Building a HybridRAG System for Financial Document Analysis: An End-to-End flow

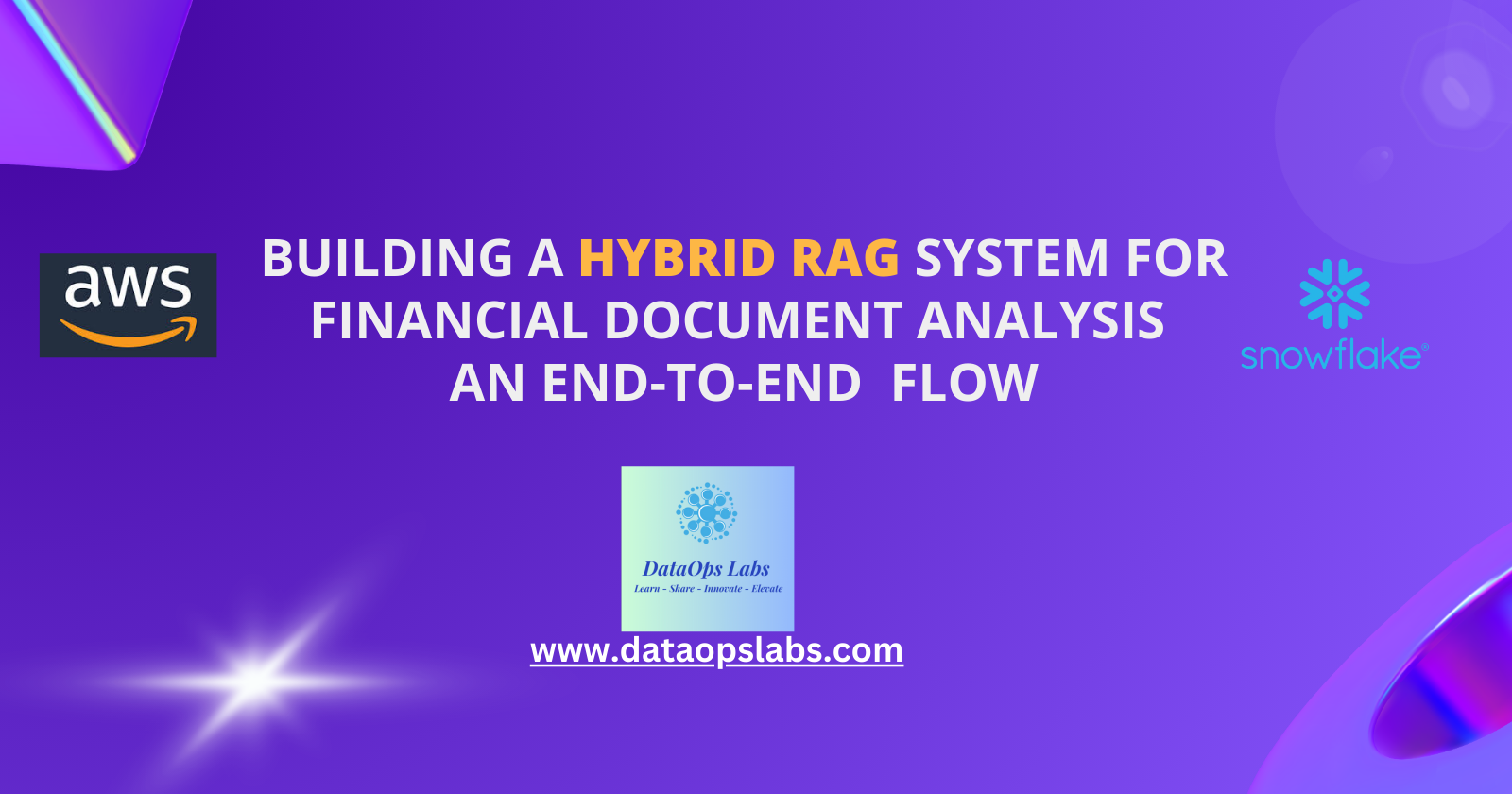
Introduction
Financial document analysis presents unique challenges for Large Language Models (LLMs). Domain-specific terminology, complex relationships, and the sheer volume of data demand a sophisticated approach. Hybrid Retrieval-Augmented Generation (HybridRAG) offers a powerful solution by combining the strengths of VectorRAG and GraphRAG. This blog post provides a comprehensive guide to building a HybridRAG system, covering everything from data ingestion to deployment, and leveraging cutting-edge technologies like Cortex Search, Cortex Native Embedding Model, Amazon Bedrock, and AWS Neptune.
