Four areas of (UXR) thinking about AI / LLMs

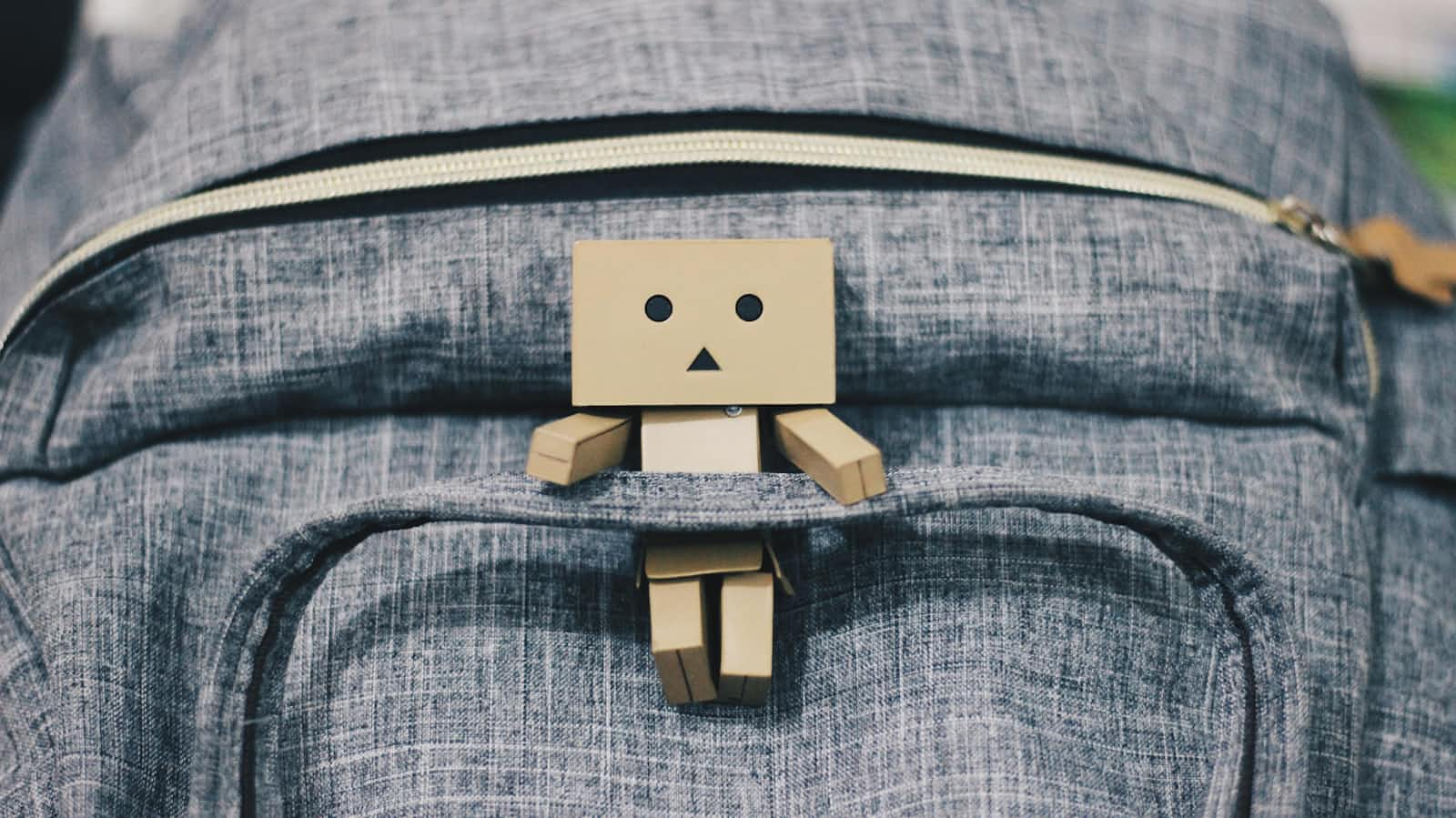
This post is my attempt to make sense (largely for myself) of various trends and responses about AI / LLMs, and thematic disagreements that I observe among UX Researchers discussing LLMs.
I structure the conversations I’ve recently had with UXRs about LLMs into four thematic areas, giving a few pro & con arguments in each. I’m writing this not to advance specific claims but instead to share a schema that has been useful to me.
