Understanding Predictive Processing: How the Brain's Perception Saves Energy and Influences Engineering

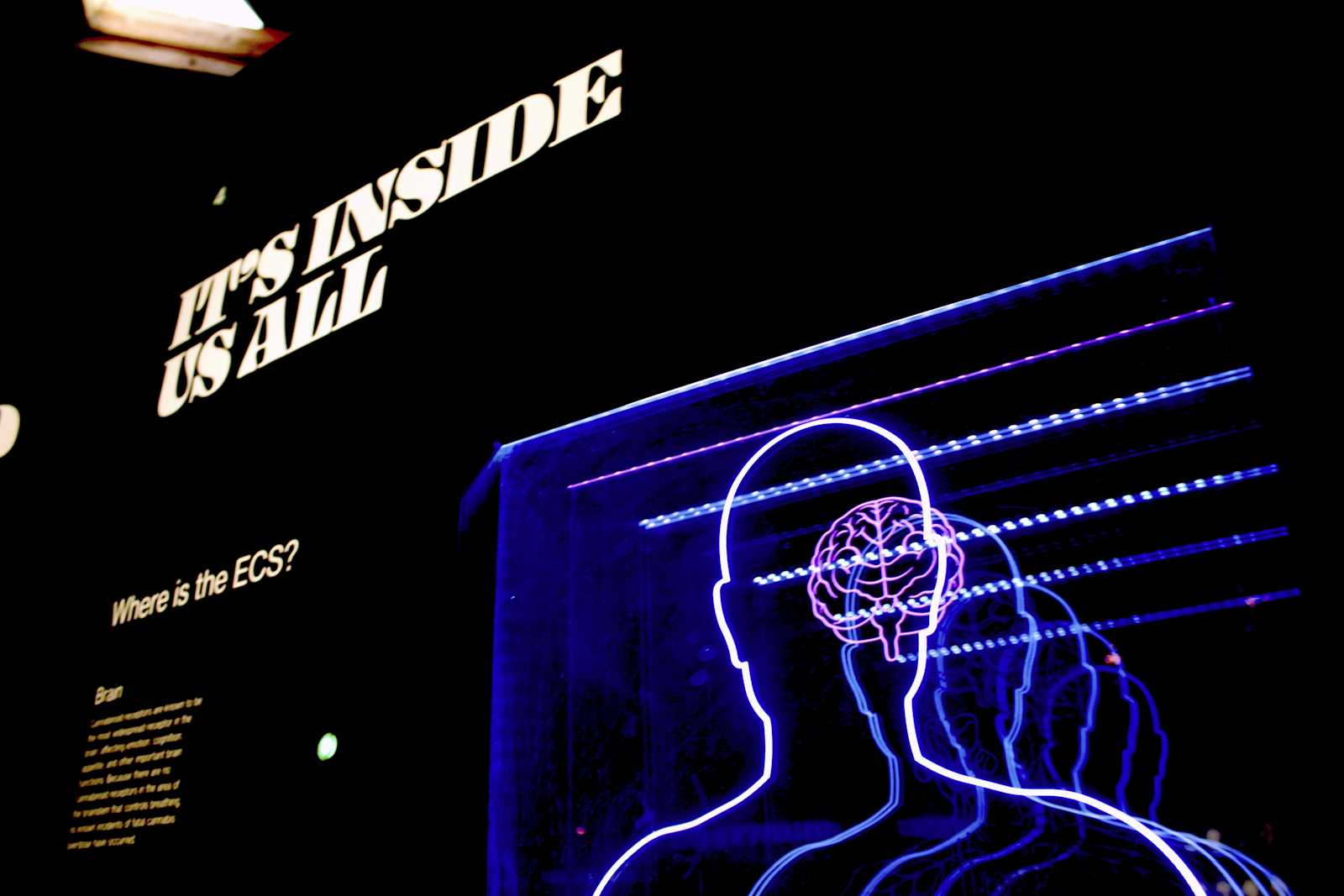
This is a summary of the bog post: To Be Energy-Efficient, Brains Predict Their Perceptions**. Here, I select the main points of the blog post and also discuss what this could mean for you.**
Predictive Processing
Predictive processing, or unconscious inferences in perception, is used in the brain's processing of stimuli.
The brain uses its prior knowledge to make inferences about incoming sensory information. These inferences, not the incoming sensory information, give rise to perception in our minds.
The more scattered the incoming information, the greater the reliance on predictive processing.
How then does the brain implement predictive processing?
One popular model used to understand how the brain implements predictive processing is predictive coding (which utilizes the top-down model of perception).
In predictive coding, the brain has two populations of neurons dedicated to different tasks. One set encodes the best prediction and the second set signals prediction errors.
In the overall analysis of the brain, the “Recurrent Neural Network”, RNN is used. In this model, the neurons have both feedforward and feedback connections, which are independent of whatever sensory input is received.
Researchers created an artificial network based on RNN that learned to predict the next frame in a video sequence. It was called PredNet, and it demonstrated the behavior seen in monkey brains. Researching how artificial neurons in PredNet conserve energy would aid in comprehending how the brain conserves energy.
After the study, it was discovered that the brain conserves energy by minimizing synaptic transmission. For synaptic transmission to be minimized, predictive processing had to be utilized.
You can see Predictive processing is a way to provide a unifying framework for brain behaviors, to explain many phenomena in one go, rather than having different models to explain different phenomena in the brain.
