Part 1 - From Digital Transformation to AI Evolution: A New Chapter in BFSI
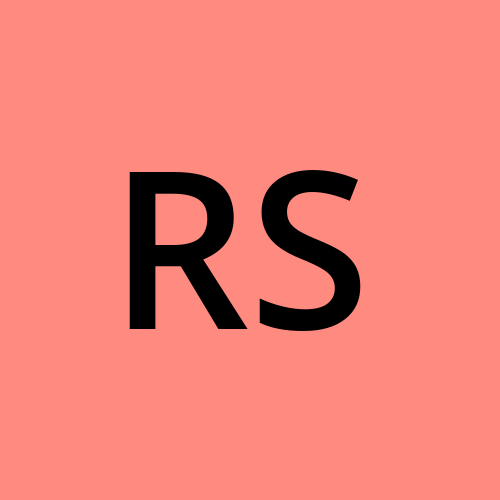
Driving the Next Wave of Digital Evolution in BFSI industry
We’ve all heard about digital transformation, right? In our previous discussion, we explored the topic of digital transformation with a focus on Cloud Adoption and advancements in Data Engineering. From cloud adoption to data lakes and containerization, we’ve witnessed technology reshaped the industry. However, for forward-looking institutions, particularly in the financial sector, it’s no longer just about transformation — it’s about evolution.
So, what’s driving this shift? The answer is Artificial Intelligence (AI).
AI is the key enabler, transforming established digital systems into powerful engines of agility, innovation, and customer-centricity.
Today, institutions in the BFSI (Banking, Financial Services, and Insurance) sector are leveraging AI to transcend traditional boundaries, ushering in an era of hyper-personalization, real-time decision-making, intelligent automation and seamless customer journeys.
But how exactly is AI shaping this evolution? Let’s explore the transformative role of AI in finance and insurance, delves into its impact on delivery and engineering processes, and highlights how it addresses the growing expectations of personalised, secure, and context-aware services. Also a quick summary on how institutions can map the next step of digital transformation to their business goals and what all they can consider to calculate the ROI for this transformation
AI — Logical Successor to Cloud Transformation
Over the last decade, cloud technologies have revolutionized the way businesses store data and scale infrastructure. However, the cloud alone cannot unlock the full potential of the vast amounts of data that financial institutions collect. This is where AI comes in. While the cloud is instrumental in enabling scalability and flexibility, AI turns all of that data into actionable insights.
For banks, this means being able to deliver personalized services based on real-time data analytics. For insurers, AI can dynamically adjust premiums based on customer data and risk profiles. AI is not just another technology to integrate into existing systems; it is a fundamental catalyst for a new wave of financial services that is data-driven, predictive, and intelligent.
AI’s Strategic Role in Banking, Finance, Insurance and Payment Industry
1. Hyper-Personalization, Customer Acquisition and Intelligent Automation
The first major area of transformation is hyper-personalization. For banks, AI can provide deep insights into a customer’s behaviour, transaction history, and preferences. Based on these insights, institutions can offer tailored financial advice, personalized loans, and pre-approved credit lines, all of which improve customer engagement.
In the insurance space, AI can not only assess customer behaviour but also adjust premiums in real-time based on risk profiles, and even identify cross-selling opportunities based on customer history. This results in a more personalized experience, driving customer loyalty and satisfaction.
But AI isn’t just about personalization — it’s also about intelligent automation. AI-powered systems can autonomously process insurance claims, recommend investment opportunities, and even manage low-risk loans without the need for human intervention. This frees up valuable human resources for tasks that require more judgment and oversight.
Hyper-Personalization
Banking Services: AI analyses customers’ financial behaviours, such as spending habits and transaction patterns, to offer tailored credit card options, personalized investment recommendations, and budget management insights. It proactively identifies customer needs for pre-approved loans or financial planning based on real-time data.
Insurance Offerings: Evaluates individual risk profiles, dynamically calculating premiums and recommending personalized add-ons like health trackers or wellness programs. It uncovers cross-selling or upselling opportunities by analysing historical claims data and lifestyle changes.
Intelligent Automation
Autonomous Financial Advisors: AI-powered systems continuously monitor market trends to adjust strategies for optimal returns on investments. AI also enable real-time decision-making, offering instant advice on portfolio rebalancing or retirement planning.
Autonomous Insurance Underwriting: AI models autonomously evaluate low-risk claims, accelerating resolution times. By leveraging predictive analytics, they anticipate policyholder risks, minimizing the need for manual interventions.
