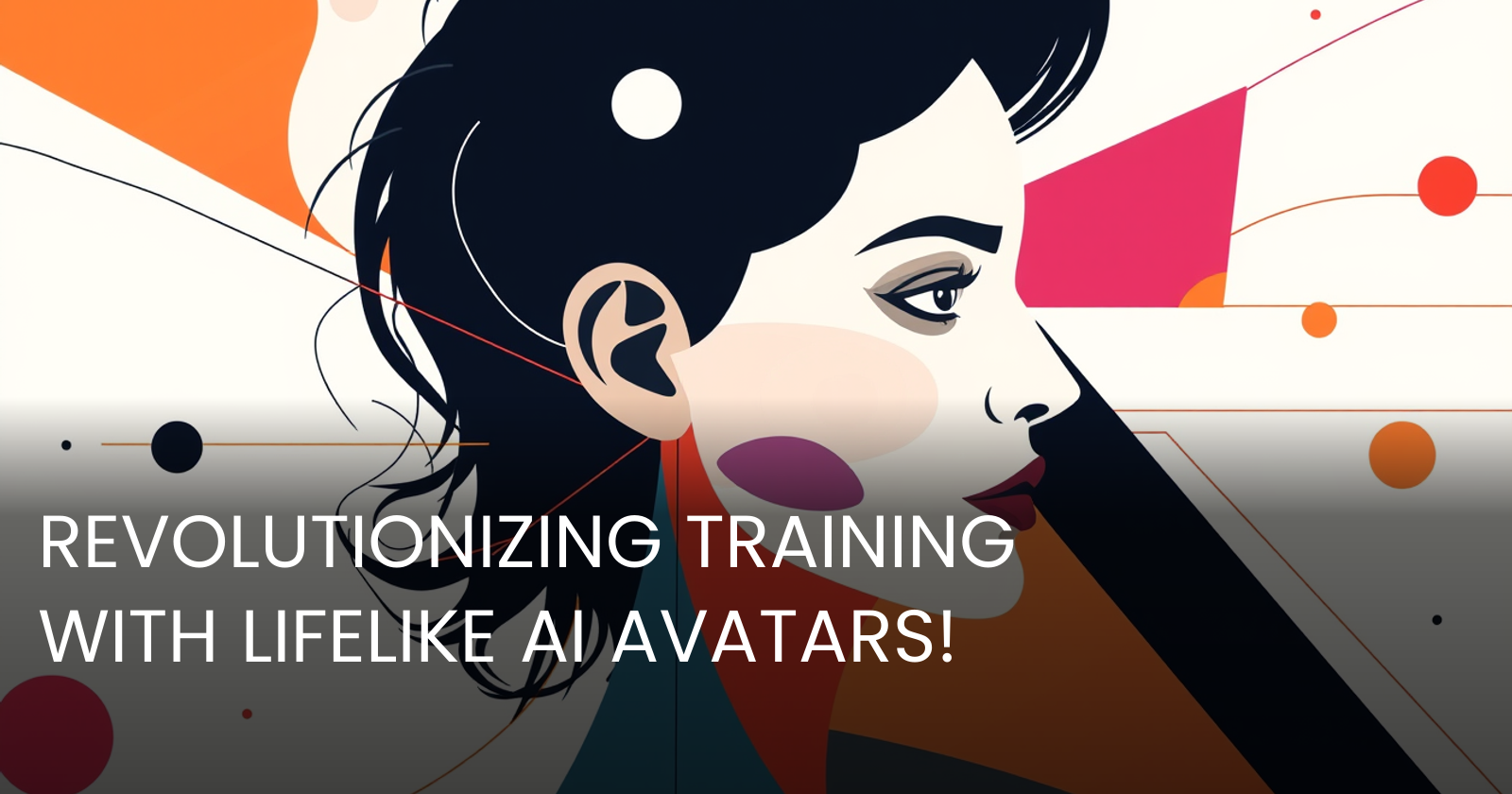
Introduction to Talking Portrait Synthesis
For businesses and organizations, technology representing virtual avatars has long promised revolutions in both customer interaction and training exercises. With the paper “Comparative Analysis Of Audio Feature Extraction For Real-Time Talking Portrait Synthesis,” a team of researchers proposes a remarkable enhancement in this realm by improving real-time talking-head generation — a breath of fresh air for applications like interviewer training, particularly within sensitive areas like Child Protective Services (CPS).
Through strategic system integrations and leveraging OpenAI's Whisper, the authors tackle longstanding challenges in Audio Feature Extraction (AFE), which often stalls progress due to latency issues, compromising the realism and immediate responsiveness of avatars. The system they propose doesn't just improve processing speed; it refines the process of synthesizing talking portraits to deliver truly lifelike and responsive virtual avatars.
- Arxiv: https://arxiv.org/abs/2411.13209v1
- PDF: https://arxiv.org/pdf/2411.13209v1.pdf
- Authors: Pål Halvorsen, Michael A. Riegler, Dag Johansen, Saeed S. Sabet, Sushant Gautam, Vajira Thambawita, Sajad Amouei Sheshkal, Pegah Salehi
- Published: 2024-11-20
Core Contributions
Main Claims
The paper makes bold claims about the efficiency and realism of a newly configured virtual avatar system tuned for interviewer training applications. By integrating OpenAI's Whisper model for AFE, the authors argue a significant reduction in processing delays, achieving smoother, more lifelike interactions. This responsiveness is crucial, especially for CPS interviewer training, where simulations demand ethical sensitivity and no room for lag or unrealistic interactions.
