Random Forest Regression

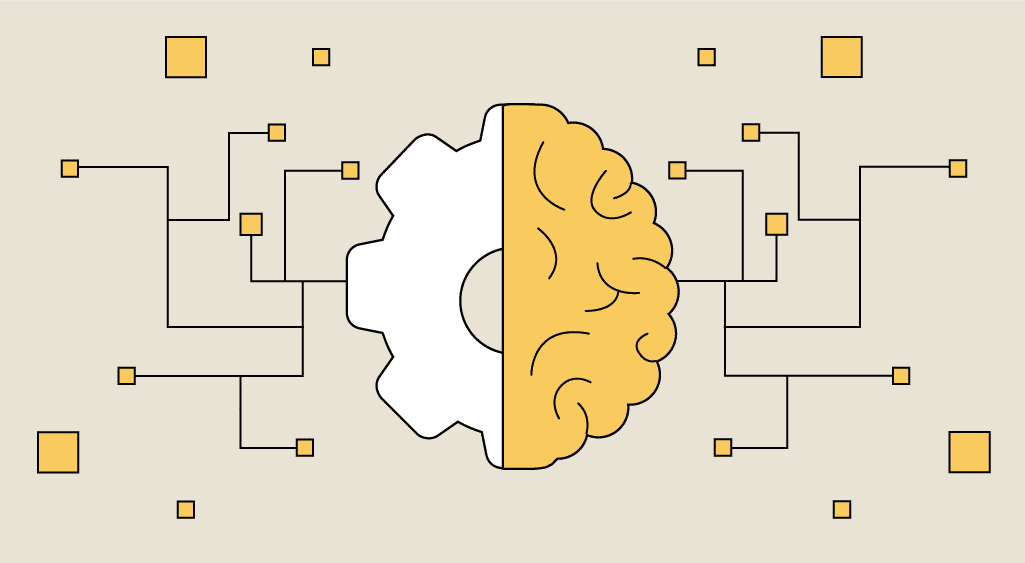
Introduction
Random Forest is one of the most powerful and widely used Ensemble Learning algorithms. It builds multiple decision trees and merges them to get a more accurate and stable prediction. It is known for its high accuracy, robustness, and ability to handle large datasets with high dimensionality.
1. What is Random Forest Regression?
Random Forest is an Ensemble Learning method that builds multiple Decision Trees and combines their predictions. In Classification, the output is the class selected by the majority of trees.
1.1 Why Use Random Forest?
Higher Accuracy: Combines multiple decision trees, reducing overfitting.
Robustness: Works well with noisy and unbalanced datasets.
Feature Importance: Identifies important features automatically.
1.2 How is it Different from a Single Decision Tree?
A single Decision Tree is prone to overfitting and variance.
Random Forest reduces variance by averaging multiple trees, making it more robust and generalizable.
2. How Does Random Forest Work?
Random Forest follows the Bagging (Bootstrap Aggregation) technique. Here's how it works:
2.1 Building the Forest:
Bootstrap Sampling: Randomly select subsets of data with replacement.
Feature Randomness: Select a random subset of features for each tree.
Build Decision Trees: Train each tree on the bootstrap sample using the selected features.
Aggregation: For classification, each tree votes for a class, and the majority vote is the final prediction.
2.2 Why Bootstrap Sampling?
Reduces Overfitting: Each tree is trained on a different subset of data, preventing overfitting.
Increases Diversity: Trees are less correlated, leading to better generalization.
2.3 Majority Voting in Classification:
For classification tasks, each tree predicts a class, and the class with the most votes becomes the final prediction.
y^ = Final prediction
hi(x) = Prediction by the ith tree
3. Mathematical Intuition
3.1 Ensemble Learning
Random Forest is a type of Ensemble Learning which combines the predictions of multiple base estimators to improve accuracy.
3.2 Why Random Features?
Reduces correlation between trees, leading to lower variance.
Improves generalization by ensuring each tree is unique.
3.3 Out-of-Bag (OOB) Error
Since each tree is trained on a bootstrap sample, about one-third of the data is left out (Out-of-Bag). These OOB samples are used to evaluate model performance without requiring a separate validation set.
N = Number of OOB samples
L = Loss function (e.g., 0-1 loss for classification)
4. Hyperparameters and Tuning
4.1 Key Hyperparameters:
n_estimators: Number of trees in the forest.
max_depth: Maximum depth of each tree.
min_samples_split: Minimum number of samples required to split a node.
min_samples_leaf: Minimum number of samples required at a leaf node.
max_features: Number of features to consider for best split.
4.2 Hyperparameter Tuning:
Grid Search and Random Search can be used for tuning.
Cross-validation is recommended to avoid overfitting.
5. Advantages and Disadvantages
5.1 Advantages:
High Accuracy: Combines multiple trees to improve accuracy and reduce overfitting.
Robustness: Handles noisy and unbalanced data well.
Feature Importance: Provides insights into feature relevance.
Versatility: Works for both classification and regression tasks.
5.2 Disadvantages:
Complexity: More complex and slower to interpret compared to a single decision tree.
Overfitting Risk: If too many trees are used, it may overfit the training data.
Large Memory Usage: Requires more memory and computational power.
6. Implementation in Python
Let’s implement Random Forest Classifier using Scikit-learn:
# Importing necessary libraries
import numpy as np
import pandas as pd
from sklearn.model_selection import train_test_split
from sklearn.ensemble import RandomForestClassifier
from sklearn.metrics import accuracy_score, classification_report, confusion_matrix
from sklearn.datasets import load_iris
import matplotlib.pyplot as plt
import seaborn as sns
# Load Dataset
data = load_iris()
X = data.data
y = data.target
# Split data into training and testing sets
X_train, X_test, y_train, y_test = train_test_split(X, y, test_size=0.2, random_state=42)
# Create Random Forest Classifier model
model = RandomForestClassifier(n_estimators=100, max_depth=3, random_state=42)
model.fit(X_train, y_train)
# Make predictions
y_pred = model.predict(X_test)
# Evaluate Model
print("Accuracy:", accuracy_score(y_test, y_pred))
print("Confusion Matrix:\n", confusion_matrix(y_test, y_pred))
print("Classification Report:\n", classification_report(y_test, y_pred))
# Feature Importance
feature_importance = model.feature_importances_
sns.barplot(x=feature_importance, y=data.feature_names)
plt.title('Feature Importance')
plt.show()
7. Real-world Applications
Finance: Credit risk modeling, fraud detection.
Healthcare: Disease prediction and diagnostics.
Marketing: Customer segmentation and personalization.
E-commerce: Product recommendation systems.
Image Classification: Object detection and image segmentation.
8. Tips for Better Performance
Feature Scaling: Not required for Random Forests.
Hyperparameter Tuning: Optimize n_estimators, max_depth, and max_features.
Ensemble Methods: Combine with other models for even better accuracy.
Cross-validation: Use k-fold cross-validation for robust evaluation.
Feature Selection: Drop irrelevant features to improve performance.
9. Conclusion
Random Forest is a powerful and versatile ensemble model that significantly improves accuracy by reducing overfitting and variance. Its robustness and interpretability make it a go-to model for many classification tasks.
9.1 Key Takeaways:
Random Forest builds multiple decision trees and averages their predictions.
It uses Bootstrap Sampling and Random Feature Selection for diversity.
Provides high accuracy and robustness against overfitting.
Suitable for both Classification and Regression tasks.
Offers insights into feature importance.
Subscribe to my newsletter
Read articles from Tushar Pant directly inside your inbox. Subscribe to the newsletter, and don't miss out.
Written by
