Part 3 - From Digital Transformation to AI Evolution: A New Chapter in BFSI
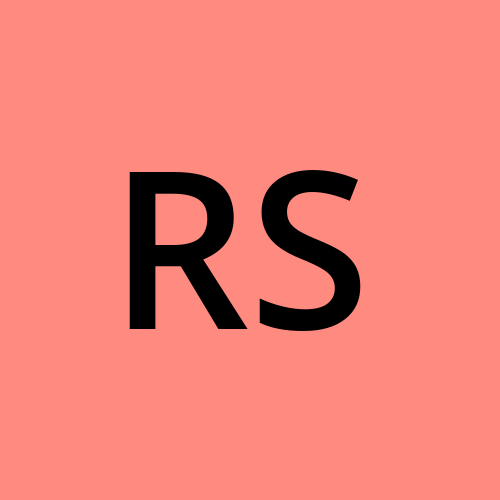
While in the previous blog, we got an overview of the transformative potential of AI across various sectors within the financial services industry, that discussion only scratches the surface — merely touching on the high-level implications. In reality, there is an extensive range of possibilities to explore in much greater depth. Specific AI techniques, such as Machine Learning (ML), Deep Learning (DL), Generative AI, Conversational AI, Large Language Models (LLMs), and Graph Neural Networks (GNNs), offer profound capabilities to tackle a wide array of complex and industry-specific challenges. Each of these technologies can be applied in unique ways to address pressing issues within different verticals of the Banking, Financial Services, and Insurance (BFSI) sectors — be it fraud detection, personalized financial advice, risk management, customer experience enhancement, or compliance monitoring. A more detailed examination of how these advanced AI methodologies can be strategically deployed to resolve specific pain points within the BFSI domain is essential for uncovering their full transformative potential.
Machine Learning (ML) in Banking and Financial Services
Imagine you’re a bank manager with millions of customers. How do you identify who’s about to default on their loan or detect fraudulent activity among billions of transactions? This is where ML steps in. It can find unusual patterns that doesn’t belong and uncover new types of fraud before they spread. ML particularly can help in :
Credit Risk Scoring and Underwriting: ML algorithms analyse historical customer data and financial behaviour to assess the likelihood of a borrower defaulting on a loan. This helps in credit scoring for both traditional and alternative data sources (e.g., social media activity, mobile phone usage). Machine learning models assess creditworthiness and make underwriting decisions without the need for manual intervention.
Fraud Detection and Prevention:
ML models detect fraudulent patterns by identifying unusual behaviour in transaction data. Supervised learning techniques classify transactions as fraudulent or legitimate.
Anomaly Detection: Unsupervised learning techniques are used to detect rare or unusual transactions, flagging potential fraud cases based on evolving patterns.
Real-Time Fraud Monitoring: ML models monitor transaction data in real time to stop fraudulent activities before they are completed, using anomaly detection techniques.
Predictive Analytics for Stock and Bond Markets:
ML algorithms analyse past price data, trading volumes, and financial indicators to predict stock, bond, or commodity prices, enabling more informed trading decisions.
Techniques like regression and time series forecasting are employed to predict price movements.
Customer Lifetime Value (CLV) Prediction: ML models predict the long-term value of customers, helping banks personalize offers and marketing strategies based on customer behaviour, transaction history, and engagement.
Personalized Banking Services: ML algorithms recommend tailored banking products (e.g., credit cards, loans, or insurance) based on a customer’s transaction history, spending patterns, and financial goals.
Churn Prediction: ML models identify customers at risk of leaving the bank by analysing patterns in customer behaviour and engagement with the bank’s products and services.
Deep Learning (DL) in Financial Services
Sentiment Analysis and Market Prediction: DL techniques, particularly recurrent neural networks (RNNs) and transformers, analyse social media, financial news, and reports to gauge market sentiment and predict stock market movements. Sentiment analysis of financial news helps to predict price fluctuations and guide investment strategies.
Natural Language Processing (NLP): DL models like BERT and GPT are used to process unstructured data such as financial documents, news articles, and reports to extract insights and identify market-moving information.
Risk Management and Portfolio Optimization: Deep learning models help identify risks in portfolios by detecting non-linear patterns in large datasets and optimizing asset allocation in real-time based on risk/reward profiles.
Supervised Learning in Financial Services
Customer Segmentation : Supervised learning algorithms segment customers based on their financial behaviour (e.g., spending patterns, income levels, investment preferences). This allows for personalized financial products, marketing campaigns, and customer engagement strategies.
Loan Default Prediction: Supervised learning models use historical loan data to predict the likelihood of loan defaults, helping lenders reduce the risk of bad loans.
Portfolio Risk Analysis: ML models use labelled financial data to assess risk levels within a portfolio, enabling risk managers to make more informed decisions about portfolio diversification and asset allocation.
Unsupervised Learning in Financial Services
Fraud Detection: Unsupervised learning models are used for anomaly detection to find new types of fraud that are not detected by traditional fraud detection systems. Clustering techniques (e.g., k-means) group transactions by similarity, which helps identify unusual or fraudulent activities in financial transactions.
