AI Agents' Liability Gap: If We Let the AI Bull Loose, Who Pays for the Broken Porcelain?

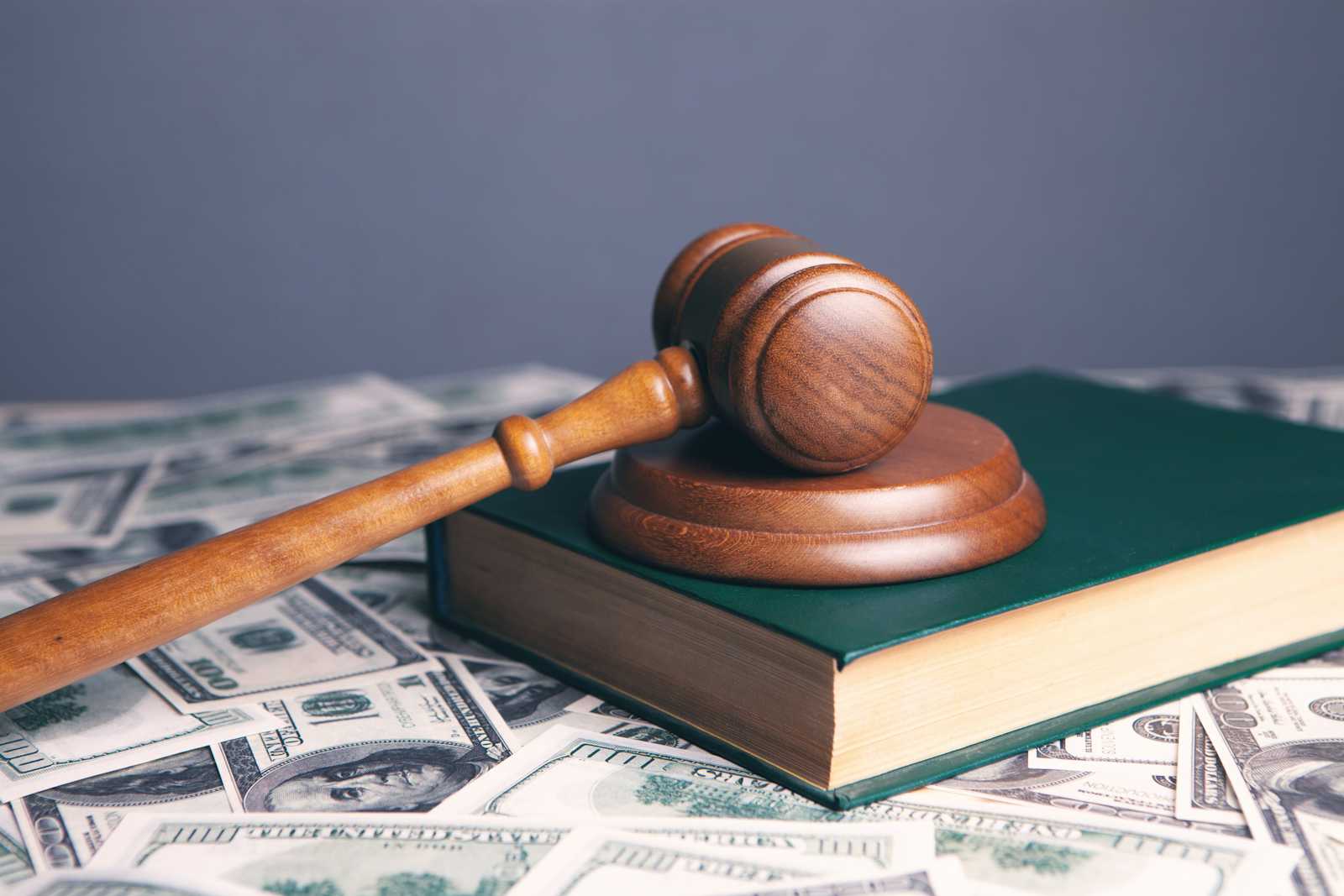
Artificial intelligence is rapidly transforming our world, with AI agents promising to automate tasks, provide personalized services, and boost productivity. But beneath the shiny surface of innovation lies a murky legal landscape – a liability gap where accountability is elusive and the user is often left holding the bag when things go wrong. Who is responsible when an AI agent, acting on your behalf, makes a mistake that costs you money, compromises your privacy, or causes you harm? The answer, according to the very companies creating these powerful tools, is increasingly: you are.