Practical Applications in Daily Life
Improved Learning: Understanding that your brain relies on prior knowledge to make sense of new information can enhance learning strategies. For example:
Teachers and trainers can build lessons on learners’ existing knowledge to make new concepts easier to grasp.
Students can focus on creating meaningful connections between new material and what they already know.
Mindfulness Practices: Predictive processing shows how expectations shape perceptions. Practicing mindfulness can help you become more aware of biases and focus on the present. This would help reduce the influence of incorrect predictions.
Communication and Relationships: Realizing that everyone’s brain interprets situations based on their prior experiences can improve empathy and communication. Being patient and clarifying intent helps bridge gaps in perception.
Technology and Innovation: Research on predictive models like PredNet can lead to advancements in AI and machine learning, creating systems that mimic human cognition and improve efficiency in applications like autonomous vehicles and video prediction.
Can Predictive Processing Be Stopped?
Predictive processing is a fundamental function of the brain and cannot be completely stopped. It is integral to how we process sensory information and interact with the world. However, its influence can be moderated:
Mediation, Mindfulness, and Awareness: Practices like meditation can help reduce automatic assumptions and increase awareness of sensory input.
Always do or learn something new: Engaging in new activities or environments forces the brain to rely less on prior knowledge and more on real-time information.
Is Predictive Processing More Pronounced in Some Individuals?
Predictive processing can vary among individuals based on:
Experience and Knowledge: People with more knowledge or experience in a field may rely more heavily on predictive processing in that domain.
Neurodiversity: Conditions like autism, ADHD, or schizophrenia can alter how predictive processing operates, leading to heightened sensitivity to prediction errors or a stronger reliance on sensory input.
Stress and Fatigue: In stressful or fatigued states, the brain may rely more on predictions to conserve energy, leading to quicker but potentially less accurate perceptions.
Applications of Predictive Processing in Engineering
Energy-Efficient Systems:
Predictive processing is fundamentally about conserving energy by minimizing unnecessary computation or synaptic transmission. Engineers can draw inspiration from this to design energy-efficient systems, such as:Power grids that predict demand and allocate resources dynamically to reduce energy wastage.
AI and machine learning models that prioritize predictive coding to lower computational overhead.
Autonomous Systems:
Autonomous vehicles and robots can use predictive models to anticipate environmental changes, obstacles, and human behavior, enabling faster and more accurate responses.- For example, collision avoidance systems can predict potential hazards based on previous patterns and adjust in real time.
Fault Detection and Maintenance:
Predictive processing principles can enhance predictive maintenance in engineering systems.By analyzing historical performance data, engineers can identify deviations (prediction errors) that indicate wear and tear, preventing system failures.
Examples include monitoring turbines, engines, and electrical systems for early signs of failure.
Signal Processing:
Just as the brain filters and prioritizes sensory input, engineers can develop signal-processing systems that emphasize relevant data while reducing noise.- Applications include medical imaging, telecommunications, and audio processing systems.
Human-Machine Interaction:
Predictive processing can improve interfaces between humans and machines.- For instance, adaptive interfaces in augmented reality (AR) and virtual reality (VR) systems can predict user intent and adjust displays or controls accordingly, enhancing usability.
Neural-Inspired AI:
Engineers can design neural networks and artificial intelligence systems that mimic predictive coding mechanisms, such as Recurrent Neural Networks (RNNs) and models like PredNet.- These systems are particularly useful in video compression, forecasting, and dynamic optimization problems.
Inspiration for Design Principles
Redundancy Reduction:
Systems can be designed to minimize redundant data processing, much like how the brain minimizes synaptic transmission.Feedback Loops:
Incorporating feedforward and feedback mechanisms ensures systems adapt dynamically to errors, similar to recurrent neural networks.
Examples in Real-World Engineering
Energy Management in Buildings: Predictive algorithms can regulate heating, ventilation, and air conditioning (HVAC) systems based on weather forecasts and usage patterns.
Predictive Traffic Systems: Using prior traffic data to forecast congestion and optimize traffic flow.
Conclusion
Predictive processing is essential for brain function, and by understanding it, we can enhance learning, communication, and innovation, while mindful practices can help us manage its impact for greater adaptability. it also has applications in engineering.
Reference
Ananthaswamy, A., 2021. To Be Energy-Efficient, Brains Predict Their Perceptions. Quanta Magazine. Available at: https://www.quantamagazine.org/to-be-energy-efficient-brains-predict-their-perceptions-20211115/ [Accessed 2 January 2025].
Subscribe to my newsletter
Read articles from Praise Nnamonu directly inside your inbox. Subscribe to the newsletter, and don't miss out.
Written by

Praise Nnamonu
Praise Nnamonu
I have an intense desire to make positive changes in Nigeria using engineering technology and to stand out. In the past, I have interned as a Mechanical Engineer at Innoson Vehicle Manufacturing and Nigerian Breweries. I have also interned as a Software Engineer at Code Day Labs and was a Teaching Assistant at the Kibo School of Technology. I have also volunteered for different roles in my association. My top strengths are gratitude, Hope, and Zest (courage), I don't do anything halfway. I have developed hard skills like Design, Programming, and maintenance, and along with those are effective communication, engineering for development, optimal learning, and also being able to follow. My experiences have taught me the importance of team building, problem-solving, and effective communication and have strengthened my commitment to leadership development. I am excited about the role technology is playing in shaping the country. I believe it is a future that we cannot escape and I want to be part of those leading the charge and making positive changes with technology in the country.