Thanks to corporate interests, there is a lot of “pro AI” discussion, and the claims on that side are well known. On the other hand, social pressures act to obscure “con” arguments, and I spend slightly more time on them. Although I outline both pro & con, I’m not advocating for either “both sides” or “no one knows.” Instead, my hope here is that any reader (and especially me) will gain clarity from the considerations and can thereby have a stronger, more specific, and more coherent framework wherever they land.
I outline the following four general areas:
Utility
Aesthetics
Social Context & Reaction
Ethics & Externalities
I present this in terms of “what UXRs are thinking”. That is only because my conversations are most often with UXRs. By understanding these areas, I hope we can disentangle arguments about LLMs, such as when claims of utility are pitted against aesthetic or ethical perceptions. After laying out the framework, I recount a small illustration of how it may help us think about claims that people make.
Theme 1: Utility
The first pattern is the most obvious for UX researchers: consideration of the utility of AI in delivering use cases for a product, or similarly, in helping with UXR activities.
On the pro side there are utility claims that LLMs deliver new experiences, assists in running surveys, can analyze user responses, summarize documents, meeting, or transcripts, draft reports, write code, and so forth.
On the negative side, I see two typical patterns:
Claims that LLMs don’t make things better, or not much better, or are not worth the effort (cf. my article on R coding)
Claims that LLMs trade off short term gains for long-term harms in utility (cf. some studies in this review of AI for developer productivity, or in this study for general cognition).
A common view is that any AI application has a “game theory” payoff matrix:
Potential benefits when AI is correct or helpful
vs. Potential risks when AI is incorrect or misleading
Some activities have low risk (e.g., recommender systems, role playing chat, games) whereas others have high risk (e.g., finance, health case, security). A complete game theoretic model could additionally include the risks and benefits from ethical considerations and externalities as noted below.
A criticism of that sort of game theoretic view is that it is based on (1) an assumption of a “net profit” model where an LLM delivers some positive value, and the question is how problems trade off against that value; and (2) that assumes that such trade-offs can be made at all. As I’m arguing here, some of the trade-offs are between incommensurate areas, such that a game theory approach — although not “wrong” as such — is limited at best and strongly misleading at worst.
Overall, utility is clearly the #1 area of discussion for UXRs and product teams generally.
Side note: unlike classic utilitarian philosophy, “utility” in the AI context is often used reductively to mean “[individual] productivity.” To the extent that such claims align with classic, longer-run utilitarian considerations, they often fall into the problems of so-called “effective altruism” and related movements. That caveat is worth noting although depth discussion is out of scope here.
Theme 2: Aesthetics
The second area I see is aesthetic evaluation of AI (again, for purposes here, meaning LLMs). By this, I mean the evaluation of the beauty, tastefulness, appeal, and similar properties of AI output. This is not restricted to AI-generated art but applies to any AI output, such as the “beauty” of AI-written code, reports, texts, etc.
Although many people are using AI-generated texts and graphics, I’m not aware of positive claims that the aesthetics of those outputs are actually good (related study). Instead, AI output appears to be regarded by its users as aesthetically tolerable. They often comment that AI results are easy or “democratized”.
On a closely related positive note, there are many UXRs and others who are fascinated by AI interactions and who enjoy engaging with AI systems at length. I would not categorize that enjoyment as aesthetic but rather as more generally emotionally engaging.
On the negative side, I observe three primary areas of aesthetic complaint:
Claims that AI products are aesthetically bad, such as being ugly, badly written, “slop,” and the like. This view seems to be common among professional writers, artists, and musicians.
Many developers see AI code as needing large scale refactoring before it is “good.”
Differently, the Authors’ Guild — verified published authors, of which I’m a member — recently launched a “human authored” certification program to guarantee “authentic” works.
Worries that AI proliferation will drown out good work, rewrite aesthetic preferences, or generally lead people to miss the important aspects of creation while focusing on mere output and “productivity”. A new book, More than Words, by John Warner discusses the latter angle in depth.
A more generalized feeling that AI diminishes the appreciation of human creativity and human experiences, and that it devalues the many years and thousands of hours of effort that it takes to master a craft such as writing, drawing, painting, music, or programming.
Overall, some professional writers, developers, artists, and musicians experience AI output with a sense of revulsion. Although such an aesthetic response is emotional, I would note that it is not only emotional.
