Teaching Gemini: How Models and Structured Prompts Actually Work

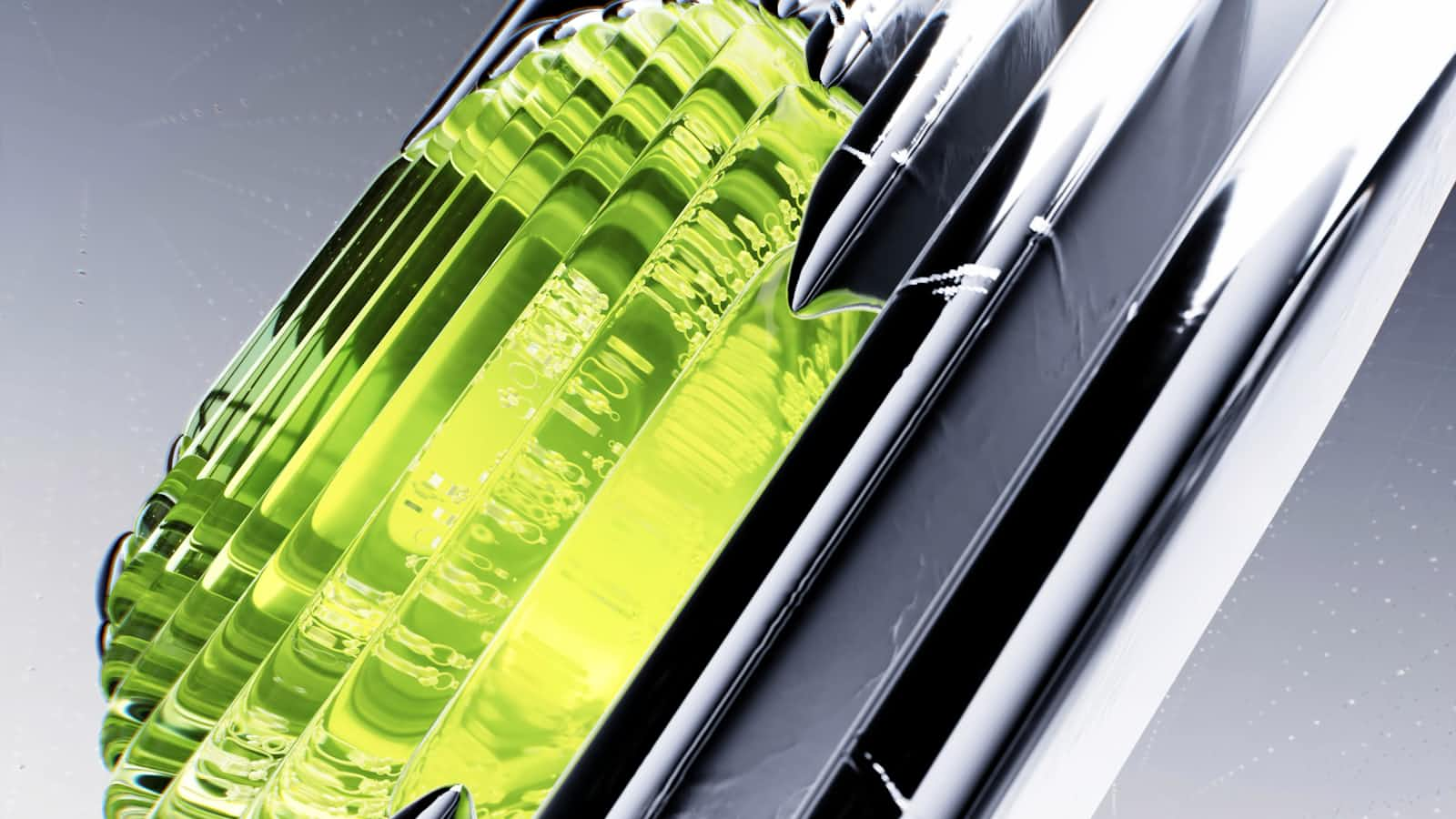
Google recently added a powerful new feature to Google AI Studio—it’s called Structured Prompts. You’ll find it in the dashboard when you’re working with Gemini, Google’s generative AI model.
At first, it might sound confusing—Gemini, AI Studio, tuning models—it all starts to feel like something out of Star Trek. Like you’re talking to a glowing orb in space. But it’s actually way simpler than that.
Here’s the real deal:
Structured prompts let you teach Gemini how to respond to specific types of content. You give it:
An input (like a paragraph from a government report or blog post)
An output (how you want it to summarize or respond)
That pairing is called a training example, and once you start adding multiple examples, you’re not just messing around with prompts—you’re actually training a model.
And yes, a “model” isn’t some floating black box in space. It’s just a version of Gemini that has learned your style. You're showing it patterns—how you think, what you want, what matters in your work.
Let’s say you're summarizing government documents—writing plain English summaries, or building brief descriptions for policy records.
That’s exactly the kind of work I’ve been doing with the LoCALDig project at the Institute of Governmental Studies Library at UC Berkeley. We’re experimenting with ways to make large collections of government documents more searchable and usable for the public.
Instead of prompting Gemini from scratch every time, I’ve been testing how to train it to understand how we might want summaries written. You’re shaping the model’s instinct, so it responds in a consistent, reliable way that reflects your goals.
When I first started learning about prompts, I had this question:
“Why train a model at all? Can’t I just create one perfect input and output and be done?”
Here’s what I learned:
If you train an AI model with only one example, you’ve shown it one scenario—but not a wide-enough range. It won’t know how to handle variety. For example, if you train the model to write summaries using one planning document from Fresno, California about housing policy, it might fail when you give it a totally different one—like a sewer bond report from Merced or a flood control document from Humboldt. Without seeing a range of styles, topics, and wording, AI starts guessing. And if you don’t correct it, it’ll drift farther and farther off track—thinking it’s doing fine the whole time.
Training a model means creating guardrails. You’re not just feeding it instructions. You’re shaping behavior. And once it’s tuned, that version of Gemini is ready to deploy, so you can stop re-explaining things and confidently let it just do the job right.
That’s what structured prompts are for. And that’s what a model really is. No orb. No Star Trek. Just pattern, logic, and learning.
Subscribe to my newsletter
Read articles from Nick Norman directly inside your inbox. Subscribe to the newsletter, and don't miss out.
Written by
