PySpark: Removing Duplicates in Large Datasets
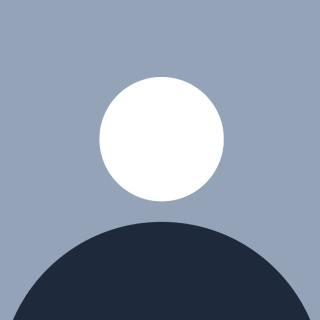
Scenario
Your dataset contains duplicate customer records. You need to remove duplicates based on the latest timestamp.
Solution: Use dropDuplicates()
& Window Functions
Step 1: Sample Data
from pyspark.sql.functions import col
from pyspark.sql import SparkSession
spark = SparkSession.builder.appName("RemoveDuplicates").getOrCreate()
data = [
(1, "Alice", "2024-03-10"),
(1, "Alice", "2024-03-15"), # Duplicate customer_id (newer date)
(2, "Bob", "2024-03-08"),
(3, "Charlie", "2024-03-12"),
(3, "Charlie", "2024-03-14") # Duplicate customer_id (newer date)
]
columns = ["customer_id", "name", "updated_at"]
df = spark.createDataFrame(data, columns)
df.display()
Step 2: Remove Duplicates Using Window Function
from pyspark.sql.window import Window
from pyspark.sql.functions import row_number
# Define a window partitioned by customer_id, ordered by updated_at DESC
window_spec = Window.partitionBy("customer_id").orderBy(col("updated_at").desc())
# Add row number column
df = df.withColumn("row_number", row_number().over(window_spec))
# Keep only latest record per customer
df_filtered = df.filter(col("row_number") == 1).drop("row_number")
df_filtered.display()
Window functions let us find the latest record per customer.
We keep only row_number = 1 (latest record).
dropDuplicates()
# Drop Duplicates on Entire DataFrame
df_unique = df.dropDuplicates()
Without column names,
dropDuplicates()
removes rows where all column values match exactly.If even one column differs, it wonβt be removed.
dropDuplicates(columnName)
# Drop duplicates based on customer_id
df_unique = df.dropDuplicates(["customer_id"])
dropDuplicates(["customer_id"])
keeps the first occurrence but doesn't always keep the latest record.
Subscribe to my newsletter
Read articles from Venkatesh Marella directly inside your inbox. Subscribe to the newsletter, and don't miss out.
Written by
Venkatesh Marella
Venkatesh Marella
π About Me: I am a Data Solution Engineer with 12+ years of experience in Big Data, Cloud (Azure & AWS), and AI-driven data solutions. Passionate about building scalable ETL pipelines, optimizing Spark jobs, and leveraging AI for data automation. I have worked across industries like finance, gaming, automotive, and healthcare, helping businesses make data-driven decisions efficiently. π What I Write About: PySpark & Big Data Processing ποΈ Optimizing ETL & Data Pipelines β‘ Cloud Engineering (Azure & AWS) βοΈ Streaming & Real-Time Data (Kafka, Spark Streaming) π‘ AI & Machine Learning in Data Engineering π€ π Why Follow Me? I share real-world data engineering challenges and hands-on solutions to help fellow engineers overcome bottlenecks and optimize data workflows. Letβs build robust, scalable, and cost-efficient data systems together! Follow for updates on cutting-edge data engineering topics!