In my view, there are good reasons to treat aesthetic truth as being on par with other kinds of truths such as propositional (classically logical) truth. However, that discussion goes deep into philosophy and related areas such as cultural studies, and that is out of scope here (for more, a starting philosopher, which is not to say an easy philosopher, is Adorno).
How does this relate to the category of utility above? Answer: aesthetics and utility are separate. An interaction between product team members might go like this:
Person A: This LLM letter-writing feature will lower the bar substantially for new writers [a utility claim]
Person B: But its output is awful to read, so bland and tasteless [an aesthetic claim]
By sorting evaluations into these two categories, as well as the subcategories noted, we can better evaluate competing claims (and identify when they are not competing but are just different).
Theme 3: Social Context & Reaction
A third set of responses to AI depend on the interplay of any particular UXR’s own social situation and personality.
As we all know, AI has had widespread pressure for institutional adoption, and has also suffered from tremendous hype. At times this has been paired with claims — such as those about AGI — that are somewhere between fraudulent and incredibly ignorant (I’ve written about AGI and AI “IQ” as examples).
Each UXR responds to the social context of AI according to their own needs and personal style. While one UXR may wish to go along with demands, another may rebel against authority or hype. One may emphasize the future promise of AI, while another will see LLMs as a tool of corporate subjugation.
The impacts of AI in social contexts are not only evolving but also are more complex than any single person could observe. As in the old story about blind men and an elephant, different aspects appear with different people.
On the negative side, I’ve observed particularly hostile reactions prompted by the following:
Hype in general. Some people detest hype and react against it (the Mystery AI Hype podcast is an example)
Illogic. Some UXRs have a particular dislike of illogical claims made about AI, as they also might in other domains (nb: I personally have this tendency!)
Management demands. Some UXRs expect the worse from management (perhaps because of past trauma) and may view AI as an extension of managerial or corporate domination.
In the specific UXR context, the pro & con trends for social context show up in claims such as:
[pro] "AI is the future and UXers have to learn it”
[con] “Management doesn’t understand what they’re talking about, and we need to stop the harm to users”
[pro & con] “Excessive hype is destroying opportunities to talk about the real value of AI”
[pro] “AI is an area where I can show thought leadership” or “By using AI, I can get ahead”
Again — as we saw above for claims of utility vs. aesthetics — the set of issues regarding the social positioning of AI is logically separate from the other areas outlined here. If someone says, “We need to learn AI to get ahead,” that doesn’t imply anything in particular, positively or negatively, about whether AI is useful, aesthetic, or as I discuss next, ethical.
Theme 4: Ethics & Externalities
Preliminary Points
This is the longest section, because ethical issues are perhaps the most contentious claims about AI. Extreme positive arguments claim that AI is a force for good that will solve cancer, mitigate climate change, and eliminate human drudgery. On the extreme negative side, AI is said to be a short term tool of theft, oppression, corporatism, and militarism, and a long term existential risk to human and the world ecosystem.
Before outlining my framework, I have two overall observations. First, my impression is that ethical takes are often derived post hoc. They may be used to reinforce stances that one has already adopted for reasons of aesthetics, emotions, self interest, etc. In other words, I believe UXRs often either like AI or not, for various reasons, and then adopt “ethical” reasons to justify a stance retroactively. I’m not saying that is always the case, but often occurs (and that is perfectly OK, in my view — there is no reason why ethics should be one’s first consideration, as long as they are an eventual consideration).
Second, and closely related, I observe that ethical takes are generally unpersuasive to anyone who doesn’t already hold them. The proponents of ethical arguments appear to hope that they will be persuasive to others — whether that is “AI will cure cancer!” or that “AI is theft!” — but I have seen no good evidence that such arguments are persuasive to anyone (in the sense of changing opinions).
Now, my claim that ethical arguments are unpersuasive does not imply that ethical arguments are wrong, nor that they are useless. Ethical positions may be useful for providing a backstop and support for views held otherwise, for giving a “record” for posterity, and for deepening one’s own thinking and moral actions. Persuasion is not the only salient outcome. (Full disclosure: I think about ethics a lot because I was a philosophy graduate student prior to psychology, and I wrote a philosophy Master’s thesis in ethics. I also taught the mandatory Research Ethics class to over 1500 employees when I worked at Google.)
With those preliminaries in place, the following points outline how I see ethical issues lining up. (n.b., this area is vast and I don’t pretend that these points are complete).
