AI-Powered Pharmacogenomics: Precision Medicine for Genetic Variability

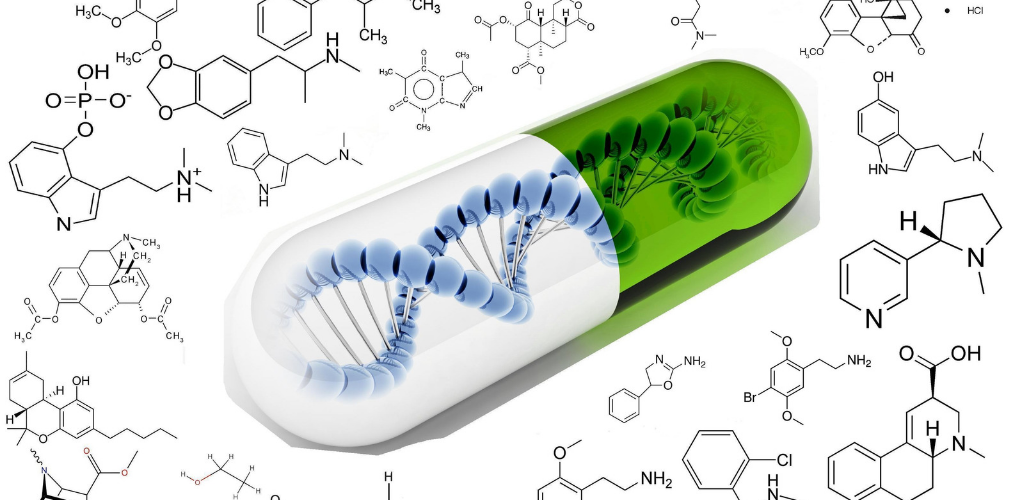
Introduction
Pharmacogenomics is the study of how genetic differences influence an individual's response to drugs. This field is crucial in advancing precision medicine by tailoring treatment strategies based on a patient’s genetic profile. Artificial intelligence (AI) is revolutionizing pharmacogenomics by analyzing vast genomic datasets, identifying genetic markers, and predicting drug responses with improved accuracy. By leveraging AI, healthcare providers can personalize treatments, reduce adverse effects, and enhance therapeutic efficacy.
The Role of Pharmacogenomics in Precision Medicine
Genetic variability significantly affects drug metabolism, efficacy, and toxicity. For example, polymorphisms in genes such as CYP2D6, CYP3A4, and TPMT can alter enzyme activity, impacting how drugs are processed in the body. Pharmacogenomics aims to identify these genetic markers to predict how individuals will respond to medications.
AI models help decode complex genetic patterns by processing large datasets derived from genomic sequencing, electronic health records (EHRs), and clinical trials. This approach enhances decision-making, ensuring patients receive the most effective treatments with minimal risk.
AI Techniques in Pharmacogenomics
AI techniques play a pivotal role in analyzing genetic data and predicting drug responses. Key methods include:
1. Machine Learning (ML) Algorithms
Machine learning techniques, such as decision trees, support vector machines (SVM), and random forests, effectively classify genetic markers and their influence on drug response. These models excel in analyzing structured data and identifying complex genetic interactions.
2. Deep Learning Models
Deep learning models, particularly convolutional neural networks (CNNs) and recurrent neural networks (RNNs), excel at analyzing unstructured genomic data. CNNs are often applied in genomics to detect mutations, while RNNs are useful for modeling time-series data related to drug effects and disease progression.
3. Natural Language Processing (NLP)
NLP algorithms extract valuable insights from biomedical literature, clinical notes, and genomic research papers. By automating data extraction, NLP accelerates the identification of genetic biomarkers relevant to drug response.
4. Reinforcement Learning (RL)
RL algorithms dynamically adjust treatment protocols based on patient-specific responses. These models optimize drug regimens, dosage adjustments, and combination therapies to enhance treatment outcomes.
Applications of AI in Pharmacogenomics
1. Predicting Drug Response
AI models analyze genomic profiles to predict how patients will respond to specific medications. For example, in cardiovascular medicine, polymorphisms in the CYP2C19 gene influence the effectiveness of antiplatelet drugs like clopidogrel. AI algorithms can predict whether patients require alternative treatments.
2. Cancer Treatment Personalization
In oncology, AI models identify genetic mutations that influence tumor growth and drug resistance. This information helps oncologists tailor chemotherapy regimens, immunotherapies, and targeted therapies for individual patients.
3. Identifying Biomarkers
AI accelerates the discovery of genetic biomarkers by identifying subtle genomic variations linked to drug response. These biomarkers guide precision medicine strategies for conditions such as epilepsy, asthma, and autoimmune disorders.
4. Adverse Drug Reaction Prediction
AI systems predict the likelihood of adverse drug reactions by analyzing genetic markers, metabolic pathways, and clinical histories. This proactive approach minimizes harmful side effects and ensures safer treatments.
5. Optimizing Drug Development
AI-driven pharmacogenomics accelerates drug discovery by predicting candidate compounds' efficacy based on genetic profiles. This reduces the time and cost of clinical trials while improving drug success rates.
Benefits of AI-Powered Pharmacogenomics
AI offers numerous benefits in advancing pharmacogenomics:
Enhanced Precision: AI models analyze complex genetic interactions, ensuring more accurate drug response predictions.
Faster Insights: AI accelerates biomarker discovery and treatment personalization by rapidly processing vast genomic datasets.
Reduced Adverse Effects: By predicting potential drug toxicity, AI minimizes harmful reactions and enhances patient safety.
Improved Treatment Outcomes: Tailoring treatments based on genetic variability optimizes drug efficacy and therapeutic success.
Challenges in AI-Driven Pharmacogenomics
Despite its potential, AI implementation in pharmacogenomics faces several challenges:
Data Complexity: Genomic data is vast, heterogeneous, and complex. Ensuring data quality, standardization, and integration remains a significant challenge.
Model Interpretability: AI models often function as "black boxes," making it difficult for clinicians to understand the rationale behind their predictions.
Ethical and Privacy Concerns: Genomic data is highly sensitive, necessitating stringent security measures to protect patient privacy.
Regulatory Barriers: Ensuring AI models comply with healthcare regulations and gain clinical approval requires extensive validation and evaluation.
Future Directions
The future of AI-driven pharmacogenomics lies in integrating multi-omics data, including genomics, proteomics, and metabolomics, to provide comprehensive insights into drug responses. Explainable AI (XAI) techniques are also gaining traction, offering transparent models that allow clinicians to interpret predictions more easily.
Additionally, advancements in federated learning enable AI models to train on decentralized genomic datasets, preserving patient privacy while improving model performance. These innovations hold promise for expanding precision medicine in diverse populations.
Conclusion
AI-powered pharmacogenomics is revolutionizing precision medicine by providing data-driven insights into genetic variability and drug response. By leveraging machine learning, deep learning, and NLP techniques, AI enables healthcare providers to deliver personalized treatment plans that enhance efficacy and minimize adverse effects. As AI continues to advance, its integration into pharmacogenomics will play a pivotal role in achieving safer, more effective, and individualized healthcare solutions..
Subscribe to my newsletter
Read articles from Zakera Yasmeen directly inside your inbox. Subscribe to the newsletter, and don't miss out.
Written by