1. Understanding the HybridRAG Advantage
Briefly recap the limitations of traditional VectorRAG and GraphRAG when applied to financial documents (summarize points from the whitepaper introduction).
Hybrid Approach Benefits: Explain how HybridRAG overcomes these limitations by:
Providing richer context through both semantic similarity (VectorRAG) and structured relationships (GraphRAG).
Improving accuracy and reducing hallucination by grounding responses in verifiable data.
Enabling more nuanced insights by capturing complex connections between financial entities and metrics.
2. Architecture Overview: A High-Level View
Present a simplified architecture diagram illustrating the key components of the HybridRAG system:
Data Ingestion (S3)
Document Chunking
Cortex Embedding Generation
Cortex Search (Vector Database)
Bedrock LLM for Knowledge Graph Construction
AWS Neptune (Graph Database)
Hybrid Retrieval and Fusion Logic
Bedrock LLM for Response Generation
User Interface (e.g., Streamlit)
Key Technologies: Briefly introduce each technology and its role in the architecture.
3. Step-by-Step Implementation Guide
This section will walk the reader through the process of building the HybridRAG system. It will be divided into sub-sections, each covering a specific stage of the implementation.
3.1. Setting Up the Infrastructure
AWS Account and Permissions: Provide a checklist of AWS resources needed and the necessary IAM permissions (S3, SageMaker (if using it for custom model training), Neptune, Lambda, Bedrock access).
Cortex Setup:
Explain how to deploy Cortex Search and the Cortex Native Embedding Model.
Provide instructions on obtaining API keys and configuring access.
Link to Cortex documentation for detailed setup instructions.
Bedrock Access: Explain how to request access to different LLMs within Amazon Bedrock (Anthropic Claude, AI21 Labs Jurassic-2, etc.).
Snowflake Setup: If integrating with Snowflake, provide instructions on creating a Snowflake account, setting up a warehouse, and creating a database.
3.2. Data Ingestion and Preprocessing
Loading Financial Documents into S3: Explain how to load financial documents (e.g., earnings reports) into an S3 bucket.
- Code Example:
import boto3
s3 = boto3.client('s3')
bucket_name = 'your-bucket-name'
file_path = 'path/to/your/document.pdf'
key = 'financial_documents/amazon_earnings_2024.pdf'
s3.upload_file(file_path, bucket_name, key)
print(f"File uploaded to s3://{bucket_name}/{key}")
Document Chunking: Discuss different chunking strategies (fixed-size, semantic chunking).
- Code Example (Conceptual):
# Simplified chunking example
def chunk_document(text, chunk_size=512):
chunks = [text[i:i + chunk_size] for i in range(0, len(text), chunk_size)]
return chunks
3.3. Building the VectorRAG Component
Generating Embeddings with Cortex Native Embedding Model:
- Provide a detailed code example for calling the Cortex API to generate embeddings for each chunk.
import requests
import json
CORTEX_API_KEY = "YOUR_CORTEX_API_KEY"
CORTEX_EMBEDDING_ENDPOINT = "YOUR_CORTEX_EMBEDDING_ENDPOINT"
def get_cortex_embedding(text):
headers = {
"Authorization": f"Bearer {CORTEX_API_KEY}",
"Content-Type": "application/json"
}
data = {"text": text}
response = requests.post(CORTEX_EMBEDDING_ENDPOINT, headers=headers, data=json.dumps(data))
response.raise_for_status()
return response.json()["embedding"]
# Example usage
text_chunk = "Amazon's AWS revenue increased by 12% in Q1 2024."
embedding = get_cortex_embedding(text_chunk)
print(f"Embedding: {embedding[:10]}...") # Print first 10 values
Storing Embeddings in Cortex Search:
- Provide a code example for indexing the embeddings in Cortex Search, along with relevant metadata (document ID, chunk number, etc.).