Ethics in AI Training
Positive claims:
LLMs are a vastly scaled-up version of fair use, making massive information available to everyone
Training creates jobs in economically disadvantaged areas like Africa and Southeast Asia
Negative claims:
LLMs are only possible because they use stolen intellectual property (example)
The workers who train LLMs are underpaid and treated abusively, undergoing substantial trauma
Ethics in AI Applications and Other Short-Term Results
Positive claims:
LLMs deliver various features and productivity enhancement (refer to the “utility” section above)
LLMs help people such as non-native speakers or people with disabilities achieve parity with others (example)
Negative claims:
LLM features have generally low marginal value (example), and may worsen products they go into
LLM features may exist not to deliver value but to capture personal data for corporate purposes
LLMs contribute to declining skills in reading, writing, and critical thinking
The push for LLMs brings an opportunity cost of less product attention to real user needs
LLMs push all outputs towards a style of white, western, English language, educated, affluent, and heteronormative modes because that style dominates their training data
LLM data centers capture water and electricity needed by homes, businesses, and agriculture
Contrary to claims that they achieve parity, LLMs perpetuate stereotypes and biases about disabled people and other groups
Externalities and Structural Issues (current, as opposed to hypothetical)
Positive claims:
AI productivity (see “utility” above) will help companies achieve higher profits
AI is helpful for non-native speakers (by which, proponents often mean “of English”) and helps them write better, grammatically, etc.
Negative claims:
LLMs contribute to the increasing domination and centering of white, male, affluent English as a structural norm (similar to the point in “applications” above). (Examples: 1, 2, 3, 4.)
Promises (or realities) of AI productivity are contributing to job losses
LLMs devalue workers and replace creators, leading to a race to the bottom for artistic creation
LLMs increase the concentration of power among a few companies and their billionaires, achieving higher profit and more power with fewer workers and worker protections
LLM data centers pollute the planet and contribute to acceleration of climate change
LLMs differentially hurt marginal communities (non-white, low-wage, global south, etc.)
Ethics & Hypothetical Future Results
Positive claims (speculative):
AI speeds up scientific discovery, and new discoveries could mitigate many scientific problems (medical research, climate change, etc.)
AI could usher in an era of productivity such that people are freed to work less, more creatively
Negative claims(speculative):
Bugs or malicious usage of AI pose a range of threats, ranging from modest scale problems (fraud, impersonation, theft) to socially catastrophic (financial collapse) to existential (engineered viruses, killer machines, nuclear war)
AI may lead to humans being massively unemployed as jobs are replaced by AI, and largely confined to “lower skilled” labor that AI cannot replace
LLMs change expectations about truth and the veracity of both information and work products
Research-Specific Ethical Issues
Positive claims:
LLMs democratize and decrease the cost of research by providing synthetic training data and by analyzing data faster (example claim)
LLMs can discover new things, faster, by exploring research domains in new ways
Negative claims:
Research applications depend on the uncredited work of other researchers
“Democratizing research” as machine output devalues research. Normalizing research-as-production is to abandon the value of researchers and their contributions (and jobs).
Synthetic data is misleading and biased, over-representing dominant social groups (as above) and specifically downplaying and systematically under-estimating marginalized groups
Subjecting respondent and user data to LLM systems violates privacy and informed consent (1, 2)
LLM research results amplify what is obvious in a data set and/or is “centroid” in its training data, rather than discovering new things or representing actual data (related)
Using a system with ethical problems (if one believes that) is itself a research ethics violation
In some discussions, folks go farther about their concerns with LLMs. In those cases, some UXRs and managers actively question the ethics of pressure that they feel to research or use AI.
That ethical questioning takes two forms. One form is whether it is ethical to do UX research about AI that is required to say something they don’t believe — namely, that LLMs add value. I often hear researchers complaining that they are being “forced” to find reasons that AI will add product value. In those cases, they feel that the users and customers they represent are being ignored or even actively harmed. They question whether there is any point in doing research that is forced to “validate” preordained executive decisions.
Side note: research usually is not directly ordered to support a decision (although that sometimes happens). Rather, results are demanded through organizational pressure, such as: having negative results challenged; being asked to do A/B testing between options that both assume some need (AI, for example); being promoted or praised for work that extolls the desired outcome; etc. Such mechanisms bias results but preserve management ability to believe that research is “honestly sharing data”.
