Stable Diffusion Explained
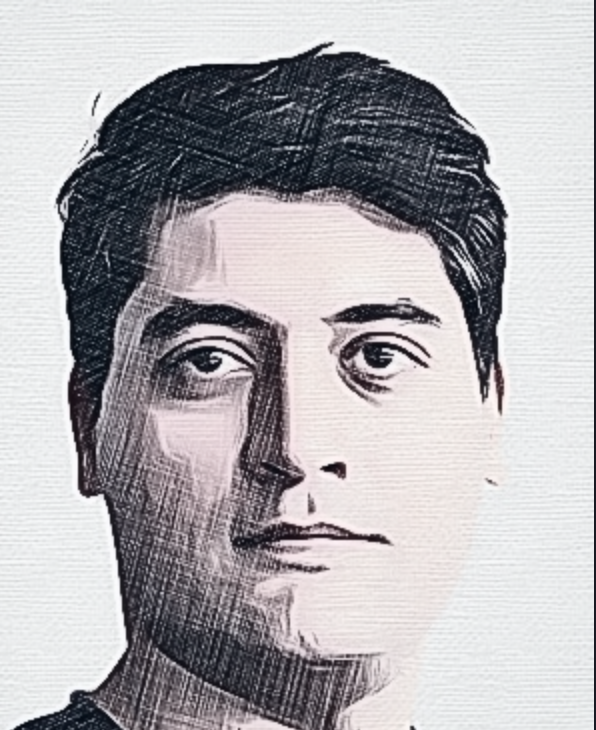
Stable Diffusion is a latent diffusion model (LDM) that generates images from text prompts using a process of iterative denoising. It operates in a compressed latent space rather than pixel space, making it more efficient while maintaining high-quality image synthesis. The model is based on deep learning techniques, particularly variational autoencoders (VAEs), U-Net architectures, and text encoders, typically using CLIP (Contrastive Language–Image Pretraining) to interpret textual descriptions.
The core principle of diffusion models is to gradually remove noise from an initially random input. Stable Diffusion first encodes an image into a lower-dimensional latent space using a VAE, reducing computational complexity. Then, a U-Net, conditioned on a text prompt processed by a CLIP text encoder, learns to predict and remove noise step by step. This denoising process is governed by a stochastic differential equation, which enables the generation of coherent and visually meaningful images from pure noise.
A key innovation in Stable Diffusion is its ability to operate in the latent space instead of the pixel space, making it significantly more efficient than earlier diffusion models like DALL·E 2 or Imagen. By applying denoising steps in a compressed latent representation, the model reduces the computational cost while preserving image fidelity. This allows it to run on consumer GPUs with as little as 4GB of VRAM, making high-quality image generation accessible to a wider audience.
Text conditioning in Stable Diffusion is achieved using a cross-attention mechanism that aligns textual features with visual representations. The CLIP text encoder translates text into a latent representation that guides the image generation process. This enables users to create highly specific outputs by modifying prompts with keywords, weights, or negative prompts to influence style, composition, and details. The cross-attention layers in the U-Net allow the model to dynamically adjust image features based on the input prompt.
it has numerous applications, including digital art, concept design, and image inpainting. Additionally, its open-source nature allows developers to fine-tune the model for specific tasks, such as generating medical images or architectural designs. Despite its advantages, the model also raises ethical concerns, such as bias in training data and potential misuse for deepfake generation. Researchers continue to explore ways to improve safety, controllability, and fairness in AI-generated content.
You can learn more about Stable Diffusion here: https://sdtools.org/
P.S: Took a while :-)
Subscribe to my newsletter
Read articles from Mahdi M. directly inside your inbox. Subscribe to the newsletter, and don't miss out.
Written by
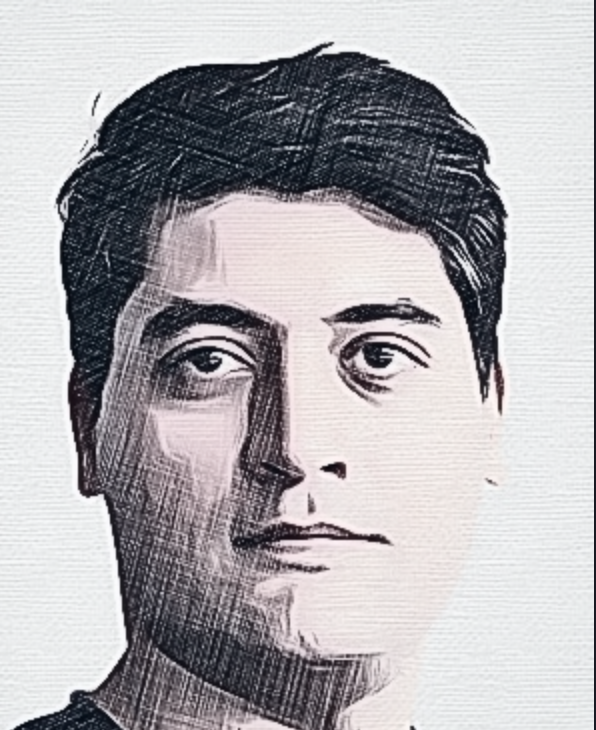