Data Cleansing in SQL: Enhancing Data Quality with Traditional and AI-Driven Approaches
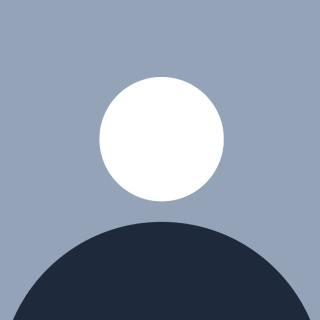
Data quality is a critical concern in modern database systems. Traditional data cleansing in SQL has long been the standard approach for addressing issues like duplicate records, missing values, and data inconsistencies. As organizations increasingly adopt generative AI solutions, questions arise about whether these traditional methods remain relevant. This article explores how SQL-based data cleaning continues to play an essential role, while also examining how generative AI complements and enhances data quality processes. We'll investigate the relationship between clean data and AI systems, demonstrating why proper data preparation remains fundamental even in an AI-driven landscape.
Core Concepts of Traditional Data Cleansing
Understanding Basic Data Quality Issues
Database systems frequently encounter several common data quality challenges that require systematic cleaning approaches. These issues can significantly impact business operations and decision-making processes if left unaddressed. The primary focus of traditional data cleansing revolves around identifying and correcting these fundamental data problems through SQL-based solutions.
Duplicate Record Management
Duplicate records represent one of the most prevalent data quality issues in databases. These duplicates can arise from multiple data entry points, system integration issues, or user error. When left unchecked, duplicate records can lead to inflated metrics, incorrect reporting, and misguided business decisions. SQL provides robust mechanisms for identifying and removing duplicates through techniques like GROUP BY clauses and window functions.
Missing Value Resolution
Databases often contain gaps where data should exist. These missing values can stem from incomplete form submissions, system failures, or data transmission errors. SQL offers various approaches to handle missing values, including:
Default value substitution
Statistical imputation using averages or medians
Pattern-based value prediction
Null value management
Data Standardization Processes
Maintaining consistent data formats across database systems is crucial for reliable operations. Standardization efforts typically focus on:
Date format normalization
Currency standardization
Unit conversion consistency
Text case and format uniformity
Anomaly Detection and Handling
Identifying and addressing data anomalies forms a critical component of the cleansing process. Statistical methods help identify outliers that could indicate either valuable insights or data errors. SQL queries can calculate standard deviations, interquartile ranges, and other statistical measures to flag potential anomalies for review. This process requires careful balance to avoid removing legitimate but unusual data points while correcting genuine errors.
Generative AI's Role in Data Analysis
The Three-Layer Architecture
Generative AI systems employ a sophisticated three-layer architecture when processing structured data. This framework consists of semantic, metadata, and context layers, each serving distinct yet interconnected functions in data analysis. Understanding these layers helps explain how AI systems interpret and process structured data differently from traditional SQL approaches.
Semantic Layer Capabilities
The semantic layer serves as the foundation for data comprehension. It creates unified knowledge graphs by identifying and connecting related entities within datasets. This layer excels at:
Building comprehensive entity relationships
Automating schema mapping processes
Resolving entity disambiguation challenges
Maintaining data consistency across sources
Metadata Layer Functions
Acting as a crucial bridge between semantic understanding and contextual awareness, the metadata layer enriches data interpretation through:
Data field annotation and mapping
Relationship definition between entities
Structure documentation and maintenance
Cross-reference management
Context Layer Operations
The context layer provides critical domain-specific understanding and real-time adaptability. It employs Named Entity Recognition (NER) to process natural language inputs effectively. This layer's primary functions include:
Processing natural language queries
Filtering irrelevant information
Applying domain-specific knowledge
Generating appropriate SQL queries
Integration Benefits
When these three layers work in harmony, generative AI systems can overcome traditional data analysis limitations. They excel at handling complex scenarios like fuzzy matching, natural language processing, and context-aware analysis. This integrated approach enables more sophisticated data interpretation and analysis than conventional SQL-only solutions, while still maintaining the importance of clean, well-structured data as a foundation.
Practical Examples and Limitations
Real-World Data Challenges
Consider a consumer goods company's database structure, where common data issues emerge across order processing, product management, and customer information. These real-world scenarios demonstrate both the necessity of data cleansing and its inherent challenges. Multiple tables often contain interconnected data quality issues that require systematic resolution approaches.
Handling Duplicate Orders
Order systems frequently encounter duplicate entries that can severely impact business metrics. For example, when identical orders appear multiple times, revenue calculations become inflated, leading to incorrect business insights. SQL provides tools to identify and remove these duplicates through careful comparison of key fields like order ID, customer ID, and transaction dates.
Customer Data Consistency
Customer information often suffers from inconsistencies such as multiple spellings of names, varying address formats, or duplicate profiles. These issues can lead to fragmented customer views and ineffective marketing efforts. Traditional cleaning methods must carefully balance between combining similar records and maintaining distinct customer identities.
Traditional Cleansing Constraints
While SQL-based data cleansing offers powerful tools, it faces several significant limitations:
Manual intervention requirements for issue identification
Reactive rather than proactive problem solving
Limited pattern recognition capabilities
Difficulty handling complex string matching scenarios
Time-intensive query development and testing
Beyond Basic Cleansing
Advanced data quality challenges often exceed traditional SQL capabilities. Fuzzy matching, for instance, requires sophisticated pattern recognition that basic REGEXP or LIKE operators cannot provide. Similarly, contextual understanding of data relationships and business rules often requires more advanced solutions than traditional SQL can offer. These limitations highlight the potential value of combining traditional cleansing methods with modern AI-driven approaches.
Future Integration Paths
The future of data cleansing likely lies in hybrid approaches that combine traditional SQL methods with AI-enhanced capabilities. This integration can provide more robust solutions for complex data quality challenges while maintaining the reliability and precision of traditional cleaning methods where they excel.
Conclusion
Traditional data cleansing techniques and generative AI each bring unique strengths to data quality management. SQL-based cleansing remains essential for foundational data quality tasks, providing precise control over duplicate removal, standardization, and basic data corrections. These traditional methods offer reliability and transparency that businesses depend on for their core operations.
Generative AI enhances rather than replaces these traditional approaches. Its three-layer architecture - semantic, metadata, and context - enables sophisticated data understanding and processing that extends beyond SQL's capabilities. This is particularly valuable for complex scenarios involving natural language processing, fuzzy matching, and context-aware analysis.
The optimal approach combines both methodologies. Organizations should maintain strong traditional data cleansing practices while strategically implementing AI-driven solutions where they add the most value. This hybrid strategy ensures robust data quality while leveraging advanced capabilities for complex scenarios.
As data systems continue to evolve, the relationship between traditional cleansing and AI will likely grow more intertwined. Success in modern data management requires understanding both approaches' strengths and limitations, implementing them appropriately, and maintaining focus on the ultimate goal: providing reliable, accurate data for business decision-making.
Subscribe to my newsletter
Read articles from Mikuz directly inside your inbox. Subscribe to the newsletter, and don't miss out.
Written by