CORTEX_SEARCH_API_KEY = "YOUR_CORTEX_SEARCH_API_KEY"
CORTEX_SEARCH_INDEX_ENDPOINT = "YOUR_CORTEX_SEARCH_INDEX_ENDPOINT"
def add_to_cortex_search(document_id, embedding, metadata={}):
headers = {
"Authorization": f"Bearer {CORTEX_SEARCH_API_KEY}",
"Content-Type": "application/json"
}
data = {
"document_id": document_id,
"embedding": embedding,
"metadata": metadata
}
response = requests.post(CORTEX_SEARCH_INDEX_ENDPOINT, headers=headers, data=json.dumps(data))
response.raise_for_status()
return response.json()
# Example
document_id = "amazon_earnings_2024_chunk_1"
metadata = {"source": "Amazon 2024 Earnings Report", "type": "Financial Report"}
result = add_to_cortex_search(document_id, embedding, metadata)
if result.get("success"):
print(f"Successfully indexed document {document_id} in Cortex Search")
else:
print(f"Error indexing: {result}")
3.4. Building the GraphRAG Component
Knowledge Graph Construction with Bedrock LLMs:
- Explain how to use prompt engineering with Bedrock LLMs (e.g., Anthropic Claude) to extract entities and relationships from the financial documents. Refer to the two-tiered LLM chain mentioned in the "paste.txt" document.
import boto3
import json
BEDROCK_REGION = "your-aws-region"
BEDROCK_MODEL_ID = "anthropic.claude-v2" # Or another suitable Bedrock model
bedrock = boto3.client(service_name='bedrock-runtime', region_name=BEDROCK_REGION)
def extract_triplets_with_bedrock(text_chunk):
prompt = f"""
You are an expert in financial document analysis. Extract the key entities and relationships from the following text chunk. Return the result as a list of (subject, predicate, object) triplets.
Text Chunk: {text_chunk}
Triplets:
"""
body = json.dumps({
"prompt": prompt,
"max_tokens_to_sample": 500, # Adjust as needed
"temperature": 0.0, # For deterministic extraction
"top_p": 1 # Use top_p sampling
})
modelId = BEDROCK_MODEL_ID
accept = 'application/json'
contentType = 'application/json'
response = bedrock.invoke_model(body=body, modelId=modelId, accept=accept, contentType=contentType)
response_body = json.loads(response.get('body').read())
# Parse the LLM's response to extract the triplets. This will depend on the output format of the LLM.
triplets = parse_triplets_from_llm_response(response_body['completion'])
return triplets
# Example
text_chunk = "Amazon's revenue increased by 15% to $574.8 billion in 2024, with AWS contributing significantly to the growth."
triplets = extract_triplets_with_bedrock(text_chunk)
print(f"Extracted Triplets: {triplets}")
# Important: Implement the parse_triplets_from_llm_response function
# This function needs to take the LLM's text output and convert it into a list of tuples.
Discuss prompt engineering techniques to improve the accuracy of entity and relationship extraction.
- Loading Knowledge Graph into AWS Neptune:
Provide a code example for loading the extracted triplets into AWS Neptune.
import boto3
from gremlin_python import traversal
from gremlin_python.driver.driver_remote_connection import DriverRemoteConnection
NEPTUNE_ENDPOINT = "YOUR_NEPTUNE_ENDPOINT" # e.g., "wss://..."
