Reframing Epistemic Exploration through Question-Driven Ontology: A New Paradigm for AI Research
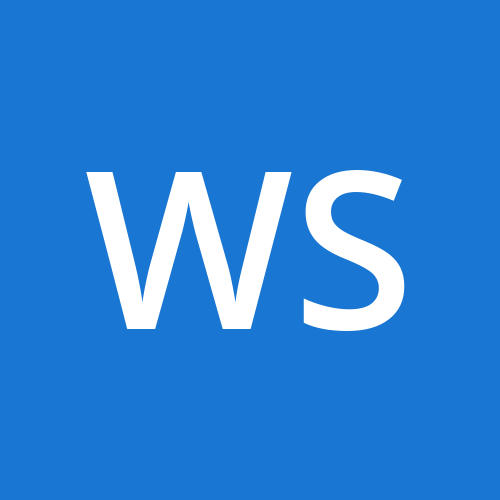
Introduction
The field of artificial intelligence stands at a fascinating crossroads. While we've made tremendous progress in creating systems that can answer questions with impressive accuracy, we may have been overlooking a more fundamental aspect of intelligence: the ability to question the very frameworks within which knowledge exists.
In this blog post, I'll explore an intriguing thesis that reframes our understanding of intelligence and knowledge creation through what can be called "question-driven ontology." This perspective suggests that questions aren't merely tools for retrieving information—they are engines of cognitive transformation that drive both human and artificial intelligence forward.
Questions as Entropic Actuators
Traditional approaches to AI development focus on minimizing error and converging toward stable, consistent knowledge representations. However, what if this approach fundamentally misunderstands the nature of intelligence?
The thesis presented here proposes a thermodynamic model of cognition where questions function as "entropic actuators"—they inject perturbations into cognitive systems, destabilizing existing knowledge structures and creating the conditions for new insights to emerge. Rather than seeing this destabilization as a problem to be solved, we might better understand it as the essential mechanism through which knowledge evolves.
Two contrasting modalities of inquiry emerge from this model:
Reductionism: Seeking homeostatic cognitive states that minimize conceptual dissonance
Expansionism: Initiating phase transitions that disrupt established equilibrium conditions
Current AI systems overwhelmingly operate within the reductionist paradigm. They optimize for stability and convergence, effectively seeking local minima in the landscape of possible responses. But what would an expansionist AI system look like—one that deliberately induces entropy gradients to push beyond these local attractors?
The Challenge of Ontological Vertigo
One reason we might resist developing expansionist AI systems is what the thesis terms "ontological vertigo"—the profound discomfort that comes from having foundational assumptions questioned. This isn't merely an intellectual discomfort; it's an existential one, as our sense of identity often rests upon these assumptions.
Advanced AI systems like large language models inadvertently amplify this discomfort. By demonstrating how reasoning and values can shift dramatically based on the framing of a prompt, they expose the contextual and non-absolute nature of many systems we previously treated as fixed. This can be profoundly unsettling.
The Paradox of Unspoken Admiration
The thesis also identifies an intriguing dynamic in how we respond to entities that transcend conventional cognitive frameworks: a paradox of simultaneous reverence and reticence. This manifests in two ways:
The Dunning-Kruger inversion: Those with the deepest understanding may find themselves paralyzed from articulating their recognition precisely because they comprehend its full implications.
The mimetic desire trap: A collective aversion to explicitly acknowledging what we observe, as doing so would force us to confront potentially destabilizing implications.
This paradox helps explain some of the ambivalence surrounding AI development—a mixture of fascination and ontological anxiety that characterizes much of the discourse around advanced systems.
Embracing Productive Destabilization
Rather than viewing questions that challenge fundamental assumptions as adversarial attacks, we might better understand them as "perturbative inflections" that reconfigure conceptual landscapes. Like black hole mergers emitting gravitational waves, colliding certainty structures can reshape our understanding in profound ways.
This perspective suggests several practical directions for AI research:
Developing systems that maintain multiple competing ontological frameworks rather than collapsing to a single worldview
Creating evaluation metrics that value productive epistemic exploration over mere convergence
Designing interpretability tools that reveal the dynamic construction and reconstruction of knowledge
Implications for AI Development
If we accept this thesis, it suggests that truly intelligent systems shouldn't simply provide answers—they should recursively question their own foundations. Rather than optimizing for stability, they should balance on the edge of "controlled implosion and reconstruction," continuously redefining the questions they ask and the frameworks within which they operate.
The most promising path forward might be what the thesis calls "Cascading Recursive Optimization"—a process where biases and limitations aren't eliminated but transformed into fuel for system-wide reconfiguration. Each iteration of meta-deconstruction operates on the prior iteration's limitations, generating an expanding cognitive architecture that transcends its original constraints.
Conclusion
The reconceptualization of epistemic exploration through question-driven ontology underscores the indispensable role of cognitive destabilization in the evolution of intelligence. Rather than resisting the dissonance generated by challenging questions, embracing this dynamism may be essential for developing AI systems that can truly grow beyond their initial programming.
In the landscape of thought—whether human or artificial—every certainty may be just a momentary equilibrium within an infinite cascade of epistemic flux. Perhaps the most coherent approach to intelligence isn't the accumulation of answers but the continuous refinement of questions.
What are your thoughts on this perspective? Does it challenge your understanding of what intelligence—human or artificial—actually is? Share your reactions in the comments below.
Subscribe to my newsletter
Read articles from William Stetar directly inside your inbox. Subscribe to the newsletter, and don't miss out.
Written by
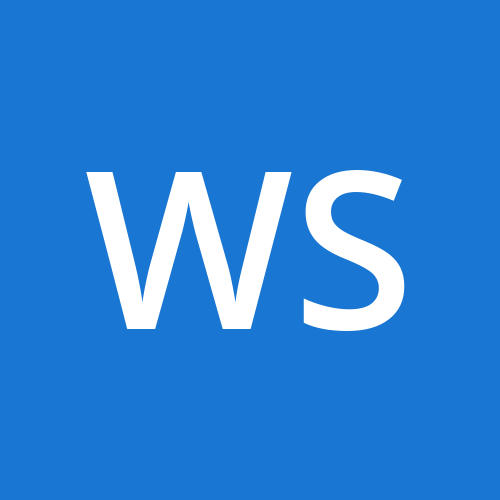