New Proposals
At its core, the paper proposes embedding Whisper's capabilities into the AFE pipeline — a drastic shift from traditional models like Deep-Speech, HuBERT, or Wav2Vec. Whisper, known for its speed and multilingual proficiency, adapts readily to process and synthesize audio inputs for real-time systems. This advancement hinges not only on audio efficiency but also on how video is rendered, coalescing human voice intonations with visual lip movements, something Whisper deftly handles.
Enhancements and Comparisons to Existing Models
Whisper outperforms other AFE models with greater synchronization accuracy and lip-sync quality, crucial for realistic avatars. It processes raw audio into log-Mel spectrograms with efficiency, achieving faster execution times than Deep-Speech or Wav2Vec models, especially with longer audio clips. This speed, synchrony, and visual quality raise the bar for interactive systems by minimizing latency, making it superior to current state-of-the-art alternatives.
Business Applications and Opportunities
Leveraging the System for Revenue Growth
By harnessing this advanced AFE model, businesses can innovate in areas such as:
- Interactive Training: Companies can employ realistic AI avatars for training scenarios in healthcare, education, and customer service industries. Particularly, sectors dealing with sensitive and ethical complexities, like CPS, can simulate scenarios that are as close to reality as possible.
- Enhanced Customer Engagement: Businesses can refine how they employ virtual assistants and chatbots, turning typical interactions into deeply personalized engagements that are quicker and more lifelike.
- Remote Education and Training Platforms: Institutions could utilize these enhanced talking portraits for more effective remote teaching, providing ‘face-to-face’ virtual interactions that mimic real human engagement.
New Product Ideas and Concepts Enabled
The improvements detailed in this paper open the door for several product innovations:
- Virtual Interviewer Avatars: Expand simulations for interviewer and psychology training where ethical and realistic scenarios are essential.
- Personalized AI Tutors: Develop educational tools using AI avatars to personalize learning experiences, adapting real-time to student questions and needs.
- Virtual Customer Agents: Deploy avatars that deal with customer queries and processes more fluidly, increasing efficiency in online service sectors.
Technical Requirements
Model Training and Datasets
In training their model, the researchers leveraged a vast array of multilingual and multitask data. The application of Whisper in this context allows for the synthesis of avatars that support diverse languages and dialects, broadening utility across global applications. The datasets primarily included high-definition speech video clips, maintaining a balance between synthetic and natural captures to ensure the model is robust across varied scenarios.
Hardware Needs
To harness the Whisper-based system, one would require a modern computing setup — a 12th Gen Intel Core i9 CPU, accompanied by an NVIDIA RTX 4090 GPU (24 GiB VRAM). This setup illustrates the necessity for cutting-edge graphics processing capabilities, pivotal for real-time, high-fidelity rendering.
Evaluating the Whisper Framework
Speed and Quality
The Whisper model accounts for significant gains in responsiveness and quality, boasting a near 80-90% reduction in processing times across various tasks compared to its peers. It also excels in synchronization, offering increased user engagement by aligning audio with visual lip movements seamlessly, helping to alleviate common uncanny valley issues.
Limitations and Areas for Improvement
Despite these advancements, there remain challenges with latency related to computationally demanding tasks like frame rendering. This area still comprises the bulk of system latency, despite Whisper’s advancements. Future research could explore optimizations in rendering, possibly through NVIDIA's Avatar Cloud Engine, to finely balance computational load and real-time performance further.
Conclusion and Future Enhancements
The integration of Whisper as an AFE solution for real-time talking-head systems promises a substantial leap in how avatars are utilized in training and customer engagement scenarios. By drastically decreasing latency while enhancing sound and motion synchronization, companies can pave the way for more lifelike interactions within digital spaces.
Looking forward, researchers and technologists should explore improving the back-end rendering processes and expand Whisper’s integration into broader real-world contexts. Besides, gathering professional feedback from actual field use will guide iterative improvements in functionality and realism.
In sum, this strategic synergy of AI tools presents a powerful avenue not only for enhanced commercial training simulations but also for customer-facing digital human interactions, heralding the next era in virtual avatar technology.
Subscribe to my newsletter
Read articles from Gabi Dobocan directly inside your inbox. Subscribe to the newsletter, and don't miss out.
Written by
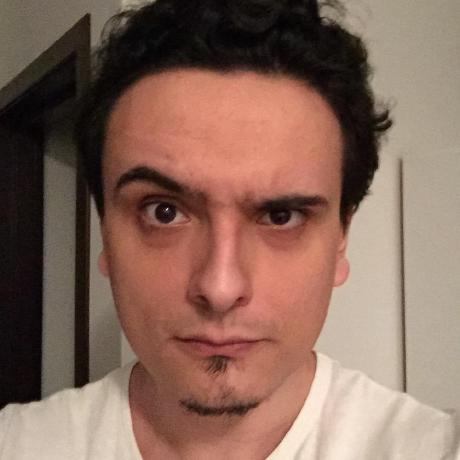
Gabi Dobocan
Gabi Dobocan
Coder, Founder, Builder. Angelpad & Techstars Alumnus. Forbes 30 Under 30.