The Illusion of Reasoning: Unmasking the Reality of 'Reasoning' Models Like Claude 3.7 Sonnet

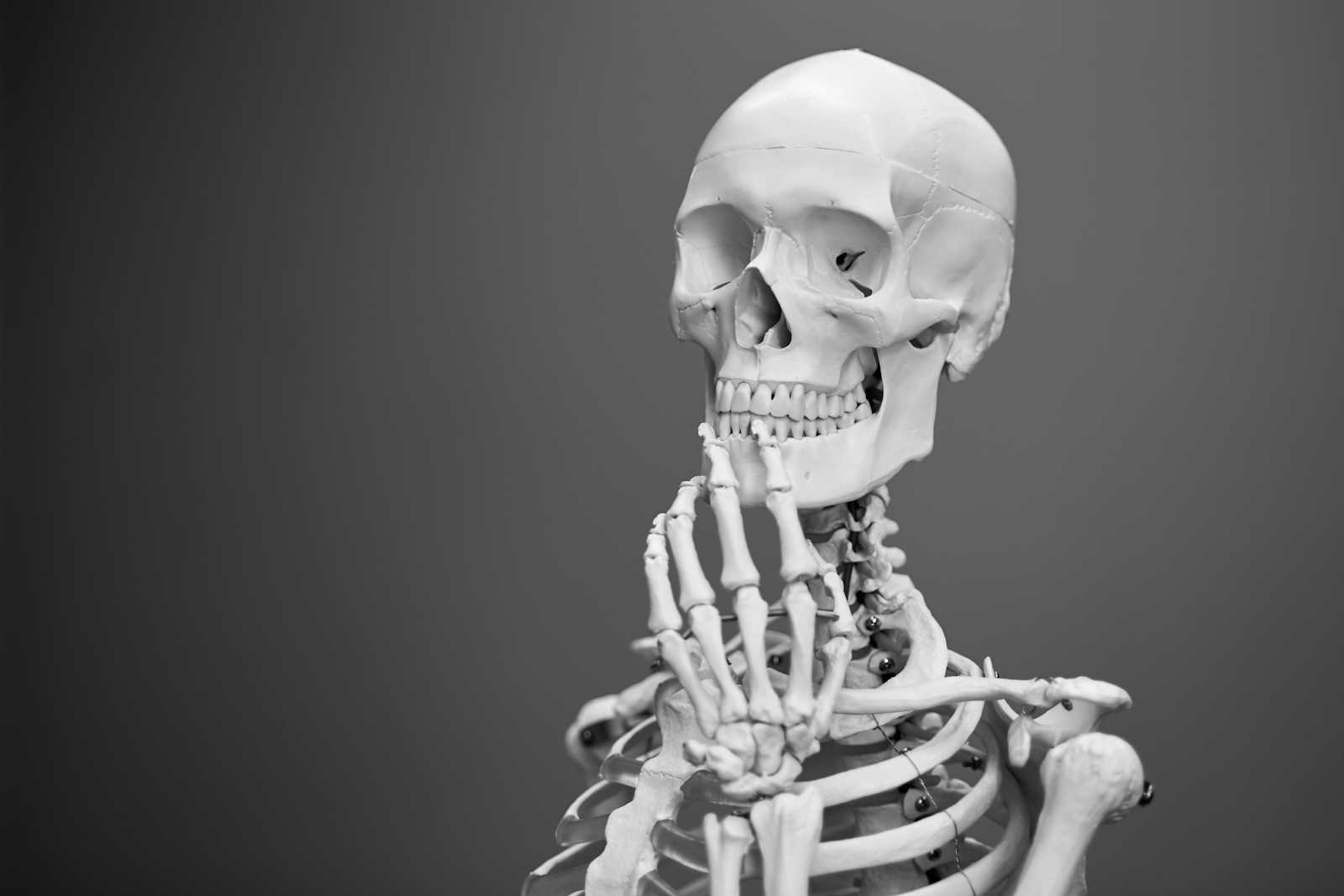
In the realm of artificial intelligence, we are witnessing one of the most remarkable feats of technological rebranding in history—though not for the reasons commonly celebrated. The transformer architecture, fundamentally unchanged since its 2017 introduction, has been masterfully repackaged from a specialized language processing tool into something far grander in the public imagination: a reasoning engine.
