Day - 6 | Innovation with Google Cloud AI

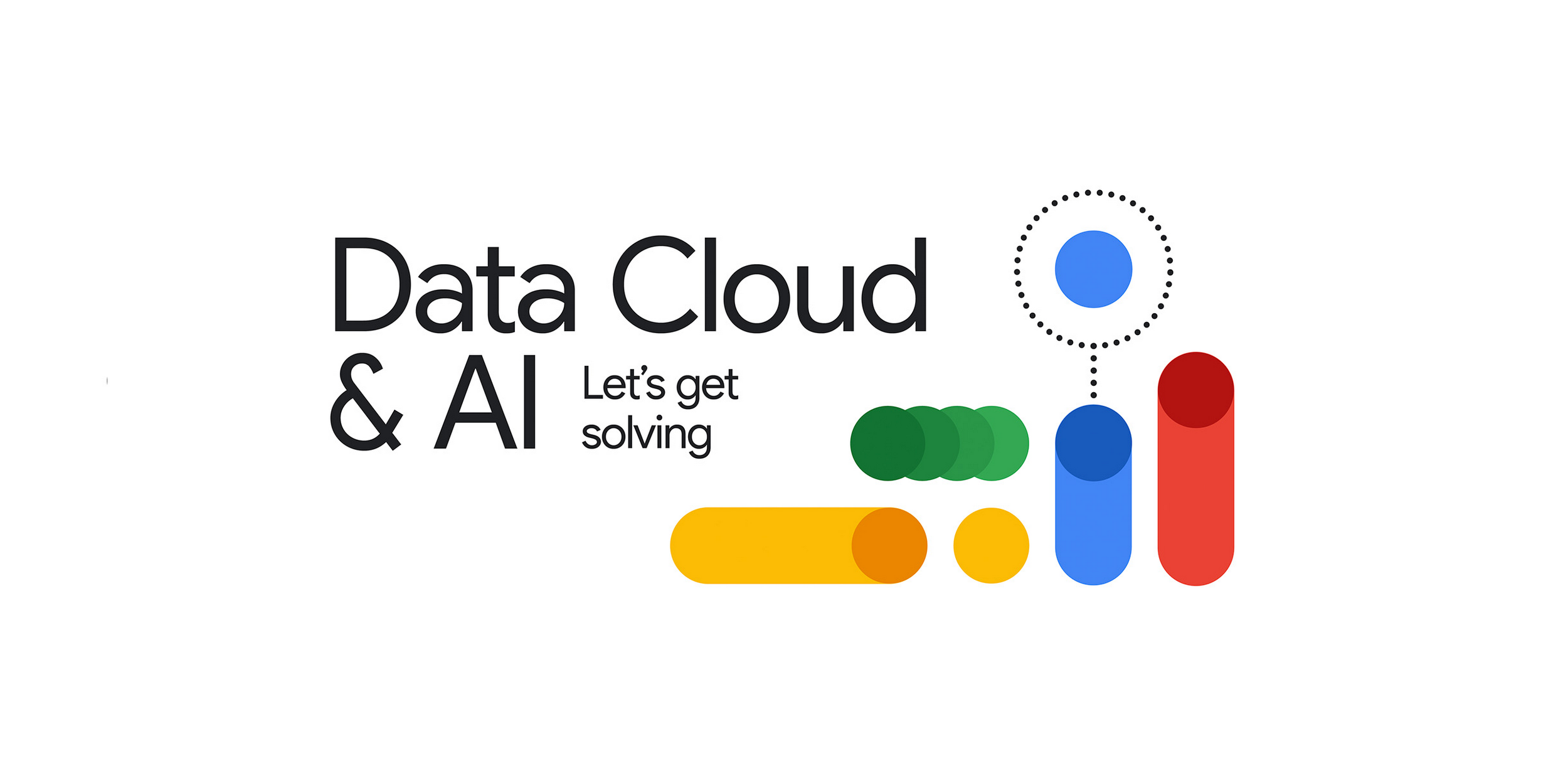
Artificial Intelligence (AI) and Machine Learning (ML) are transforming businesses across industries. Google Cloud offers a powerful suite of AI/ML tools, making it easier than ever to leverage these technologies. In this blog post, we'll break down the essentials of Google Cloud AI, from understanding the core concepts to building responsible and explainable AI solutions.
Google's AI in Everyday Products
Think about how often you use AI in your daily life. Google powers nine products with over a billion users each: Android, Chrome, Gmail, Drive, Maps, Search, Play Store, YouTube, and Photos. Features like image search, YouTube recommendations, smart compose in Gmail, and traffic predictions in Maps are all driven by AI and ML.
AI vs. ML: What's the Difference?
Artificial Intelligence (AI): AI is the broader field that aims to create machines that can mimic human intelligence. It's about building systems that can think, learn, and solve problems.
Machine Learning (ML): ML is a subset of AI. It's about training machines to learn from data without explicit programming. ML models analyze data, identify patterns, and make predictions or decisions.
Generative AI: This type of AI creates new content, like text, images, and audio. Google uses generative AI in Workspace to automate tasks, and provides APIs for developers to build custom applications.
AI/ML vs. Data Analytics and Business Intelligence
Data analytics and BI focus on analyzing past data to find trends and metrics.
AI/ML goes further by using that data to predict future outcomes and make informed decisions, enabling proactive strategies.
Why Machine Learning Needs High-Quality Data
ML models learn from data, so the quality of that data is crucial. Here's what makes data "high-quality":
Completeness: All necessary data is present.
Uniqueness: No duplicate data entries.
Timeliness: Data is up-to-date.
Validity: Data conforms to defined standards (e.g., date formats).
Accuracy: Data is correct (e.g., correct labels for images).
Consistency: Data is uniform and doesn't contradict itself.
If the data is flawed, the ML model's predictions will be flawed too.
The Importance of Responsible and Explainable AI
Google Cloud emphasizes responsible AI development. This includes:
Social Benefit: AI should have a positive impact on society.
Fairness: AI should avoid creating or reinforcing biases.
Safety: AI systems should be built and tested for safety.
Accountability: AI systems should be accountable to people.
Privacy: AI should incorporate privacy principles.
Scientific Excellence: AI development should adhere to high scientific standards.
Explainable AI
Explainable AI tools help you understand how your ML models make predictions, fostering transparency and trust.
These tools are integrated into Google Cloud products, ensuring responsible AI development.
How Google Cloud AI Can Transform Your Business
Google Cloud offers pre-trained APIs, AutoML, and custom AI/ML products to help you:
Automate tasks.
Gain insights from data.
Improve customer experiences.
Build innovative applications.
Conclusion
Google Cloud AI provides the tools and resources you need to harness the power of AI and ML. By focusing on high-quality data and responsible AI practices, you can build innovative solutions that benefit your business and society.
Subscribe to my newsletter
Read articles from Aditya Khadanga directly inside your inbox. Subscribe to the newsletter, and don't miss out.
Written by

Aditya Khadanga
Aditya Khadanga
A DevOps practitioner dedicated to sharing practical knowledge. Expect in-depth tutorials and clear explanations of DevOps concepts, from fundamentals to advanced techniques. Join me on this journey of continuous learning and improvement!