Cross-Sentence Aspect Interactions for Sentiment Analysis in QA Forums
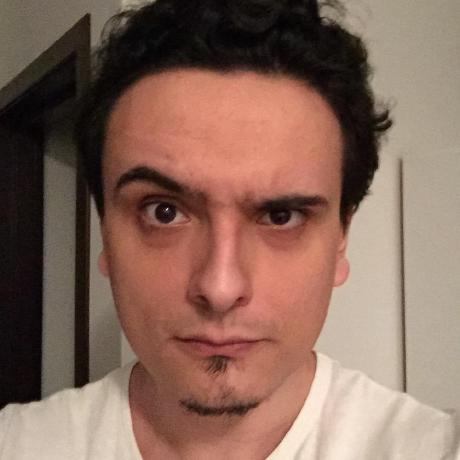

Understanding Aspect-Based Sentiment Analysis in QA Forums
The digital age has ushered in numerous platforms for information exchange, with Question Answering (QA) forums becoming a pivotal space for users to express opinions and seek advice on products. These forums harbor abundant insights woven into question-answer exchanges. A fascinating new study by Wenxuan Zhang and his team explores the frontier of Aspect-Based Sentiment Analysis (ABSA) within these QA forums, presenting new methodologies to help companies harness this rich data source.
- Arxiv: https://aclanthology.org/2021.findings-emnlp.390
- PDF: https://aclanthology.org/2021.findings-emnlp.390.pdf
- Authors: Wai Lam, Lidong Bing, Xin Li, Yang Deng, Wenxuan Zhang
- Published: null
Main Claims of the Study
The core assertion of the research posits that existing ABSA methods, primarily used for single sentence reviews or opinions, fall short when applied directly to QA forums. Such forums involve complex interactions between questions and their corresponding answers. The challenge is to extract aspects and sentiments when these elements may not be explicitly mentioned across the interconnected sentences of the QA pairs. Thus, this paper advances the field by proposing a specific model for effectively tackling the ABSA task within the context of QA forums, intricately handling cross-sentence aspect-opinion interactions.
New Proposals and Enhancements
The novel model introduced in this study incorporates cross-sentence attention mechanisms into the traditional ABSA framework. It emphasizes three main components:
Cross-Sentence Aspect Information Fusion: Leveraging inter-sentence attention, the model aligns the aspects in a question with opinions in the answer. This involves a sophisticated neural architecture grounded in BERT (Bidirectional Encoder Representations from Transformers) to manage contextual information across two separate but related text blocks.
Answer-Guided Sentiment Prediction: The model accentuates critical sentiment elements by encoding answer text to highlight pivotal opinion aspects, refining sentence representations.
QA Pair Matching Pre-Training: By pre-training the model on QA pair matching tasks, it equips the neural networks with the ability to recognize syntactic and semantic alignments between question and answer sentences, improving the capabilities in aspect-opinion linking.
Business Potential and Applicability
For enterprises, the ability to accurately interpret sentiment in QA forums presents multiple avenues to enhance customer engagement and business strategy:
Product Development: Real-time analysis of consumer sentiment can guide product modifications and the development of new features based on user feedback discerned from QA forums.
Customer Support Optimization: Companies can integrate this sentiment analysis into customer support systems to preemptively address negative feedback and improve user experience.
Market Analysis: The aggregate sentiment extracted from forums can inform market trends and competitor analysis, providing a robust basis for strategic planning.
Reputation Management: Monitoring sentiment polarity about different aspects of a brand allows proactive management of public perception.
Training the Model: Datasets and Methodologies
The research utilized datasets from the largest e-commerce platform in China, Taobao, specifically from electronics, beauty, and bags categories. The datasets comprised annotated QA pairs detailing various aspects discussed and their associated sentiment polarities.
The model harnessed BERT as the backbone for capturing contextual nuances between tokens in the text. It involved emphasis on critical sub-tasks like Aspect Term Extraction (ATE) and QA pair matching to train the model in a multi-task learning framework. This comprehensive training approach ensures the system comprehensively learns to associate aspects with sentiments across complex linguistic structures.
Hardware Requirements
The model’s training leveraging BERT, a heavyweight in natural language processing, necessitates significant computational resources. It was trained on a system equipped with a GeForce GTX 1080 Ti GPU. This requirement underscores the need for businesses looking to implement similar models to invest in substantial hardware capabilities or consider cloud-based resources to handle the processing load.
Comparative Analysis with State-of-the-Art (SOTA) Models
In benchmarking the proposed model against existing SOTA models, it revealed superior performance by handling the cross-sentence dynamics intrinsic to QA pairs, typically overlooked by conventional ABSA models designed for single sentence reviews. The improved F1 scores across test dataset evaluations underscore the model’s efficacy in nuanced sentiment detection.
Conclusions and Areas for Improvement
This study drives home the point that understanding consumer sentiment in QA forums is complex yet revealing, offering crucial business insights. The proposed model advances the frontier by faithfully capturing the interplay of aspects and sentiments across QA sentence pairs, boasting an enhanced capacity over traditional singular ABSA methods. However, future work could look into lightening the computational load, potentially improving accessibility for wider business application.
In conclusion, this research outlines a potent tool for businesses. By enabling nuanced sentiment analysis in QA forums, companies can tap into the authentic voice of their consumers, using these insights to drive strategic business decisions. As machine learning continues to evolve, embracing these innovations will be key for companies keen on staying ahead in an increasingly opinion-shared digital economy.
Subscribe to my newsletter
Read articles from Gabi Dobocan directly inside your inbox. Subscribe to the newsletter, and don't miss out.
Written by
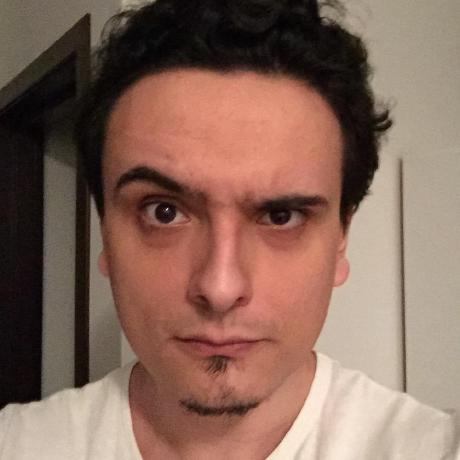
Gabi Dobocan
Gabi Dobocan
Coder, Founder, Builder. Angelpad & Techstars Alumnus. Forbes 30 Under 30.