Building a ChatOps AI Bot with LangChain and LLMs in Slack

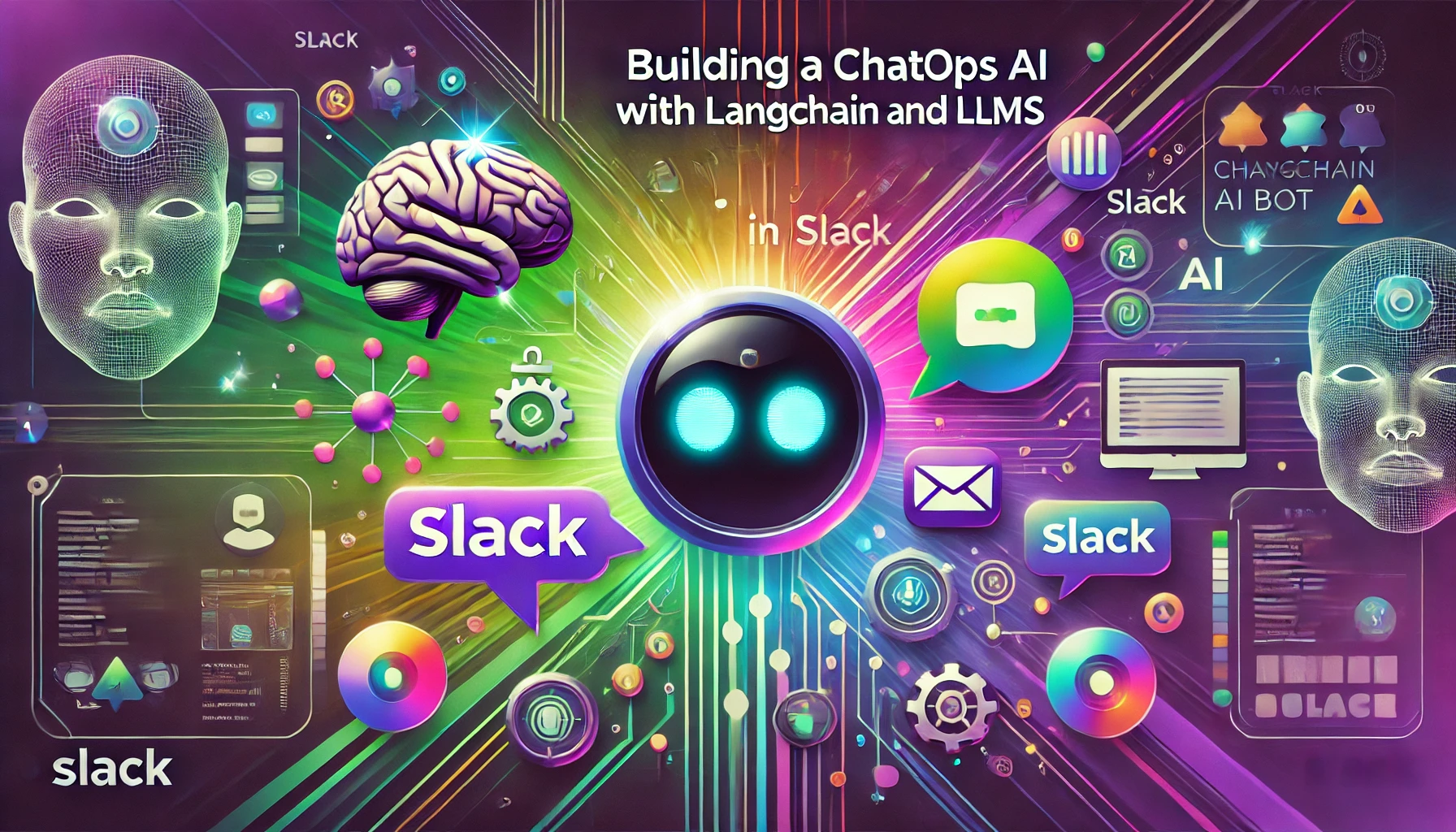
Intro
When it comes to managing operations, wouldn't it be great to have a more intuitive, convenient way to execute tasks, delegate work, and empower teams for self-service? That's what ChatOps brings to the table.
