The Ethics of Data Science: What Every Professional Should Know
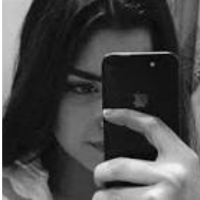

Introduction
Data science has become one of the most influential fields in today's technology-driven world. Its applications span various industries, from healthcare and finance to marketing and sports. With its ability to derive insights from vast amounts of data, data science can significantly improve decision-making and outcomes. However, as with any powerful tool, there are ethical considerations that professionals must address. The ethical responsibilities of data scientists go beyond technical skills—they also involve understanding the implications of their work on individuals, organizations, and society at large.
In this article, we will explore the key ethical considerations every data science professional should be aware of.
1. Privacy and Data Protection
One of the fundamental ethical concerns in data science is the protection of personal data. With the increasing amount of personal information being collected through various digital platforms, data scientists must ensure that this data is handled responsibly and securely.
Key Considerations:
Informed Consent: Before collecting data from individuals, it’s important to obtain their consent. This means explaining how their data will be used and ensuring that they have the option to opt out.
Data Anonymization: Data scientists should anonymize data to protect personal identities and prevent misuse. This is especially important in industries like healthcare and finance, where sensitive personal data is prevalent.
Compliance with Regulations: Data protection laws such as the General Data Protection Regulation (GDPR) in Europe and the California Consumer Privacy Act (CCPA) impose strict guidelines on how personal data should be handled. Professionals must be aware of these regulations and ensure their projects comply.
2. Bias and Fairness
Another critical issue in data science is the potential for bias in data analysis and algorithmic decision-making. Data scientists need to ensure that their models and algorithms are fair and do not perpetuate or exacerbate existing biases.
Key Considerations:
Identifying Bias: Data can contain inherent biases, whether they arise from biased human behavior, flawed data collection methods, or skewed sampling. It's essential to identify and mitigate these biases before building models.
Fair Algorithms: Algorithms should be tested to ensure that they do not unfairly favor or discriminate against any group. For example, a hiring algorithm should not unintentionally favor certain genders or ethnicities over others.
Inclusive Data: Using diverse, representative datasets can help reduce bias. Data scientists should work to ensure that the data used reflects all relevant groups to avoid unfair outcomes.
3. Transparency and Accountability
Transparency in how data is collected, analyzed, and used is crucial for maintaining trust with stakeholders. Data scientists must ensure that their methodologies, models, and decision-making processes are clear and understandable.
Key Considerations:
Explainability: Data science models, particularly machine learning models, can be complex. It's essential for data scientists to make the results of their analysis and models understandable, even for non-technical stakeholders. This includes being able to explain why certain decisions or predictions were made.
Accountability: When a model makes a mistake or results in negative consequences, data scientists should take responsibility. Whether the mistake is due to biased data, incorrect assumptions, or a flaw in the model, professionals should be prepared to correct the error and learn from it.
Documentation: Keeping detailed records of the methodologies, assumptions, and data used in a project is essential for maintaining transparency and enabling accountability.
4. The Impact on Society
The work of data scientists can have far-reaching consequences for society. From predictive policing to credit scoring, data science is increasingly influencing decisions that impact people's lives. Therefore, ethical data science requires careful consideration of how these decisions affect individuals and communities.
Key Considerations:
Long-Term Consequences: Professionals must think beyond immediate benefits and consider the long-term effects of their work. For example, algorithms that predict criminal behavior or assess creditworthiness could perpetuate social inequalities if not designed with care.
Social Responsibility: Data scientists should be aware of the societal implications of their work. For instance, in the case of algorithms used in hiring, there is a risk that automated systems could reinforce existing social biases. Understanding these broader implications ensures that technology serves the public good.
Avoiding Harm: One of the core principles in ethics is to "do no harm." Data scientists must assess whether their work could cause harm to individuals, groups, or communities, and if so, work to minimize that risk.
5. Integrity and Professionalism
Integrity in data science involves conducting work that is not only technically sound but also ethically responsible. Professionals should strive to uphold high standards of honesty and fairness in their practices.
Key Considerations:
Honest Reporting: Data scientists should be transparent about their findings, including limitations or uncertainties. It’s essential to report results in a way that does not mislead stakeholders, even if the outcomes are not as expected.
Conflicts of Interest: Professionals must avoid conflicts of interest that may influence their objectivity. For example, if a data scientist is working on a project for a company that stands to benefit from certain results, this could bias their analysis.
Professional Development: Ethics in data science is an evolving field, and professionals must commit to ongoing learning. Keeping up with best practices, ethical guidelines, and new technologies is essential for responsible practice.
6. Collaboration and Ethical Leadership
Data science is a collaborative field, often involving teams of professionals from various disciplines. As such, ethical leadership is key to fostering an environment of responsibility and trust.
Key Considerations:
Fostering Ethical Culture: Data scientists should work to cultivate an ethical culture within their teams and organizations. This includes advocating for ethical standards, providing mentorship, and encouraging open discussions about ethical dilemmas.
Cross-Disciplinary Collaboration: Ethical issues often require input from professionals in other fields, such as law, social sciences, and philosophy. By working across disciplines, data scientists can gain a more comprehensive understanding of the ethical implications of their work.
Conclusion
The ethics of data science are not just about following rules they are about understanding the profound impact that data science can have on individuals, organizations, and society as a whole. Data scientists must balance their technical expertise with ethical considerations, ensuring that their work serves the public good and respects individual rights. By prioritizing privacy, fairness, transparency, and integrity, professionals in data science—and even those pursuing related fields like an Automation Testing Training course in Delhi, Noida, Pune, Mumbai and other cities in India can help guide their domains toward a more responsible future. If you’re interested in contributing to this evolving landscape, staying informed and proactive about ethical concerns is essential for building trust and driving innovation.
Subscribe to my newsletter
Read articles from shakyapreeti directly inside your inbox. Subscribe to the newsletter, and don't miss out.
Written by
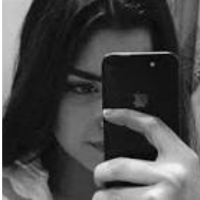
shakyapreeti
shakyapreeti
ABOUT I am Preeti, working as a Digital Marketer and Content Marketing.