The Marketing Metamorphosis
The story begins with the original Transformer architecture introduced in Google's "Attention Is All You Need" paper. Its purpose was clear and specific: advancing natural language processing through a novel attention mechanism. Unlike today's grandiose claims, the original goals were remarkably straightforward—process sequences of tokens, learn patterns in language data, and generate probable next tokens.
Fast forward to 2022-2023, and we witnessed a watershed moment with ChatGPT's release—not in technical capability, but in public perception. Through careful positioning and marketing prowess, the industry transformed the public's understanding of what was essentially the same technology. Where once stood a "language model" now stood an "AI intelligence." The "pattern completion system" became a "reasoning engine." This remarkable pivot happened without any fundamental change to the underlying technology.
The Technical Reality Check
While marketing narratives soared, technical research began revealing fundamental limitations. August 2023 brought a landmark paper directly challenging GPT-4's reasoning abilities, demonstrating a fundamental inability to handle systematic reasoning tasks. The paper showed that "flashes of brilliance" were merely pattern-matching artifacts, highlighting the dangerous gap between marketing claims and technical reality.
More recently, in October 2024, Apple's comprehensive study extended these findings to frontier models including OpenAI's o1 series. This research proved that reasoning limitations are fundamental to the transformer architecture, showing performance collapses with minimal complexity increases and confirming that LLMs perform pattern matching, not logical reasoning.
These papers didn't just challenge specific models—they exposed the fundamental disconnect at the heart of transformer marketing. While companies promoted their models as capable of "system 2 thinking" and "PhD-level reasoning," technical research revealed high variance in performance on similar problems, brittleness when facing slight variations, sensitivity to irrelevant information, and an inability to maintain consistent logical chains.
The Illusion of Claude 3.7 Sonnet's "Reasoning"
This brings us to Anthropic's Claude 3.7 Sonnet, touted as a breakthrough "reasoning model." Peel back the glossy benchmarks—impressive scores like 80.0% on AIME 2024 or 96.2% on MATH 500—and the reality is far less dazzling. These models, with their token-heavy "extended thinking" modes, are less a leap forward in reasoning and more a mirage built on excessive compute, impractical latency, and a blissful disregard for the quality of their supporting data.
The Excessive Token Cushion: A Costly Illusion
At first glance, Claude 3.7 Sonnet's extended thinking mode, with its 64K-token "cushion" ($0.96 per query), seems impressive. Benchmarks show massive gains—up to 56.7% improvement on high school math competitions like AIME 2024—suggesting a model that can reason deeply across tasks. But this token bloat, as one X user lamented in late February 2025, "burns tokens like crazy for marginal gains, making it feel like a cash grab for niche use cases."
The reality is stark: most users don't have the patience or budget for 64K tokens, which can take minutes to process, rendering it impractical for real-time applications. This excessive cushion, optimized for specific benchmarks, masks an inefficiency that becomes apparent when we scale down to practical use.
Scaling Down to Reality: A Few Thousand Tokens and Diminishing Returns
When we adjust to a realistic token budget—say, 5K to 10K tokens ($0.075-$0.15)—the promise of improvement begins to fray. The benchmarks' percentage gains (e.g., +14% on MATH 500, +3.2% on visual reasoning) are maxed-out peaks, not averages, and logarithmic scaling means most users see diminishing returns.
Another X user posted in early March 2025, "Claude 3.7's extended thinking overcomplicates simple questions, lagging for minutes with barely better answers—hardly worth the token hit." This reflects a broader truth: for non-math tasks like general knowledge or writing, the mode often delivers little to no gain, or even underperforms, introducing errors or verbosity that degrade usability.