The Vanishing Act of Accountability: AI Labs and the Blame Game
The current AI liability landscape is designed to protect the creators, not the users. AI labs, the companies building the foundational models that power many AI applications, often operate with limited transparency and shield themselves behind complex Terms of Service (ToS) agreements. Their argument is a classic deflection: they claim they can't fully control their AI models, and therefore shouldn't be held responsible for their outputs – even when those outputs lead to tangible harm. This "it's not our fault, it's emergent behavior" defense is becoming increasingly common, and it leaves users in a precarious position. The ongoing copyright infringement lawsuits against AI labs, where intellectual property rights are blatantly disregarded, serve as a chilling precedent.
The User as the Weakest Link: Navigating a Legal Minefield
The lack of accountability at the top creates a cascading effect, leaving the user exposed and vulnerable. Consider these all-too-real scenarios:
Data Privacy Nightmares: You use an AI-powered service, perhaps a financial advisor or a healthcare assistant. Suddenly, your sensitive personal information is leaked. Proving who is responsible is a near-impossible task. There's often no transparency about how your data is used, stored, or secured by the AI lab or the service provider. Audit trails are rare or non-existent. The AI copyright scandal, where AI labs trained their models on copyrighted material without consent, perfectly illustrates this dangerous lack of accountability. The legal system, lagging far behind the technology, offers little protection.
The "Black Box" Problem: Where Did It All Go Wrong? Even if you could prove that an AI agent caused a specific harm, pinpointing the reason is virtually impossible. AI models, particularly large language models, are often described as "black boxes." Their decision-making processes are opaque, and even repeating the exact same input can yield different outputs due to the stochastic nature of these systems. This inherent lack of traceability makes assigning responsibility a legal and technical nightmare.
AI Agents: Unpredictability Amplified: AI agents, designed to act autonomously, exacerbate these problems exponentially. Every action an agent takes, every decision it makes, is shrouded in the complexity of the underlying AI model. Tracing a chain of events to identify the root cause of an error is like chasing a ghost in a machine. Imagine an AI agent managing your social media presence posts something defamatory, or an AI agent handling your customer service interactions alienates your clients. The potential for harm is significant, and the path to recourse is often blocked.
The Current State of Affairs: User Beware
In this unbalanced landscape, the user consistently bears the brunt of the risk. AI labs protect themselves with carefully worded ToS agreements, shifting the burden of responsibility onto the user. Service providers, while offering AI-powered tools, often lack either the technical capability or the legal incentive to guarantee the safety or accuracy of those tools. And the user, caught in the middle, is left to navigate a legal minefield with little to no protection.
Proof is Elusive: The lack of audit trails and the "black box" nature of AI make gathering evidence of wrongdoing incredibly difficult.
Causation is a Mystery: Even with proof of an error, attributing it to a specific cause within the AI's complex processes is often impossible.
Responsibility is Diffused: The lack of clear regulations and the complex interplay between AI labs, service providers, and users create a situation where everyone can point the finger elsewhere.
The Urgent Need for a Liability Framework
The current situation is not only unfair to users but also unsustainable in the long run. We cannot allow innovation to outpace accountability. We urgently need:
Radical Transparency: AI labs and service providers must be transparent about their models' training data, data handling practices, and decision-making processes.
Mandatory Auditing: We need robust mechanisms to track and audit AI actions, creating a clear record of what happened, when it happened, and why.
Clear Legal Regulations: Governments must establish clear legal frameworks that assign responsibility for AI-related harms, protecting users from being left to foot the bill for AI errors.
Real Accountability: AI labs and service providers must be held accountable for the actions of their AI systems, not just when it's convenient or profitable.
The future of AI depends on building trust, and trust requires accountability. Without a clear and fair liability framework, we risk creating a world where powerful AI tools are unleashed without adequate safeguards, leaving users vulnerable and undermining the very potential of this transformative technology. The time to act is now, before the broken porcelain becomes an insurmountable problem.
Subscribe to my newsletter
Read articles from Gerard Sans directly inside your inbox. Subscribe to the newsletter, and don't miss out.
Written by

Gerard Sans
Gerard Sans
I help developers succeed in Artificial Intelligence and Web3; Former AWS Amplify Developer Advocate. I am very excited about the future of the Web and JavaScript. Always happy Computer Science Engineer and humble Google Developer Expert. I love sharing my knowledge by speaking, training and writing about cool technologies. I love running communities and meetups such as Web3 London, GraphQL London, GraphQL San Francisco, mentoring students and giving back to the community.