Customer Relationship Management
AI enhances customer relationship management by enabling hyper-relevant, data-driven engagement strategies.
360-Degree Customer View: Integrates data from multiple sources to provide a unified understanding of customer preferences and interactions.
Next Best Action (NBA): Recommends the most relevant product or service for each customer based on real-time analytics.
Conversational AI: AI chatbots deliver personalized services in regional languages, bridging gaps for underserved communities.
2. Real-Time Decision-Making and Intelligent Digital Twins
AI also plays a crucial role in real-time decision-making. In today’s fast-paced world, businesses cannot afford to be reactive. They must be proactive. AI enables banks and insurers to predict risks, forecast trends, and make decisions based on real-time data rather than relying on past information.
A great example of this is the use of digital twins — virtual models of real-world systems. In finance, digital twins are used to simulate customer behavior, adjust pricing strategies dynamically, and optimize workflows. This kind of real-time decision-making allows institutions to respond to market fluctuations and customer needs with agility and precision.
Real-Time Decision-Making and Fraud Prevention : AI-powered systems in BFSI monitor transactions in real-time, detecting anomalies and flagging potential fraud based on learned patterns. Digital twins replicate customer behaviours to offer tailored services, enhancing customer personalization in banking, insurance, and investments. Additionally, AI enables dynamic pricing by adjusting premiums or interest rates in response to market conditions, customer risk profiles, and competitor pricing, ensuring competitiveness.
Operational Efficiency and Optimization : AI-driven simulations optimize various operational aspects such as branch operations, ATM cash management, and resource allocation, improving cost efficiency and service delivery. Digital twins help streamline workflows, predict disruptions, and enhance operational efficiency across BFSI processes, including banking, lending, and insurance.
Risk Management, Strategic Planning, and Forecasting : Digital twins assess emerging risks such as climate change, market fluctuations, and customer behaviour shifts, enabling proactive risk management and compliance strategies. By modelling these factors, BFSI institutions can better anticipate challenges and implement mitigation strategies. Additionally, digital twins provide valuable insights for strategic planning and forecasting, allowing organizations to allocate resources optimally and align business strategies with market dynamics for long-term growth.
Innovation and Product Development : AI and digital twins accelerate innovation by simulating market responses and customer demand, helping BFSI institutions design and test new financial products more effectively. This enables faster time-to-market for new offerings, ensuring that products are aligned with customer needs and market trends.
3. Risk Management and Regulatory Compliance (Reg-Tech)
With ever-increasing regulatory pressures, financial institutions are leveraging AI to manage risk and ensure compliance. AI can analyse vast amounts of transaction data, detect patterns of fraudulent behaviour, and predict loan defaults before they happen. The ability to automatically detect anomalies in real-time means that banks and insurance companies can respond to risks before they become major issues.
On top of that, AI is being used to automate and streamline compliance reporting for AML (Anti-Money Laundering) and KYC (Know Your Customer) requirements. What once took hours or days can now be accomplished in real-time, reducing the likelihood of human error and ensuring that institutions remain compliant with regulatory standards.
Risk Management
Proactive Credit Risk Assessment: AI analyses behavioural and transactional data alongside macroeconomic indicators to predict potential loan defaults, providing early warnings and helping mitigate systemic risks across portfolios.
Stress Testing and Scenario Analysis: AI simulates adverse economic conditions to assess readiness and ensure compliance with frameworks like Basel III or Solvency II, identifying vulnerabilities in balance sheets across various market scenarios.
Regulatory Compliance
Automated Reporting: NLP models analyse transactional data to generate reports for AML (Anti-Money Laundering) and KYC (Know Your Customer) compliance, reducing human errors and speeding up reporting processes.
Document Review: AI extracts key insights from regulatory documents and contracts, ensuring compliance with changing legal requirements.
Continuous Monitoring: AI continuously monitors communications and transactions to identify potential compliance violations, insider trading, or unethical activities.
4. Wealth Management and Investment Optimization
AI isn’t just for established customers; it also opens doors for financial inclusion. For instance, AI-driven credit scoring models can now assess the creditworthiness of individuals who may not have a traditional credit history, extending banking services to underserved populations.
In wealth management, AI-powered Robo-Advisors are enabling investors to receive tailored financial advice without having to interact with a human advisor. These platforms are capable of analysing market trends, assessing risk, and dynamically adjusting portfolios, making wealth management services more accessible and affordable for a larger audience.
Robo-Advisors: Provide personalized financial advice based on goals, risk tolerance, and market dynamics. Automate tax-loss harvesting and rebalancing to improve returns.