The second form of UXR ethical questioning is at a higher level: whether it is ethical to use LLMs at all or to work for a company that promotes them or requires one to use them. That arises if one holds some of the negative ethical views above. Similarly — but as far as I can tell, much less frequently — a UXR who is an AI proponent may have ethical concerns about NOT using AI tools. This may occur when they are in an organization that is not using LLMs because of concerns about privacy, data protection, and the like.
How the Themes Help: a Brief Example
Here’s a small example of how I personally find this framework to be helpful. Yesterday I was reading a LinkedIn post and comment that went like this (I’m not going to call out anyone, so I paraphrase):
Post: AI tools seem so potentially useful for research! What are people doing with them?
Comment: I use them all the time. Anyone not using them is a dinosaur and will be left behind.
In the past, I would have read this sort of exchange as annoyingly lacking in information, as being hype, and as name-calling (“dinosaur”) that uses belittlement instead of informed discourse (perhaps to cover up the commenter’s own anxiety).
However, using the 4 themes outlined here, I now decode it as follows:
Utility: there is a implicit claim of utility but no evidence
Aesthetics: ignored
Social context: an explicit claim that AI can help one get ahead, again without evidence
Ethics: ignored
In short, the exchange boils down to an explicit claim that AI can help someone advance, and it ignores (or somewhat denigrates) the other potential positions. I imagine this reflects anxiety more than anything else.
With the 4 elements of the framework, the argument can be deconstructed to see (a) where it is making claims and not making claims; and — just as importantly — (b) partially defused of emotion, anger, confusion, and the like, because it is clearer what is going on.
Conclusion
If you made it this far, congratulations :) As I noted at the beginning, my goal here has been to organize some areas of discourse and debate for my own thinking.
Reviewing that framework, a few themes and observations stand out:
The four themes here — utility, aesthetics, social function, and ethics — are mostly incommensurate and not directly comparable. That leads to two things:
The various sides in debates tend to miss each others’ points by responding across domains, such as answering a utility claim with an ethical rebuttal or vice versa. That convinces no one.
People simply ignore themes where the prevailing argument don’t agree with them. For example, proponents of utility dismiss may concerns about aesthetics and ethics.
After outlining the themes here, I observe that most of the discussion about AI reflects a strongly productivist orientation — in society as well as in product teams and among UXRs. LLMs are discussed in terms of how much they help people produce, how fast one can write reports or code or create images, how much data one analyze, how many articles summarized, and so forth.
The UXRs I talk with are increasingly frustrated at the situation because it leads to untenable demands on research. I believe much of that results from two things:
The incommensurability of the themes as outlined. Researchers have increasing cognitive dissonance and dissatisfaction as the thematic areas collide. For example, they may have ethical concerns competing with pressure to be productive; or pressure to support an AI feature competing with user data that suggests dissatisfaction. That leads to sharply decreased researcher morale.
They feel particular tension around the productivist orientation, that research is to be judged primarily on the basis of faster and more output (as opposed, say, to user benefit, or truth).
None of this implies a both-sides equivalence. I outline the pro and con arguments not to equate them but instead to reflect that different people hold different views. Any given UXR will — and IMO should — take a principled stance that aligns with their own perspective. To reiterate, my goal in this article was to clarify the issues, so I can better understand the positions I take.
Finally, I would note that none of these tensions are new with AI. The tensions between research demands, ethics, productivity, and so forth have always existed in product research. However, the confusions and tensions appear to be worsening rapidly. I think that reflects two things:
With its expectations, hype, and extremes, AI brings the preexisting tensions into sharper and more intense focus.
This is happening at a time of organizational and “cultural” turmoil within tech, where UXRs already feel acutely anxious. With that baseline anxiety and uncertainty, the intensified tensions of AI are more difficult to understand, process, or resolve..
What should we do about it? I don’t have specific recommendations for now. Instead, what I believe is that awareness of the situation is the first step towards finding whatever is next.
I hope these reflections and an outline of the themes will contribute to such awareness. Cheers!
Subscribe to my newsletter
Read articles from Chris Chapman directly inside your inbox. Subscribe to the newsletter, and don't miss out.
Written by

Chris Chapman
Chris Chapman
President + Executive Director, Quant UX Association. Previously: Principal UX Researcher @ Google; Amazon Lab 126; Microsoft. Author of "Quantitative User Experience Research" and "[R | Python] for Marketing Research and Analytics".