3 Ways AI is Transforming Metadata for Large-Scale Projects

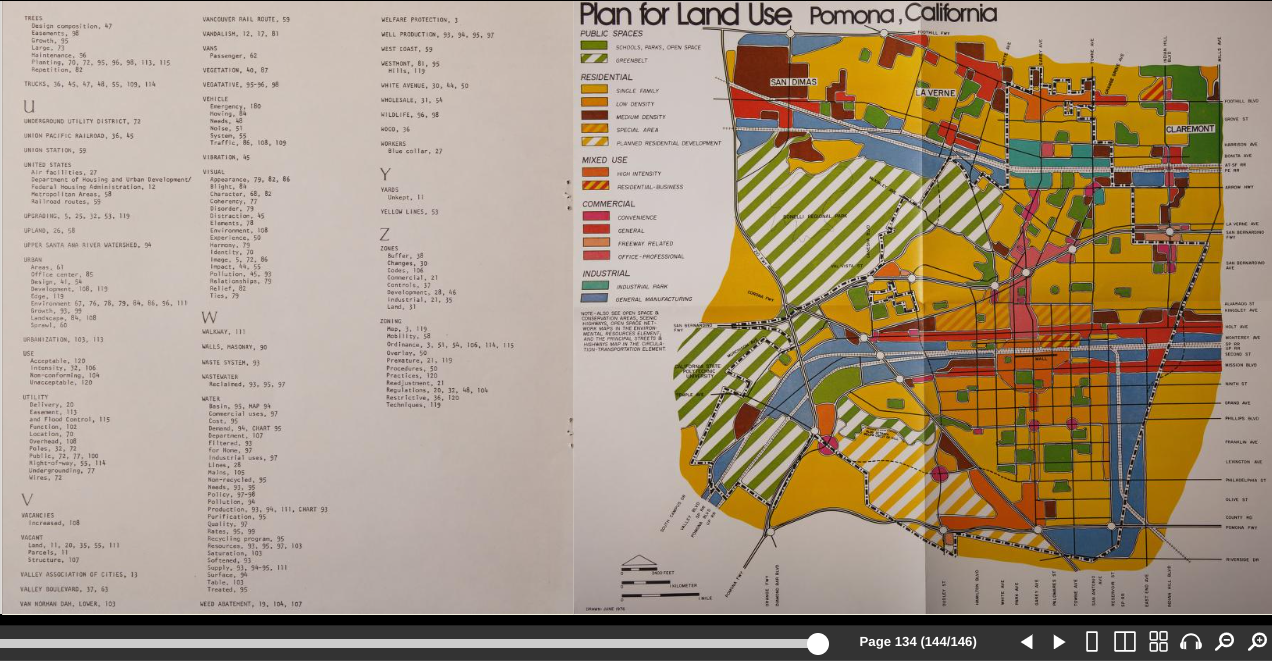
📷 Image Reference: IGSL Document Example from Internet Archive
As part of my work with AI, I’ve been using a collection of documents at UC Berkeley’s Institute of Governmental Studies Library (IGSL) to experiment with. My focus has been testing how AI can structure metadata, generate new insights, and ultimately help researchers discover the right materials more efficiently.
📌 Three Areas I’ve Been Exploring…
Extracting Metadata from Existing Documents
Before AI can harness metadata, it first needs to pull what’s already there. Since AI doesn’t inherently “know” where to find metadata, we have to tell it where to retrieve metadata that has been prepared on sites like Internet Archive, where the IGSL collections is hosted.
Of the three levels of metadata extraction I’ve been exploring, this process is the simplest. However, it is the foundation of everything that comes next.
Creating AI-Driven Metadata
While AI can pull existing metadata, there are times when new metadata needs to be created. This is where AI moves beyond simple extraction and starts generating summaries, keywords, and researcher classifications. However, creating metadata is more complex than extracting it because AI must make interpretive decisions.
Unlike existing metadata, which tends to follow a clear format, AI-generated metadata has a degree of subjectivity—it requires AI to define meaning, relevance, and audience alignment.
Example:
Say we want AI to determine who a document is most relevant to—should it be categorized for historians, political researchers, or economists? This requires AI to analyze the document's content, but because research relevance is nuanced, AI may struggle to stay on track.
To help guide AI, we can train it to reference existing foundational metadata as an early-stage guardrail. Even though AI is generating new metadata, checking against structured metadata (such as title, author, or original subject tags) can help keep its classification process aligned. This ensures the metadata it generates is based on both structured data and deeper content understanding. (an early-stage "compass", helping AI stay on course)
AI-Assisted Research Discovery & Interlinked Data
Once AI creates metadata or generates new insights, the next step is using that data to connect researchers with the right materials. This is where metadata stops being just an organizational tool and starts enhancing research discovery.
The real power of AI emerges when it doesn’t just describe documents but links them together—helping researchers find related materials, cross-references, and even external sources that expand their understanding.
Examples of AI-Assisted Research Discovery:
AI-powered recommendations within IGSL’s collection 🔍
AI suggests related materials based on themes, keywords, and researcher classifications.
A historian looking at one document might recommend three others from the same collection that share similar topics.
Interlinked metadata across research networks 🔗📎
AI can extend beyond IGSL’s collection, linking documents to modern news reports, policy papers, and academic research.
A government report from the 1960s on housing policies could be connected to recent economic studies or legislative updates.
Personalized research notifications & automated alerts 🎯
Instead of researchers searching for documents manually, AI can proactively notify them about relevant materials.
A political scientist focusing on urban planning could receive a weekly email with newly discovered policy documents.
While metadata is often seen as a static tool that informs audiences about the contents of documents, it also has the potential to become a dynamic system—one that actively helps researchers make unexpected but valuable connections.
This isn’t just the next stage or frontier of metadata—it’s already here. The tools to build intelligent, research-focused systems are at our fingertips—it’s just a matter of how we use them.
If you have questions or you're working with large-scale digital collections, I'd love to hear how you're thinking about AI-powered research automation! 🚀
Subscribe to my newsletter
Read articles from Nick Norman directly inside your inbox. Subscribe to the newsletter, and don't miss out.
Written by