Sentiment Analysis: Analyse global news, social media trends, and market sentiment to uncover risks or opportunities in real-time.
Dynamic Portfolio Management: : AI algorithms dynamically adjust asset allocations based on predictive analytics, minimizing risks while maximizing returns.
Scenario Forecasting: Models project the performance of various investment strategies under differing market conditions.
5. Lending, Credit, and Financial Inclusion
AI is transforming lending practices and promoting financial inclusivity for underserved populations.
Lending and Credit
Alternative Credit Scoring Models: Use unconventional data sources, such as utility payments and social media behaviour, to assess creditworthiness. Extend credit access to underbanked or unbanked individuals.
Loan Default Prediction: Predict borrowers at risk of default and proactively offer loan restructuring or tailored repayment plans.
Dynamic Pricing for Loans: AI optimizes interest rates in real-time, ensuring competitive pricing while managing risk.
Financial Inclusion
Microfinance Support: Assess credit potential for individuals lacking formal credit histories using behavioural data. Design tailored financial products for marginalized communities.
Buy Now, Pay Later (BNPL): Detect fraud by analysing repayment patterns and transactional behaviour. Enhance repayment rates through personalized reminders and nudges.
6. Payments, Treasury, and Capital Markets
AI is also optimizing the more transactional aspects of the BFSI sector, like payments, treasury management, and capital markets.
In payments, AI can analyze transaction patterns in real-time, making cross-border transactions faster and more cost-effective. In treasury, AI algorithms help optimize cash flow and liquidity management by predicting financial trends and ensuring that funds are deployed efficiently.
In capital markets, AI is transforming trading strategies. High-frequency trading algorithms, powered by AI, can execute trades in fractions of a second, allowing institutions to capitalize on market opportunities that would be impossible for humans to spot.
Although some of the large institution have already started creating large language models to detect risks and frauds, for others there is still far way to go to optimise cash flow, detect frauds and identify upcoming potential risks.
Payments and Transaction Banking : Intelligent routing reduces transaction costs and ensures fast cross-border payments. Real-time fraud detection prevents account takeovers and identifies suspicious merchant activity.
Treasury and Liquidity Management: Predict cash flows to optimize liquidity and hedge currency risks effectively. Customize treasury product pricing based on market trends and customer profiles.
Trading and Capital Markets: AI algorithms execute high-frequency trades at lightning speed, improving profitability. Identify market anomalies and emerging risks with real-time analytics.
7. Insurance Transformation
The insurance industry is one of the biggest beneficiaries of AI. Claims processing, which has traditionally been a labour-intensive process, is now automated with AI. Fraudulent claims can be flagged and flagged instantly, helping insurers mitigate risk.
Additionally, insurers are leveraging AI to create more personalized insurance policies, using real-time data from IoT devices to assess individual risk factors and dynamically adjust premiums. This approach helps insurers offer more relevant products to customers, improving both customer retention and profitability.
Automated Claims Processing: AI assesses claims for authenticity, flagging fraudulent activities and accelerating genuine approvals.
Dynamic Underwriting: Use non-traditional data like IoT or wearable device inputs to assess risks and design personalized policies.
Predictive Maintenance for Claims: Forecast potential claims in property and auto insurance based on customer behaviour or external factors like weather.
8. Cybersecurity and Operational Efficiency
AI secures systems against threats while improving operational processes for optimal efficiency.
Cybersecurity: Use behavioural biometrics to detect unauthorized access by identifying deviations in user behaviour. Aggregate threat intelligence to prevent cyberattacks proactively.
Operational Optimization : Predict branch foot traffic and optimize staffing schedules. Ensure ATM cash availability through predictive replenishment schedules. Identify and resolve inefficiencies in back-office processes, reducing costs and error rates.
9. Predictive Analytics and Adaptive Forecasting
AI is revolutionizing financial analytics by transitioning from retrospective evaluations to predictive and prescriptive insights, fundamentally changing how institutions approach strategy and risk management.
Sophisticated Trend Prediction:
Leveraging machine learning algorithms, financial institutions can analyze extensive historical datasets to predict trends and inform strategic decisions with high confidence levels.Scenario Simulation with Granular Accuracy:
AI facilitates advanced scenario modeling, allowing organizations to simulate various economic, market, and geopolitical conditions, thereby preparing for multiple contingencies.Enhanced Risk Assessment Frameworks:
AI goes beyond traditional statistical models by incorporating alternative data sources such as consumer behavior, macroeconomic indicators, and social trends, offering a comprehensive risk perspective.Dynamic and Real-Time Forecasting:
AI systems continuously refine financial forecasts by incorporating real-time data streams, ensuring that institutions remain responsive to market volatility and emerging opportunities.
