The Future of AI in Financial Risk Management: Integrating Neural Networks, Reinforcement Learning, and Generative AI for Proactive Fraud Detection

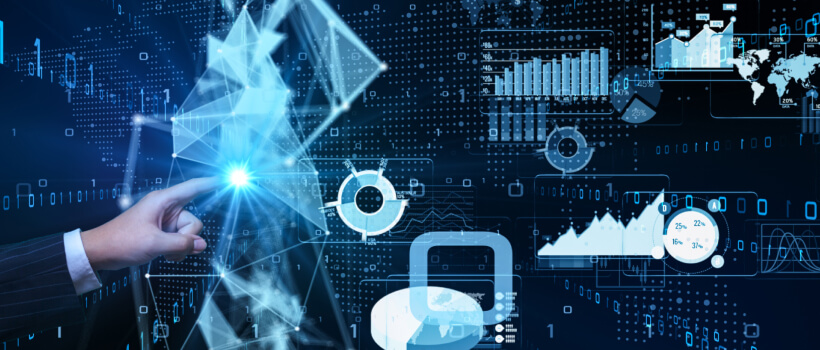
Abstract
The financial sector faces increasing risks due to sophisticated fraud techniques, data breaches, and regulatory challenges. Artificial intelligence (AI) solutions leveraging neural networks, reinforcement learning, and generative models are transforming financial risk management by enhancing fraud detection and prevention capabilities. This paper explores the role of these AI technologies in achieving proactive fraud detection and strengthening financial security frameworks.
Neural Network for Fraud Risk Prediction
Introduction
Financial institutions constantly grapple with evolving threats, from identity theft to transaction manipulation. Traditional risk management approaches struggle to adapt quickly to such changes. AI-driven methods, particularly neural networks, reinforcement learning, and generative AI, offer a more dynamic and responsive approach to fraud detection. By employing these advanced techniques, financial organizations can automate threat detection, minimize false positives, and improve decision-making accuracy.
Neural Networks in Fraud Detection
Neural networks excel at identifying complex patterns and learning from vast data sets, making them highly effective in fraud detection models.
Feedforward Neural Networks (FNNs): These networks are ideal for identifying risk patterns in structured financial data, such as credit scores, transaction amounts, and customer profiles.
Convolutional Neural Networks (CNNs): Used for document verification, CNNs excel at image recognition tasks like detecting forged signatures or manipulated identity documents.
Recurrent Neural Networks (RNNs): RNNs are valuable in analyzing sequential transaction data, tracking unusual activity patterns over time.
By integrating these neural models into financial platforms, institutions can identify anomalies and suspicious activities in real-time, enhancing fraud prevention mechanisms.
Reinforcement Learning for Adaptive Risk Management
Reinforcement learning (RL) models are designed to learn optimal decision strategies by interacting with dynamic environments. In financial risk management, RL agents can:
Predict fraud risk by learning from past fraudulent transactions.
Adjust transaction limits dynamically to minimize risk.
Optimize security policies for evolving threats.
For instance, in real-time payment systems, RL can track user behavior patterns and adaptively flag transactions deviating from established norms. By leveraging reinforcement learning's ability to self-learn and improve, financial systems can maintain proactive risk management frameworks.
Generative AI for Fraud Simulation and Data Augmentation
Generative AI models, including Generative Adversarial Networks (GANs) and Variational Autoencoders (VAEs), are valuable for simulating fraudulent behaviors and enhancing training datasets.
Synthetic Fraud Data Generation: GANs can create realistic synthetic transaction data that mimics genuine patterns. This enables financial institutions to train fraud detection models without exposing sensitive customer information.
Scenario Simulation: Generative models can simulate potential attack strategies, empowering risk management teams to develop proactive security protocols.
Anomaly Detection: By training on both real and synthetic data, models can effectively differentiate between legitimate and fraudulent activities.
Reinforcement Learning for Dynamic Fraud Detection
Integration Strategies for AI Technologies
For optimal results, financial institutions must integrate neural networks, reinforcement learning, and generative AI in a cohesive framework:
Data Fusion: Combining structured financial data with unstructured sources like social media, email logs, and transaction metadata enhances fraud detection.
Automated Decision Systems: Reinforcement learning agents can trigger automated responses (e.g., transaction freezes or KYC revalidation) when high-risk behavior is detected.
Continuous Learning Pipelines: Adaptive models should be continuously trained with evolving fraud data to improve accuracy over time.
Risk Score Aggregation for Decision-Making
Challenges and Risks
Despite its potential, integrating AI into financial risk management presents certain challenges:
Data Privacy and Security: Handling customer data requires compliance with strict data protection regulations.
Model Interpretability: Neural networks and generative AI models often operate as "black boxes," raising concerns about explainability.
Adversarial Attacks: Malicious actors can manipulate AI systems by introducing deceptive data patterns, undermining model accuracy.
Future Directions
The future of AI in financial risk management lies in:
Advancing explainable AI (XAI) solutions to improve model transparency.
Leveraging blockchain technology for secure data exchange in AI-driven financial systems.
Developing hybrid AI models that combine rule-based systems with adaptive neural networks for improved reliability.
Conclusion
The integration of neural networks, reinforcement learning, and generative AI offers powerful solutions for proactive fraud detection in financial systems. By combining predictive accuracy, adaptive learning, and synthetic data generation, financial institutions can enhance risk management capabilities, reduce losses, and improve customer trust. Future advancements in explainable AI and secure data-sharing frameworks will be essential for fully realizing the potential of AI in global financial risk management.
Subscribe to my newsletter
Read articles from Srinivasarao Paleti directly inside your inbox. Subscribe to the newsletter, and don't miss out.
Written by
