The Importance of Data Quality in Analytics

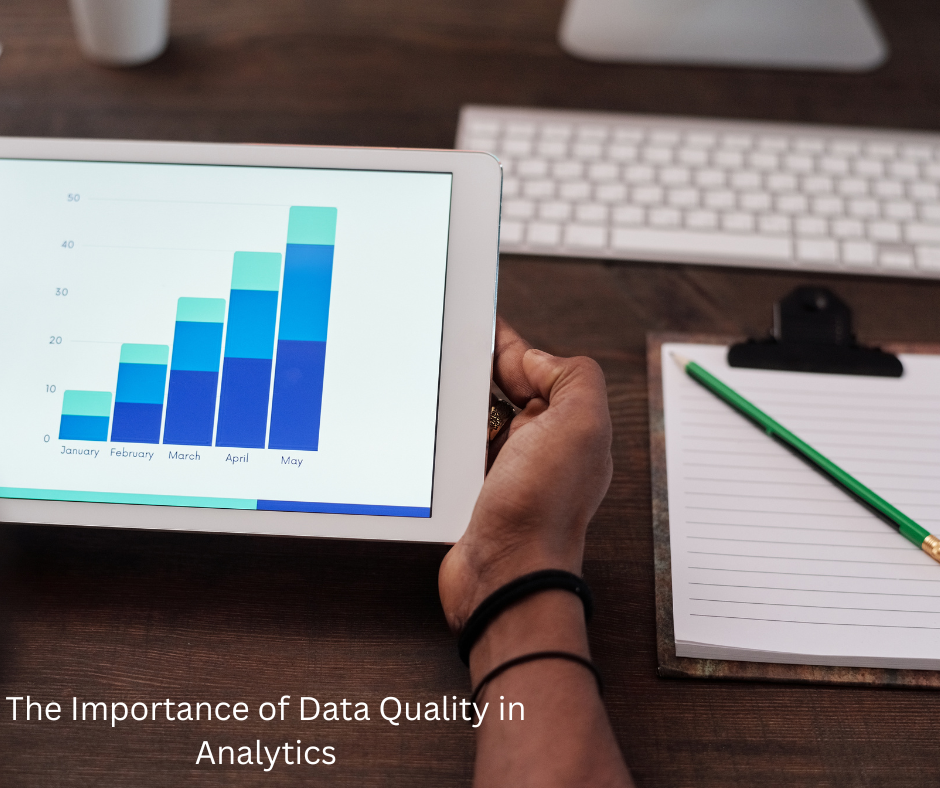
Data has become a vital asset for organizations and businesses across the globe. With the increasing use of data analytics, companies rely heavily on the insights provided by the data to make informed decisions. However, the value of data is only realized when its quality is high. Data quality plays a pivotal role in ensuring accurate, reliable, and actionable insights. In this article, we will discuss why data quality matters in analytics and how it impacts decision-making processes.
What Is Data Quality?
Data quality refers to the condition or state of data based on several factors, including accuracy, completeness, consistency, reliability, and timeliness. High-quality data is accurate, well-organized, and up-to-date, making it useful for generating insights and supporting business decisions. Poor-quality data, on the other hand, can lead to misleading conclusions, which may negatively impact business performance.
Why Is Data Quality Important in Analytics?
1. Accurate Decision-Making
Data analytics serves as the foundation for decision-making in organizations. When data is of poor quality, the insights derived from it are also flawed. Inaccurate or incomplete data leads to incorrect conclusions, which can result in costly mistakes. For example, if a business uses inaccurate sales data to forecast future performance, it could make poor investment decisions that could harm profitability.
Ensuring high-quality data helps organizations make well-informed, accurate decisions based on real insights.
2. Enhances Business Performance
High-quality data helps businesses identify key trends, customer preferences, market dynamics, and operational inefficiencies. With this valuable information, businesses can optimize processes, improve products or services, and achieve better results.
For example, companies that maintain clean and reliable customer data can tailor their marketing strategies more effectively, boosting engagement and customer satisfaction. By leveraging data quality, businesses can gain a competitive edge and achieve long-term success.
3. Improved Predictive Analytics
Predictive analytics involves using historical data to make forecasts about future events or behaviors. The accuracy of predictive models depends on the quality of the data used to build them. If the data is incomplete, outdated, or inconsistent, predictions will be unreliable.
With high-quality data, predictive analytics can offer valuable insights, such as predicting customer churn, identifying potential market opportunities, or forecasting inventory needs. Accurate forecasts enable businesses to plan more effectively and allocate resources efficiently.