It's not just a trend—it's a paradigm shift in how we interact with our systems. While bots themselves aren't new, we're about to revolutionize how we interact with them, making the experience smarter, more intuitive, and deeply conversational.
Today, I'm going to walk you through how I built a ChatOps bot using Slack, LangChain, and an LLM of my choice, all wrapped in Python.
Why ChatOps?
ChatOps is all about making operations more accessible and collaborative. By integrating operations directly into Slack (or any chat platform), it becomes:
Intuitive: Users interact in natural language rather than remembering complex commands.
Convenient: Operations happen where your team is already communicating.
Empowering: Enables delegation and self-service, minimizing reliance on admins for every minor operation.
Automated: Offloads repetitive tasks, leaving your team free to focus on higher-level decisions.
ChatOps with LLM
Traditionally, ChatOps bots like ErrBot work by triggering commands—users invoke specific commands directly. For example, typing !status
would return the status of a service.
But what if we could make it smarter and more conversational? Instead of explicitly typing exact commands, we can leverage LLM to make our bot understand and execute intent, even if the phrasing isn't an exact match. This is where LangChain comes in.
LangChain
LangChain is a powerful framework that simplifies the integration of LLMs (large language models) into real-world applications. It manages components like querying the LLM, parsing responses, and chaining together operations to make it seamless for developers like us. LangChain enables us to easily adapt traditional bots like ErrBot, injecting them with the intelligence of an LLM—while still keeping operations under tight control.
Defining My ChatOps Framework
For any good operational framework, a few essentials are non-negotiable:
RBAC (Role-Based Access Control): Define who can trigger which commands and limit the actions they can perform.
Channel Control: Specify where the bot can operate—not all commands should work in every channel for safety reasons.
Command Rules: Decide what tasks the bot can execute.
In my case, I’m using ErrBot as the underlying framework for simplicity and layering LangChain on top of it to add conversational intelligence.
Features My Bot Supports
Before introducing LangChain to the mix, my bot already supported the following commands:
help: Lists all the available commands.
status: Returns the current status of a service or environment.
approve_release: fetches the pending releases and prompts for approval
These commands work perfectly, but they lack the flexibility of natural language interactions. For example:
- Instead of typing
!status
, wouldn’t it be nice to type “What’s the system status?” and have the bot figure out the intent?
Architecture: The Big Idea
Here’s how I designed the bot after integrating LangChain:
Intent Classifier
The heart of the system! It takes the user’s prompt, runs it through an LLM (via LangChain), and extracts the intent. For example:Input: “Can you check on the system?”
Result: Intent is classified as
status
.
The intent classifier strips down the natural language input into actionable commands for ErrBot. This ensures that only valid intents trigger actions, reducing the risk of LLM hallucinations or misfires. It's contextual and scoped, making it both powerful and safe.
LLM Integration with JSON Responses
The LangChain model responds with structured JSON, making it easy to decode:{ "intent": "status", "parameters": {} }
By relying on this structured response, we can map intents to specific bot commands confidently.
Mapping Intent to Commands
Once the intent is identified, the bot triggers the appropriate command handler. It’s a simple and modular pipeline that feels scalable and robust: - User types natural language. - LLM determines intent. - The bot executes the mapped command.
Why This Approach Works
This architecture minimizes AI hallucination by restricting the context in which the LLM operates. The intent classifier ensures the bot doesn’t go rogue—it only triggers predefined, purposeful commands. This guards your ChatOps setup and makes AI-powered automation more predictable.