Transformative Impact of AI — Enhanced Engineering Practices
Accelerating Delivery Through AI-Driven Automation
AI accelerates software delivery by automating time-consuming tasks, enabling faster iteration cycles, and improving the overall efficiency of the software development lifecycle.
Intelligent Testing: AI automates regression testing by intelligently identifying and selecting test cases that are most likely to detect defects, ensuring comprehensive coverage and faster validation cycles. Machine learning algorithms can predict edge cases and potential vulnerabilities based on historical data, enhancing the robustness of the testing process and reducing the chances of issues emerging in production. AI-driven testing frameworks also adapt to changes in the application, continuously refining their test strategies to address new features or modifications, reducing manual intervention.
CI/CD Optimization: AI enhances Continuous Integration and Continuous Delivery (CI/CD) pipelines by analysing deployment data and optimizing deployment strategies, ultimately reducing rollout time and minimizing system downtime. Machine learning models assess historical deployment performance, identify bottlenecks, and recommend improvements for smoother and faster delivery cycles. Automated deployment and rollback processes, powered by AI, ensure greater consistency and stability, even during high-stakes releases, by predicting potential failures and mitigating risks beforehand.
Enhancing DevSecOps with AI
In highly regulated sectors like BFSI (Banking, Financial Services, and Insurance), AI plays a pivotal role in strengthening security and compliance within DevSecOps processes, ensuring faster, more secure software delivery.
Real-Time Security Monitoring: AI systems can continuously monitor infrastructure, applications, and networks to detect and respond to potential security threats in real time. By analysing patterns in data access, network traffic, and user behaviour, AI identifies anomalies that might indicate unauthorized access or malicious activity. AI-driven security tools can detect potential zero-day vulnerabilities, ensuring proactive measures are taken before these issues affect the system. Real-time alerts help security teams respond more swiftly, reducing the time window for potential breaches.
Automated Threat Mitigation: Beyond detection, AI can autonomously take immediate corrective actions, such as isolating affected systems, blocking malicious traffic, or rolling back compromised transactions. Advanced machine learning algorithms identify attack vectors, prioritize threats based on severity, and initiate appropriate remediation strategies without human intervention, reducing response time and minimizing operational disruption.
Optimizing DORA Metrics for Performance Excellence
AI directly impacts the DORA (DevOps Research and Assessment) metrics — deployment frequency, lead time for changes, Mean Time to Recovery (MTTR), and change failure rate — by enhancing the ability to monitor, measure, and optimize these critical performance indicators.
Reducing Lead Time and Change Failure Rates: AI-powered predictive analytics can forecast potential issues during the development cycle, allowing teams to address coding bottlenecks or integration problems early. By pinpointing patterns and correlations in code changes, AI reduces the likelihood of failures and optimizes release velocity. Machine learning models can also offer recommendations on optimal release windows, based on historical success rates, thereby reducing the risk of errors in production.
Improving MTTR with Real-Time Insights: AI can provides continuous monitoring of application health, using metrics like system performance, user behaviour, and infrastructure status to offer real-time insights into potential issues. In the event of an incident, AI algorithms analyse the root cause and suggest remediation steps, allowing engineering teams to resolve issues faster. Predictive capabilities ensure that future problems are pre-emptively mitigated, reducing downtime and accelerating recovery.
Driving Engineering Maturity
AI not only optimizes engineering practices but also fosters long-term improvements in team maturity and system efficiency by providing actionable insights and predictive capabilities.
Proactive Infrastructure Monitoring: AI systems can leverage predictive models to foresee infrastructure failures, such as server overloads or database crashes, before they occur. By using historical data and real-time system behaviour, AI can recommend auto-scaling solutions or resource reallocation strategies, ensuring consistent performance during peak loads. These systems can also automate routine maintenance tasks, such as patch management and system health checks, ensuring optimal infrastructure performance without manual intervention.
Continuous Learning and Optimization: AI-driven systems can create feedback loops that continuously learn from system performance, user interactions, and operational challenges. By applying this data, AI enhances decision-making processes, recommending improvements in workflows, testing protocols, and deployment strategies. This culture of continuous learning accelerates engineering maturity by enabling teams to adapt rapidly to changing environments, optimize workflows, and continuously evolve their engineering practices to meet organizational goals.