NEPTUNE_PORT = 8182
def load_triplets_to_neptune(triplets):
try:
conn = DriverRemoteConnection(f'wss://{NEPTUNE_ENDPOINT}:{NEPTUNE_PORT}/gremlin', 'g')
g = traversal().withRemote(conn)
for subject, predicate, object in triplets:
# Add vertices and edges
g.addV(subject).property('name', subject).next()
g.addV(object).property('name', object).next()
g.V(subject).addE(predicate).to(g.V(object)).next()
conn.close()
print("Triplets loaded successfully into Neptune.")
except Exception as e:
print(f"Error loading triplets into Neptune: {e}")
# Example usage:
sample_triplets = [
("Amazon", "revenue", "$574.8 billion"),
("Amazon", "growth", "15%"),
("AWS", "contributes_to", "Amazon revenue")
]
load_triplets_to_neptune(sample_triplets)
3.5. Hybrid Retrieval and Fusion
Querying Cortex Search: Show how to query Cortex Search with a user's question to retrieve relevant text chunks.
# (Code snippet from previous Cortex Search section, adapted for querying)
Querying AWS Neptune: Demonstrate how to query AWS Neptune to retrieve relevant entities and relationships based on the user's question. Provide example Gremlin queries.
# Example Gremlin query to find all companies related to "AWS" gremlin_query = "g.V('AWS').inE('related_to').outV().valueMap()" # Execute the Gremlin query using boto3 and the Neptune API (similar to the load_triplets example)
Fusion Strategies: Explain and demonstrate how to combine the results from Cortex Search and AWS Neptune. Options include:
Ranking/Scoring: Combine the relevance scores from both retrieval methods.
Filtering: Use the GraphRAG results to filter the VectorRAG results (e.g., only keep results that are connected to a specific entity in the KG).
Enrichment: Use the GraphRAG results to add more information to the VectorRAG results (e.g., append related entities and relationships to the text chunks).
3.6. Response Generation with Bedrock LLMs
Explain how to format the combined context from Cortex Search and AWS Neptune and pass it to a Bedrock LLM for response generation.
# (Code snippet from previous Bedrock LLM interaction section, adapted for HybridRAG context)
Discuss prompt engineering strategies to ensure the LLM effectively uses the combined context.
Example Prompts:
"Based on this text from Cortex Search: [text_chunks] and this knowledge graph information from Neptune: [entities_and_relationships], answer the question: [user_question]"
"You are a financial expert. Use the following information to answer the question: [user_question]. Relevant text: [text_chunks]. Related entities and relationships: [entities_and_relationships]"
4. Deployment and Scaling
Deploying the HybridRAG System as an AWS Lambda Function: Explain how to package the code and deploy it as a Lambda function.
API Gateway Integration: Discuss the use of API Gateway to create an API endpoint for the Lambda function.
Scaling Considerations:
Scaling Cortex Search: Discuss the scalability options for Cortex Search (e.g., increasing the number of replicas).
Scaling AWS Neptune: Discuss the scalability options for AWS Neptune (e.g., increasing instance size, adding read replicas).
Scaling Bedrock: Highlight Bedrock's ability to handle concurrent requests.
Subscribe to my newsletter
Read articles from DataOps Labs directly inside your inbox. Subscribe to the newsletter, and don't miss out.
Written by

DataOps Labs
DataOps Labs
I'm Ayyanar Jeyakrishnan ; aka AJ. With over 18 years in IT, I'm a passionate Multi-Cloud Architect specialising in crafting scalable and efficient cloud solutions. I've successfully designed and implemented multi-cloud architectures for diverse organisations, harnessing AWS, Azure, and GCP. My track record includes delivering Machine Learning and Data Platform projects with a focus on high availability, security, and scalability. I'm a proponent of DevOps and MLOps methodologies, accelerating development and deployment. I actively engage with the tech community, sharing knowledge in sessions, conferences, and mentoring programs. Constantly learning and pursuing certifications, I provide cutting-edge solutions to drive success in the evolving cloud and AI/ML landscape.