Retrieval-Augmented Generation: Solving the Reliability Crisis in Large Language Models

Table of contents
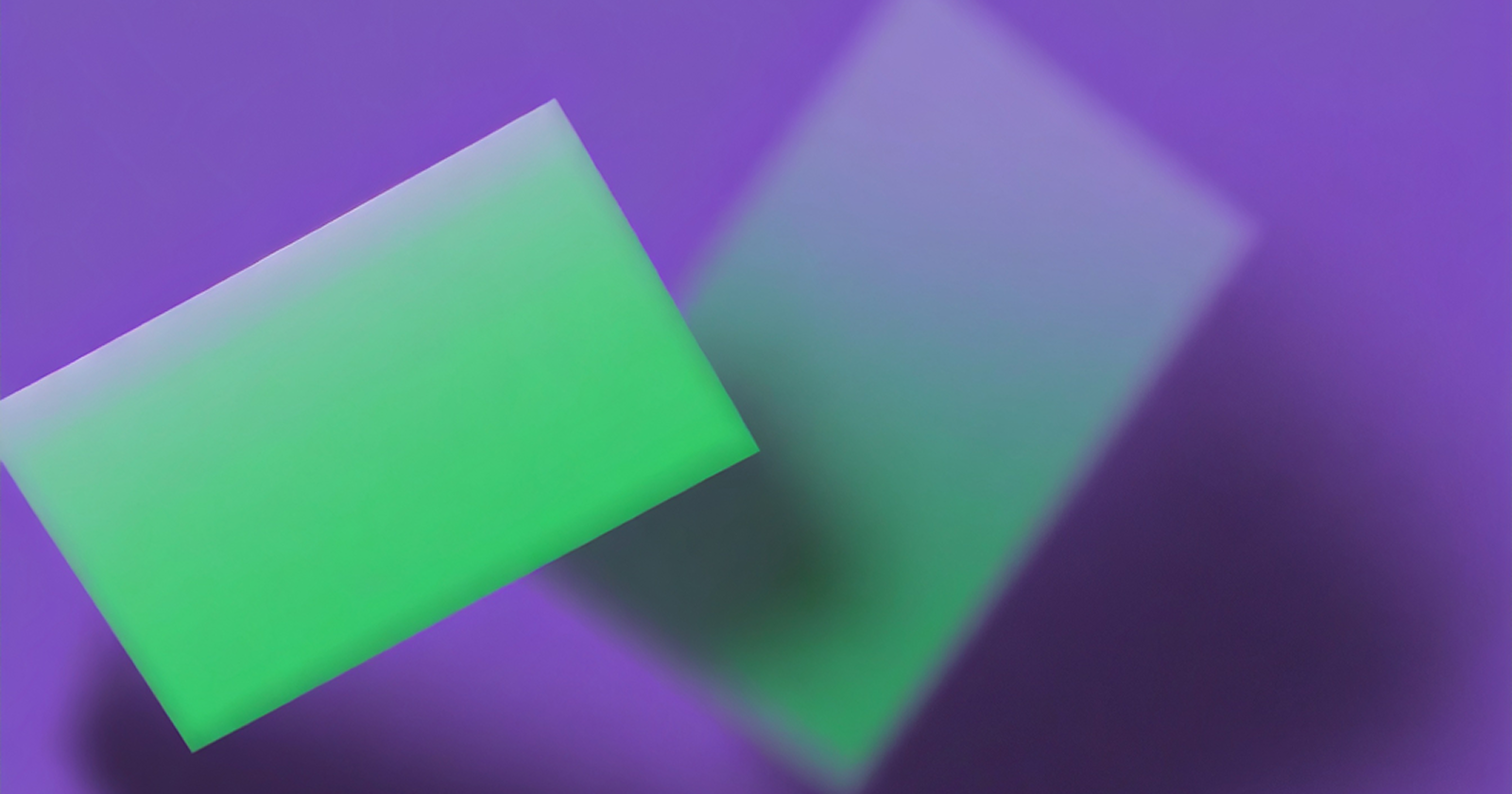
In the rapidly evolving world of artificial intelligence, large language models have promised transformative capabilities—but they've consistently fallen short in one critical area: reliability. Retrieval-Augmented Generation (RAG) is emerging as the breakthrough technology that's bridging this crucial gap.
The Fundamental Problem with AI Language Models
Traditional AI models are like brilliant students who occasionally make up answers during an exam. They generate text with remarkable fluency, but without a genuine connection to factual truth. Hallucinations—completely fabricated "facts"—have been the Achilles' heel of AI language technologies.
Enter Retrieval-Augmented Generation
RAG isn't just an incremental improvement; it's a fundamental reimagining of how AI systems interact with information. By dynamically retrieving and integrating external knowledge, RAG transforms large language models from creative guessers into precise, verifiable information processors.
How RAG Actually Works
The RAG framework operates through a sophisticated four-stage process:
Query Processing: Intelligently parsing the initial information request
Retrieval: Searching external knowledge bases for relevant information
Contextualization: Integrating retrieved data with the original query
Generation: Producing responses grounded in actual, verifiable sources
Tangible Benefits for Real-World Applications
Organizations implementing RAG are experiencing transformative results:
Accuracy Improvements: Up to 30% reduction in factual errors
Dynamic Knowledge Updates: Eliminating traditional training data limitations
Domain-Specific Expertise: Instant adaptation to specialized fields
Transparent Information Sources: Traceable, citable response generation
Technical Challenges and Innovative Solutions
While RAG represents a significant leap forward, it's not without complexity. Advanced implementations are exploring:
Multi-modal information retrieval
Adaptive search strategies
Self-verifying retrieval mechanisms
Multi-agent collaborative architectures
The Infrastructure Imperative
Deploying sophisticated RAG systems requires more than sophisticated algorithms—it demands robust, scalable infrastructure. This is where platforms like Genezio become critical, offering serverless environments specifically designed to handle the computational demands of advanced AI systems.
Future Horizons: Beyond Current Limitations
RAG is just the beginning of a broader transformation in artificial intelligence. We're witnessing the emergence of systems that don't just generate text, but genuinely understand and contextualize information.
The Road Ahead
As AI continues to evolve, retrieval-augmented generation represents our most promising path toward more reliable, transparent, and genuinely intelligent systems.
Explore the Full RAG Landscape
This overview merely hints at the complexities of retrieval-augmented generation. For a comprehensive, in-depth exploration of RAG's mechanics, challenges, and future potential, dive into Genezio’s blog and uncover the technologies reshaping artificial intelligence today!
Subscribe to my newsletter
Read articles from Horatiu Voicu directly inside your inbox. Subscribe to the newsletter, and don't miss out.
Written by