Market Segmentation: Unsupervised algorithms group customers or transactions into clusters based on behavioural data, enabling firms to understand different customer needs and personalize services.
Reinforcement Learning (RL) in Finance
Have you ever wondered how trading firms execute thousands of trades in milliseconds? The answer lies in Reinforcement Learning (RL). RL is like teaching a robot to play chess — it learns strategies through trial and error. In trading, RL algorithms analyse market trends, make decisions, and adapt in real-time.
Algorithmic Trading: RL is used to develop self-learning trading algorithms that adapt to market conditions over time. These systems optimize trading strategies by exploring different actions and receiving feedback from the market.
Portfolio Optimization: RL models are used to adjust portfolio allocations dynamically, learning over time which combinations of assets lead to optimal returns while minimizing risk.
Personalized Financial Advice: RL can optimize personalized investment strategies by continually learning from customer interactions and market conditions.
Generative AI in Financial Services
Synthetic Data Generation: Generative adversarial networks (GANs) generate synthetic financial data for training ML models when real data is limited or too sensitive (e.g., for privacy reasons). Financial institutions use synthetic data to train fraud detection and risk models without compromising privacy or security.
Financial Scenario Simulation: Generative AI is used to simulate various financial scenarios, such as market crashes or sudden economic changes, to help institutions stress-test their portfolios and risk management strategies.
Market Trend Prediction: Generative models simulate possible future market trends, helping investors understand a wider range of market possibilities and making better-informed decisions.
Conversational AI in Financial Services
How many of you have interacted with a chatbot that felt almost human? That’s Conversational AI in action! These chatbots are transforming customer service, handling millions of queries without breaking a sweat.
Virtual Assistants and Chatbots: AI-powered chatbots assist customers in tasks like account balance inquiries, bill payments, money transfers, loan application processes, and more. These chatbots use NLP and ML models to understand customer intent and provide personalized responses.
Personalized Financial Coaching: Conversational AI provides financial advice, investment recommendations, and budget management suggestions based on a customer’s financial behaviour and goals.
Large Language Models (LLMs) in Financial Services
Document Analysis and Processing: LLMs like GPT-3 and BERT extract key insights from large volumes of unstructured text, such as financial reports, contracts, or regulatory filings. This can help banks, investors, and analysts make data-driven decisions quickly.
Regulatory Compliance and Reporting: LLMs automatically scan regulations and compliance documents to ensure banks remain compliant with evolving laws, minimizing legal and financial risks.
Automated Customer Service: LLMs power intelligent virtual assistants and chatbots that can handle complex customer inquiries and provide real-time support in natural language.
Graph Neural Networks (GNNs) in Finance
Fraud Detection: GNNs model relationships between entities, such as accounts, transactions, and individuals. By analysing these relationships, GNNs help detect fraudulent behaviour based on unusual patterns or connections within the data.
Credit Scoring and Loan Approval: GNNs can model complex borrower relationships and transactions, improving the accuracy of credit risk assessments and loan approvals by understanding the broader financial context.
Financial Network Analysis: GNNs are used to map and analyse relationships in financial networks, helping identify systemic risks, market trends, and interconnected financial institutions.
Image and Facial Recognition in Financial Services
Biometric Authentication: Image recognition technologies are used in biometric authentication for secure customer identification during logins, ATM withdrawals, or mobile banking transactions.
Document Verification: Image recognition algorithms extract and validate information from scanned documents (e.g., ID cards, utility bills) for KYC (Know Your Customer) compliance during account opening and loan applications.
Cheques and Bills Processing: Image recognition is employed for automating cheque and bill payments by capturing and reading the details from images, streamlining the process and reducing errors.
Digital Twin in Financial Services
Customer Behaviour Modelling: A digital twin of a customer creates a virtual model that continuously learns and predicts their financial behaviour, allowing for personalized product offerings and proactive customer service.
Risk Simulation and Stress Testing: Financial institutions can use digital twins to simulate economic conditions and financial risks, enabling them to test how portfolios or financial systems might perform under various adverse conditions.
Investment Simulation: Digital twins of investment portfolios help simulate various financial scenarios to assess potential outcomes and optimize investment strategies.
Natural Language Processing (NLP) in Financial Services
Sentiment Analysis: NLP techniques analyze financial news, reports, and social media to gauge market sentiment and predict stock market movements. This can be used by traders and investors to gain insights into market conditions.
Document and Contract Management: NLP automates the extraction of key information from financial documents (e.g., loan contracts, regulatory filings), saving time and improving accuracy in decision-making processes.
Compliance Monitoring: NLP tools help financial institutions monitor and interpret changes in regulations and compliance requirements, ensuring adherence to legal standards and reducing regulatory risks.
Applications in Specific Sectors:
- Microfinance and Lending:
Credit Scoring with Alternative Data: ML models use non-traditional data like mobile phone usage and social interactions to score borrowers without credit histories.
Loan Default Prediction: Predictive models assess loan repayment likelihood, helping microfinance institutions minimize defaults.
2. Buy Now, Pay Later (BNPL):
Risk Profiling: ML models assess the creditworthiness of BNPL users by analysing purchase behaviour and transaction data.