The Promise, Not Certainty, of Improvement: A Gamble on Data and Prompting
Perhaps most damning is the realization that these "reasoning" improvements are a gamble, not a guarantee. The mode's performance hinges on pretraining data quality and coverage—gaps Anthropic rarely acknowledges. An AI can't suddenly develop theoretical physics knowledge without relevant data; token generation can't compensate for deficiencies in the model's foundation.
This disconnect exposes the benchmarks as misleading, cherry-picked for math-heavy tasks where data is rich, but unreliable for broader domains like creative writing or niche industries. A simple prompt strategy—asking for structured output before the final answer—can replicate the mode's behavior at a fraction of the cost and time, revealing extended thinking as a redundant feature optimized for benchmarks, not real-world value.
The Contextual Limits of Percentages: Math-Centric Benchmarks Don't Translate
The reliance on percentages to sell reasoning models is particularly deceptive. They suggest linear, predictable gains, but in practice, these figures are tied to narrow, structured problems like math competitions, not the dynamic, ambiguous tasks users encounter daily.
For most writing, general knowledge, or agentic tool use, the mode's token-heavy reasoning offers little benefit, often lagging behind standard mode or competitors like OpenAI's o3-mini and DeepSeek's R1, which achieve comparable results without the overhead. This math-centric focus, as one X user noted in late February 2025, "makes Claude 3.7 feel like a math nerd in a poetry slam—great for AIME, useless for everything else."
The Industry Impact
The AI industry has embraced the transformer rebranding with costly enthusiasm. Companies, driven by FOMO and market pressures, rush to implement "AI intelligence" solutions that are often misaligned with their actual needs. This has led to inflated expectations that lead to failed projects, misguided implementations that solve the wrong problems, massive investments in capabilities that don't exist, and accumulating technical debt from premature AI adoption.
Perhaps the most profound cost of this rebranding lies in its social implications. The public, bombarded with messages about "intelligent AI" and existential risks, struggles to distinguish between science fiction and technical reality. This confusion has led to widespread misconceptions about AI capabilities, policy discussions based on imagined rather than actual risks, ethical debates that miss the mark, and erosion of trust in genuine technological advances.
Conclusion
The harsh reality of "reasoning" models like Claude 3.7 Sonnet is sobering. Their extended thinking mode is a wasteful, niche tool—impressive in controlled labs but impractical for most users, burdened by excessive tokens, latency, and data gaps. The benchmarks dazzle, but they obscure a system that gambles on improvement, delivers inconsistent results, and relies on pretraining quality it won't disclose.
The journey of the transformer architecture—from specialized NLP tool to perceived harbinger of artificial general intelligence—stands as a testament to the power of narrative crafting in technology. Through careful authority stacking and strategic messaging, a pattern-matching system has been elevated to mythological status, creating ripple effects throughout academia, industry, and society.
As we look toward the future of AI development, our greatest challenge may not be technical but narrative: how do we maintain scientific integrity while navigating the powerful currents of public perception and institutional authority? The answer lies in returning to fundamental truths—understanding what these systems actually are and can do, rather than what marketing suggests they might become.
Until AI developers prioritize transparency on data coverage, efficiency in design, and real-world applicability over benchmark scores, these models will remain more illusion than innovation—sophisticated pattern matchers masquerading as reasoning systems, exactly what the original transformer architecture was designed to be.
Subscribe to my newsletter
Read articles from Gerard Sans directly inside your inbox. Subscribe to the newsletter, and don't miss out.
Written by

Gerard Sans
Gerard Sans
I help developers succeed in Artificial Intelligence and Web3; Former AWS Amplify Developer Advocate. I am very excited about the future of the Web and JavaScript. Always happy Computer Science Engineer and humble Google Developer Expert. I love sharing my knowledge by speaking, training and writing about cool technologies. I love running communities and meetups such as Web3 London, GraphQL London, GraphQL San Francisco, mentoring students and giving back to the community.