Improving DevEx (Developer Experience) across organisation
Artificial Intelligence (AI) can change how developers work by integrating standard IDEs with Co-Pilot or Low-Code/No-Code tools, streamlining workflows, enhancing productivity, and optimizing code quality. By automating mundane tasks, providing advanced debugging and testing tools, and fostering collaboration, AI is reshaping the developer experience across the software development lifecycle. Here’s how AI can significantly improve the developer experience
Intelligent Code Assistance and Autocompletion : AI tools like GitHub Copilot, powered by OpenAI’s Codex, can autocomplete entire code blocks or functions based on context, allowing developers to focus more on solving business problems than writing boilerplate code.
Automated Code Refactoring : AI can suggest or automatically perform code refactoring, helping to improve code readability, efficiency, and maintainability without changing its functionality.
Improved Code Reviews and Collaboration : ML based models can help detect anomaly in standards and principals defined by organisation. It can also to a certian extent detect architectural flaws in the code and help raise early warning during code merge.
The Transformational Technologies Driving This Evolution
AI’s transformative impact on BFSI operations is underpinned by a suite of cutting-edge technologies that are seamlessly integrated into financial ecosystems:
Natural Language Processing (NLP):
NLP deciphers complex regulatory documents, extracts actionable insights, and enhances the clarity and accuracy of compliance reporting.Machine Learning Algorithms:
These algorithms power predictive modeling, fraud detection, and dynamic decision-making, enabling institutions to respond swiftly to changing conditions.Blockchain Integration:
Blockchain ensures secure, immutable records for financial reporting, particularly in multi-party transactions, enhancing transparency and trust.Advanced Data Analytics:
Analytics platforms process vast datasets to uncover actionable insights, detect trends, and optimize decision-making processes in real time.Cloud-Based Intelligent Systems:
Scalable and resilient cloud architectures enable financial institutions to adopt AI-driven systems without the limitations of traditional infrastructure.
The Path Forward: AI-Driven Evolution
AI has transitioned from being a tool to an indispensable enabler of strategic differentiation in finance and insurance. Its role extends beyond improving operational efficiencies to redefining how institutions innovate and deliver value.
Leaders in these sectors must approach AI with a dual focus:
Strategic Implementation: Prioritize use cases that align with business goals, whether enhancing customer journeys, driving operational efficiencies, or navigating complex regulatory landscapes.
Ethical AI Adoption: Foster trust by embedding transparency, accountability, and fairness into every AI initiative.
As organizations evolve, AI will not only transform the way they operate but also redefine the value they deliver to customers, stakeholders, and society. The future of finance and insurance lies in the seamless integration of AI into every facet of their digital ecosystems.
Balancing Hyper-Personalization with Ethical AI
The adoption of AI introduces challenges related to data privacy and algorithmic transparency. For financial institutions, which handle highly sensitive data, maintaining trust is paramount.
Privacy-First Personalization: Institutions must implement mechanisms like anonymization and encryption to balance personalization with privacy.
Transparent AI Models: AI systems must be explainable, ensuring that decisions (e.g., loan rejections or premium increases) are well-documented and free from bias.
By embedding ethical principles into their AI frameworks, organizations can foster trust while delivering value.
Mapping Digital Transformation with Business Goals and ROI
Well, We all agree that the cost of digital transformation, regardless of its scale, is a significant financial undertaking that most institutions or organizations do not have a dedicated department to thoroughly assess and quantify. As a result, the first set of questions from the board is often focused on justifying the investment required for such a transformation. One of the most compelling arguments in favour of digital transformation, particularly with the integration of AI technologies, is the substantial potential for return on investment (ROI) it offers. For banks and financial institutions, AI can drive efficiency, optimize revenue streams, and significantly enhance customer satisfaction, all of which contribute to a strong business case for the transformation. By leveraging AI, institutions can not only streamline operations and reduce costs but also unlock new opportunities for growth and innovation, ultimately leading to a more competitive and resilient organization
Mapping with Institution/Business Goals
Clear Alignment with Business Goals : Define specific business outcomes (e.g., improved customer experience, efficiency, scalability) and ensure alignment with the organization’s strategy, emphasizing customer experience as a key driver.
Understanding ROI : Conduct a cost-benefit analysis of transformation expenses vs. expected returns like efficiency, growth, and compliance. Set KPIs to track ROI, such as cost savings, service speed, and customer satisfaction.
