ETL vs ELT: Key Differences, Evolution, and Choosing the Right Data Integration Approach
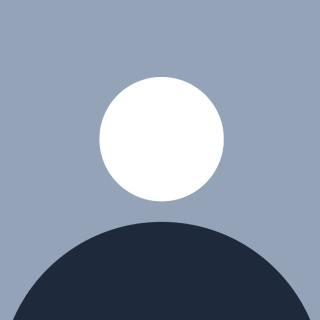
In the world of data integration, two main approaches have emerged as dominant architectural patterns: ETL vs ELT. ETL (Extract-Transform-Load) represents the traditional method where data undergoes transformation before reaching its final destination, while ELT (Extract-Load-Transform) takes a more modern approach by transforming data after it arrives at the target system. Both methodologies serve distinct purposes in data processing pipelines, each with their own advantages and trade-offs. Understanding these differences is crucial for organizations looking to build efficient, scalable data integration solutions that meet their specific business needs.
Core Differences Between ETL and ELT Architectures
ETL: The Traditional Approach
ETL architecture operates by processing data through a dedicated transformation engine before it reaches its destination. This approach leverages specialized processing frameworks like Apache Spark or Hadoop, where developers can implement complex transformations using various programming languages or SQL. The key advantage lies in its ability to handle intricate data manipulations while maintaining complete control over the transformation process.
ELT: The Modern Alternative
ELT takes a different path by loading raw data directly into the target system, where transformations occur afterward. This method capitalizes on the powerful processing capabilities of modern data warehouses and lakehouses. By postponing transformations until after data loading, organizations gain flexibility in how they structure and analyze their data.
Schema Management and Flexibility
ETL systems excel in environments with stable, well-defined schemas where data structures rarely change. This makes them ideal for traditional enterprise applications with consistent reporting requirements. However, they can become cumbersome when dealing with frequent schema changes or evolving business needs.
In contrast, ELT systems offer superior flexibility when handling dynamic schemas. Since raw data is loaded first, analysts and engineers can modify transformation logic without rebuilding the entire pipeline. This adaptability makes ELT particularly valuable for organizations with rapidly changing analytical requirements or those working with varied data sources.
Processing Capabilities and Cost Considerations
ETL provides granular control over transformation processes, allowing organizations to optimize processing costs through careful planning and resource allocation. Teams can aggregate similar transformations and implement efficient processing strategies. However, maintaining separate processing engines requires additional infrastructure and expertise.
ELT shifts the processing burden to the destination platform, potentially leading to higher costs as transformations are executed on the full dataset within the warehouse. While this simplifies infrastructure management, organizations must carefully monitor their warehouse usage to prevent unexpected cost escalations, especially when dealing with large data volumes or complex transformations.
Evolution and Historical Context of Data Integration Methods
Origins of Data Processing Patterns
The development of data integration methods has followed a circular path. Early database systems actually employed ELT-like processes, utilizing built-in SQL engines for data transformation. Organizations would bulk load data into databases and perform transformations using native database capabilities. This approach served well when data volumes were manageable and processing requirements were relatively simple.
Rise of Distributed Processing
As data volumes grew exponentially in the early 2000s, traditional databases struggled to handle massive transformation workloads. This limitation gave rise to distributed processing frameworks like Hadoop and later Apache Spark. These technologies enabled the ETL pattern to become dominant, offering unprecedented processing power and the ability to handle complex transformations outside the database environment.
Modern Data Warehouse Capabilities
The landscape shifted again with the emergence of cloud-based data warehouses and modern lakehouse platforms. These systems combine massive storage capabilities with powerful distributed SQL engines, effectively reviving the ELT approach. Modern platforms like Snowflake, Amazon Redshift, and Google BigQuery can process enormous datasets efficiently, making in-database transformations viable once again.
Current Technology Landscape
Today's data integration landscape features a mix of both approaches. Cloud platforms have revolutionized data storage and processing capabilities, making ELT more attractive for many use cases. These platforms offer elastic computing resources, allowing organizations to scale processing power on demand. Meanwhile, ETL remains relevant for scenarios requiring specialized transformations or strict data governance.
Framework Integration
Modern data platforms often bridge the gap between ETL and ELT by offering hybrid capabilities. Tools like dbt (data build tool) provide software engineering practices for ELT workflows, while traditional ETL tools have evolved to support cloud-native operations. This convergence has created a more flexible environment where organizations can choose the most appropriate approach for their specific needs, or even combine both methods within their data architecture.
Critical Decision Factors for Choosing Between ETL and ELT
Data Structure Requirements
Organizations with fixed schema requirements and standardized reporting typically benefit from ETL's structured approach. This method excels when data transformations follow consistent patterns and business rules remain stable. Development teams can implement robust error handling, validation checks, and quality controls within the transformation layer, ensuring data reliability before it reaches the destination system.
Engineering Complexity
ETL implementations demand higher technical expertise and specialized knowledge of processing frameworks. Teams must maintain separate transformation environments and manage complex data pipelines. However, this complexity brings benefits in code organization, version control, and testing capabilities. Modern platforms like Dataforge help minimize these challenges by providing abstraction layers over complex processing frameworks.
Flexibility and Agile Analytics
ELT shines in environments requiring rapid experimentation and frequent requirement changes. Analytics teams can quickly iterate on transformation logic without waiting for pipeline modifications. This approach particularly suits organizations adopting agile methodologies or those exploring new data insights. The ability to transform data on-demand enables faster decision-making and more dynamic analysis capabilities.
Resource Management
Storage and processing costs differ significantly between approaches. ELT requires more storage capacity as it maintains complete raw data copies in the destination system. However, it may reduce operational complexity by eliminating separate transformation infrastructure. ETL often provides better cost control for transformation processing but requires additional infrastructure management and maintenance resources.
Governance and Compliance
Data governance requirements heavily influence the choice between ETL and ELT. ETL provides stronger controls for sensitive data handling, allowing organizations to implement precise masking, filtering, and access controls before data reaches the destination. ELT shifts these responsibilities to the warehouse platform, potentially limiting governance options to available platform features. Organizations handling sensitive information or operating under strict regulatory requirements must carefully evaluate these implications.
Conclusion
Choosing between ETL and ELT architectures requires careful evaluation of organizational needs, technical capabilities, and business objectives. Neither approach stands clearly superior to the other - each serves distinct purposes in modern data integration strategies. ETL remains valuable for organizations requiring strict data governance, complex transformations, and established processing workflows. Its mature ecosystem and robust tooling support enterprise-grade data processing requirements and standardized reporting needs.
ELT offers compelling advantages for organizations prioritizing agility and rapid analytics development. Its flexibility in handling evolving requirements and unstructured data makes it particularly suitable for modern cloud-native architectures. The reduced complexity in initial setup and maintenance can accelerate time-to-insight for data teams.
Many organizations find success in implementing hybrid approaches, leveraging both architectures where they make the most sense. For example, using ETL for sensitive data processing and compliance requirements while employing ELT for exploratory analytics and rapid prototyping. As data platforms continue to evolve, the distinction between these approaches may blur further, offering even more flexible and powerful options for data integration. The key lies in understanding these patterns' strengths and limitations to make informed decisions that align with specific use cases and business requirements.
Subscribe to my newsletter
Read articles from Mikuz directly inside your inbox. Subscribe to the newsletter, and don't miss out.
Written by