4. Minimizing Risks
When data quality is compromised, it can introduce risks into business operations. For instance, errors in financial data can lead to incorrect tax filings, penalties, or audits. Poor customer data can result in improper targeting or miscommunications, damaging brand reputation.
By maintaining high-quality data, businesses can minimize these risks and avoid legal, financial, or reputational consequences. Ensuring data quality is an essential aspect of risk management in data-driven environments.
Key Dimensions of Data Quality
Understanding the different aspects of data quality is critical in ensuring that data is reliable and useful for analytics. The following are some of the key dimensions of data quality:
1. Accuracy
Accuracy refers to how closely the data represents the real-world scenario it is intended to reflect. For example, customer contact details like phone numbers or email addresses should be precise and up-to-date. Data that is inaccurate can lead to misinterpretation and flawed analytics.
2. Completeness
Data completeness refers to whether all necessary data is present and accounted for. Missing values or incomplete datasets can distort analytics and create gaps in understanding. For example, a customer database missing key demographic information might hinder personalized marketing efforts.
3. Consistency
Consistency ensures that the data does not contain contradictions across different sources or within the same dataset. Inconsistent data can cause confusion and lead to incorrect conclusions. For example, if a customer’s name is recorded differently in multiple systems (e.g., John vs. Jon), it can lead to inefficiencies in data processing.
4. Timeliness
Timeliness refers to how up-to-date the data is. In the world of analytics, timely data is crucial. Outdated data can result in irrelevant insights, especially when working with fast-paced industries. For instance, outdated inventory data may cause businesses to overstock or understock products.
5. Reliability
Reliable data is stable and consistent over time. It should be repeatable and provide consistent results when accessed or queried at different times. Inconsistent or unreliable data can undermine trust in the analytics process and skew results.
The Impact of Poor Data Quality
While high-quality data offers numerous benefits, poor data quality can have significant negative consequences. Here are some of the key impacts of poor data quality:
1. Inaccurate Reporting and Analysis
When data quality is compromised, it can lead to inaccurate reporting and analysis. This, in turn, affects decision-making and overall business performance. For example, if a company uses faulty sales data for performance reporting, it might make incorrect conclusions about its profitability, leading to flawed business strategies.
2. Inefficient Operations
Poor data quality can lead to inefficiencies in operations. For example, inaccurate inventory data may result in overstocking or understocking, causing delays or disruptions in supply chains. Inconsistent customer data can create problems with order fulfillment, leading to poor customer experiences.
3. Increased Costs
Maintaining poor-quality data often requires additional resources for cleanup, validation, and correction. These additional costs can drain resources from other areas of the business. Moreover, if poor-quality data leads to bad decisions, the financial impact could be substantial.
4. Damage to Reputation
Data quality issues can also damage a company's reputation. For example, incorrect or misleading information about products, services, or customers can result in dissatisfaction, negative reviews, or even legal actions. It’s essential for businesses to protect their reputation by ensuring the quality of their data.
How to Improve Data Quality
Improving data quality requires a systematic approach and a combination of processes and technologies. Here are some best practices for improving data quality:
1. Data Cleansing
Data cleansing involves identifying and correcting errors, inconsistencies, and inaccuracies in datasets. It is essential to regularly clean the data to ensure its accuracy and consistency.
2. Data Validation
Data validation helps ensure that the data entered into systems meets certain standards. This process can include checks for correct formats, required fields, and logical consistency.
3. Data Governance
Establishing a strong data governance framework is essential to ensure data quality. This includes defining data standards, ownership, and access control. It also involves monitoring data quality over time and making necessary improvements.
4. Use of Automation Tools
Automation tools can help streamline data management processes, including data collection, cleaning, and validation. Implementing automated workflows helps reduce human error and ensures that data is consistently maintained at a high standard.
Conclusion
In today’s data-driven world, the quality of data plays a central role in determining the effectiveness of analytics and business decisions. By maintaining high-quality data, organizations can make informed decisions, improve operational efficiency, minimize risks, and drive better business outcomes. Organizations must prioritize data quality to leverage the full potential of their data and stay competitive in their respective industries. To ensure the best practices in data management and analytics, pursuing the Best Data Analysts Training in Noida, Delhi, Pune, Bangalore, and other parts of India can provide professionals with the necessary skills to enhance data-driven decision-making and contribute to organizational success.
Subscribe to my newsletter
Read articles from Ruhi Parveen directly inside your inbox. Subscribe to the newsletter, and don't miss out.
Written by

Ruhi Parveen
Ruhi Parveen
I am a Digital Marketer and Content Marketing Specialist, I enjoy technical and non-technical writing. I enjoy learning something new.