Fraud Detection: AI-based systems detect fraudulent transactions in BNPL services by monitoring spending patterns and flagging anomalies.
3. Capital Markets:
Algorithmic Trading: Advanced ML and RL models predict stock and commodity price movements and execute trades in real time.
Market Sentiment Analysis: NLP and sentiment analysis tools process news, social media, and financial reports to gauge market sentiment and inform trading strategies.
4. Wealth Management:
Robo-Advisory: AI algorithms provide automated investment advice based on individual preferences, financial goals, and risk tolerance.
Portfolio Optimization: AI models dynamically optimize investment portfolios, considering risk, return, and economic conditions.
5. Trade Finance:
Document Automation: NLP and AI tools automatically process trade-related documents, reducing manual errors and accelerating transactions.
Fraud Detection in Trade: AI detects fraudulent trade activities by analysing patterns in trade documents, payments, and shipping data.
6. Payments Industry:
Fraud Prevention: AI models continuously analyse transaction patterns to identify fraudulent payments in real time.
Smart Payments: AI and ML are used to optimize payment processing, ensuring quicker and more secure transactions.
These advanced technologies are transforming financial services, creating efficiencies, enabling better risk management, and providing a more personalized experience for customers. As these tools evolve, the scope for their applications will continue to grow and expand across the financial ecosystem.
A Closing thought : Can AI replace Human in Financial Sector
The idea of Artificial Intelligence (AI) completely replacing humans in the finance industry might sound intriguing with the use-cases and application we discussed above, but it remains highly improbable. While AI is undeniably powerful, many decisions — especially those involving investing, strategic planning, and relationship management — demand a level of personal judgment, emotional intelligence, and ethical discernment that only humans can provide. The ability to interpret complex contexts, navigate uncertainty, and make value-based decisions keeps humans firmly at the center of critical financial operations.
That said, the role of AI is transformative and undeniable. Its unparalleled capacity for processing vast amounts of data, identifying patterns, and executing tasks with speed and precision has revolutionized operational efficiencies. By automating routine and lower-level tasks, such as financial research, data analysis, and underwriting, AI allows professionals to shift their focus to more strategic and innovative aspects of their roles.
Imagine a financial analyst spending less time combing through reports and more time crafting tailored investment strategies. Or consider how AI-powered tools can assist underwriters in evaluating risks more accurately, leaving them free to engage in customer-centric decision-making. These are just glimpses of how AI is not here to replace but rather to augment human capabilities.
Looking ahead, the synergy between AI and human expertise is set to redefine the finance industry. AI will continue to grow, driving cost savings, accelerating decision-making processes, and unlocking new possibilities. But at its core, it will remain a tool — a powerful ally — supporting the unique ingenuity and adaptability of human professionals. The future of finance isn’t about choosing between humans and AI; it’s about leveraging the best of both to create smarter, more innovative solutions.
What’s Next
In Parts 1 and Part 2 of this series, we discussed the transformative potential of Artificial Intelligence (AI) within the banking, financial services, and payments sectors, discussing a variety of impactful use cases and the ways AI is reshaping the industry. These sections set the stage by showcasing how AI can address complex challenges, enhance customer experiences, drive operational efficiencies, and unlock new business opportunities.
Looking ahead, the next section will take a more comprehensive and technical dive into the practical implementation of AI within financial organizations and the challenges that institutions face during this transformation journey. We will focus on the steps required to bring AI-driven transformation to life, emphasizing the role of cutting-edge technologies, architectural strategies, and organizational readiness. Whether an institution is just beginning its digital transformation journey or has already made significant progress by establishing foundational capabilities, we will explore tailored approaches to ensure successful adoption and value realization. From building scalable AI infrastructures to overcoming integration hurdles, we aim to provide a clear roadmap for navigating this transformative journey. Stay tuned for an in-depth exploration and actionable strategies to propel your organization into the future of finance.
Subscribe to my newsletter
Read articles from Ravinder Singh directly inside your inbox. Subscribe to the newsletter, and don't miss out.
Written by
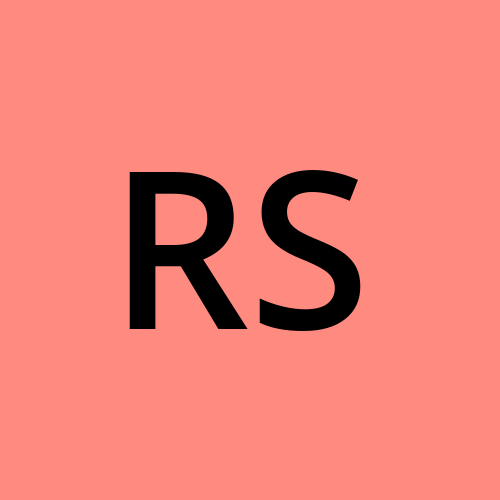
Ravinder Singh
Ravinder Singh
Senior Architect with 15 years of experience in development, Design, Analysis, and Implementation of large-scale distributed systems using Java, micro services, open source, messaging, streaming and cloud technologies. Experience in Core technology and deployment strategy, System Design, Architecture and Technology Consulting for various transformation, migration or Greenfield projects.