Code Example
Here’s a simplified Python example showing how this would work using LangChain and Slack as the frontend:
from typing import Optional, Dict, Any
from langchain_openai import OpenAI
from langchain.prompts import PromptTemplate
from langchain.schema.runnable import RunnablePassthrough
import json
import logging
logger = logging.getLogger(__name__)
class IntentClassifier:
def __init__(self, api_key: str, api_base: str, config: dict):
"""Initialize the intent classifier with LangChain"""
self.llm = OpenAI(
model="claude-3-5-sonnet-latest",
temperature=0.3, # Lower temperature for more consistent results
openai_api_key=api_key,
openai_api_base=api_base
)
# Get commands from config
self.commands = config.get("bot", {}).get("commands", {})
# Build intent descriptions from commands
intent_descriptions = []
for cmd_name, cmd_config in self.commands.items():
if cmd_config.get("enabled", True):
description = cmd_config.get("description", "")
aliases = cmd_config.get("aliases", [])
alias_text = f" (aliases: {', '.join(aliases)})" if aliases else ""
intent_descriptions.append(f"- {cmd_name}: {description}{alias_text}")
logger.debug(f"Loaded {len(intent_descriptions)} commands for intent matching")
# Define the classification prompt template
template = """
You are a Slack bot designed to classify user messages into predefined intents.
Your task is to analyze the user message and match it to the closest predefined intent or respond if no match is found.
Predefined intents include:
{commands}
User message: {message}
Return a JSON object with:
- intent: The matched intent name or null if no match
- confidence: Confidence score between 0 and 1
- extracted_params: Any parameters extracted from the message (optional)
Important rules:
1. Only return intents from the predefined list above
2. Consider command aliases when matching intents
3. Extract any relevant parameters mentioned in the message
4. Return null if no intent matches with high confidence (below 0.6)
5. Ensure the response is valid JSON with no additional text
6. Be generous with confidence scores when the intent is clear
"""
self.prompt = PromptTemplate(
input_variables=["message", "commands"],
template=template
)
self.commands_str = "\n".join(intent_descriptions)
# Create a runnable chain
self.chain = (
{"message": RunnablePassthrough(), "commands": lambda _: self.commands_str}
| self.prompt
| self.llm
)
logger.info("Intent classifier initialized successfully")
async def classify_message(self, message: str) -> Optional[Dict[str, Any]]:
"""Classify a message and return the intent details"""
try:
logger.debug(f"Classifying message: {message}")
# Run the classification chain
result = await self.chain.ainvoke(message)
logger.debug(f"Raw classification result: {result}")
# Parse the JSON response
intent_data = json.loads(result)
# Validate the response format
if not isinstance(intent_data, dict):
logger.error(f"Invalid classification response format: {result}")
return None
required_fields = ["intent", "confidence"]
if not all(field in intent_data for field in required_fields):
logger.error(f"Missing required fields in classification: {result}")
return None
# Log the classification result
intent = intent_data.get("intent")
confidence = intent_data.get("confidence", 0)
params = intent_data.get("extracted_params", {})
logger.info(
f"Classification result - Intent: {intent}, "
f"Confidence: {confidence}, Params: {params}"
)
return intent_data
except Exception as e:
logger.error(f"Error classifying message: {str(e)}")
return None
def _validate_confidence(self, confidence: float) -> bool:
"""Validate confidence score is between 0 and 1"""
return isinstance(confidence, (int, float)) and 0 <= confidence <= 1
In this example:
The LLM determines intent based on my input.
Based on the intent (
status
,echo
), the bot routes the input to the relevant handler.Only predefined commands are triggered, keeping the system secure and deterministic.
Thank you !!!
Subscribe to my newsletter
Read articles from Jothimani Radhakrishnan directly inside your inbox. Subscribe to the newsletter, and don't miss out.
Written by

Jothimani Radhakrishnan
Jothimani Radhakrishnan
A Software Product Engineer, Cloud enthusiast | Blogger | DevOps | SRE | Python Developer. I usually automate my day-to-day stuff and Blog my experience on challenging items.