Technology Assessment, Size, and Maturity : Evaluate current infrastructure, digital maturity, tech stack, and skills. Ensure scalability and external expertise readiness. Prepare for cultural shifts and assess the impact on processes and operational agility.
Leadership Commitment and Support : Secure C-suite commitment and establish a clear governance structure with defined roles and responsibilities for stakeholders.
Mapping Transformation to Customer Experience : Enhance customer experience across touchpoints using AI for personalization and automation, ensuring seamless integration across all channels.
Timeline and Milestones : Implement the transformation in phases with clear milestones. Use pilot programs to test and refine technologies before full-scale adoption.
Regulatory and Compliance Considerations : Ensure compliance with regulations (e.g., GDPR, PCI DSS, AML) and establish robust data governance for security and effective decision-making. Integrate compliance systems into the digital infrastructure.
Some of my thoughts where AI can directly impact ROI:
Operational Efficiency: AI can automate routine tasks, reducing operational costs and freeing up resources for higher-value activities. It can streamline workflows and predicts disruptions, resulting in cost savings and improved service delivery.
Proactive Risk Management: AI enhances credit risk assessment, fraud detection, and compliance by analysing vast datasets in real-time. This can further reduce losses, ensures regulatory compliance, and avoids penalties, leading to more stable returns.
Revenue Optimization: AI can enable dynamic pricing for loans and premiums, adjusting based on market conditions and customer profiles ensuring competitive pricing while managing risk, optimizing revenue without compromising profitability.
Customer Personalization: AI can provide tailored services to individual customer needs, driving loyalty, higher conversion rates, and upselling opportunities. Personalized offerings boost revenue by increasing customer lifetime value.
Faster Product Innovation: AI can accelerates market response by simulating customer demand and product performance, enabling faster time-to-market and capturing new revenue streams.
Cost Reduction and Scalability: AI can reduce manual processes, enhancing resource allocation and cutting costs. Its scalability supports long-term growth, accommodating new products or markets without significant investment increases.
Closing Thoughts
AI is no longer a futuristic concept — it’s actively transforming the BFSI sector right now. It stands as the catalyst, driving innovation, efficiency, and customer-centricity. For CXOs and senior leaders, the imperative is clear: leverage AI to build resilient, intelligent, and future-ready organizations. The question is no longer whether AI will transform the industry, but how effectively your organization can embrace this change.
AI’s versatility ensures that its impact is not confined to any single vertical. It has the potential to redefine every aspect of the BFSI industry, from core operations like lending, investing, and compliance to transformative initiatives in customer experience, financial inclusion, and real-time risk management. It’s potential to drive smarter decision-making, enhance customer experiences, and improve operational efficiencies is unparalleled.
Organizations that effectively integrate AI into their strategy will not only gain competitive advantages but also unlock new opportunities to innovate and create value in a rapidly evolving financial ecosystem.
In the next chapter, we will see what could be the possible application of machine learning (ML), deep learning (DL), supervised and unsupervised learning, reinforcement learning (RL), generative AI, conversational AI, large language models (LLMs), graph neural networks (GNNs), image and facial recognition, digital twin, and natural language processing (NLP) in various sectors such as banking, finance, fintech, micro-finance, lending, BNPL, trading, capital markets, wealth management, trade finance, and payments.
Subscribe to my newsletter
Read articles from Ravinder Singh directly inside your inbox. Subscribe to the newsletter, and don't miss out.
Written by
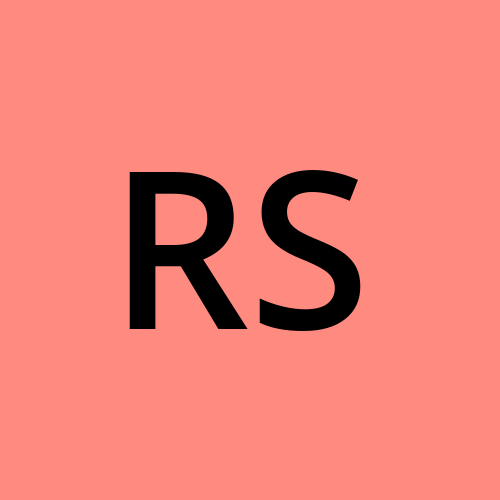
Ravinder Singh
Ravinder Singh
Senior Architect with 15 years of experience in development, Design, Analysis, and Implementation of large-scale distributed systems using Java, micro services, open source, messaging, streaming and cloud technologies. Experience in Core technology and deployment strategy, System Design, Architecture and Technology Consulting for various transformation, migration or Greenfield projects.