AI-Driven Vaccine Development: Optimizing Immune Response Prediction

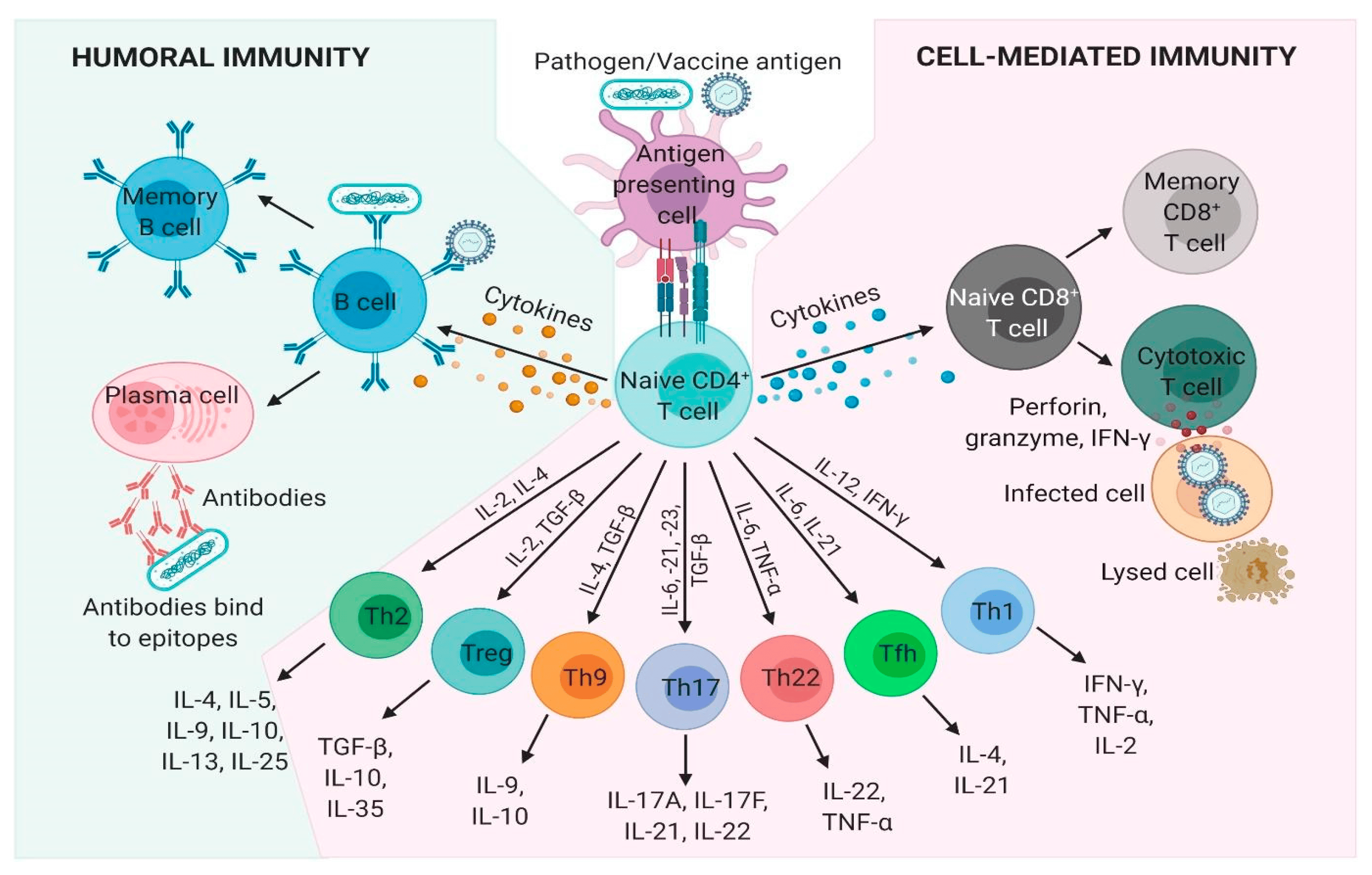
Introduction
Vaccine development has long been a cornerstone of global health, helping to prevent the spread of infectious diseases and reducing mortality rates. Traditionally, the process of developing a vaccine is time-consuming, often taking years or even decades. However, advancements in artificial intelligence (AI) are revolutionizing this field by significantly accelerating vaccine discovery, optimizing immune response prediction, and improving vaccine efficacy. AI-driven approaches offer novel solutions to predict how the immune system will respond to various vaccine candidates, ultimately enhancing vaccine design and deployment.
This article explores how AI is transforming vaccine development, focusing on its role in immune response prediction, the integration of machine learning models, and future prospects for AI-driven immunology.
The Challenges of Traditional Vaccine Development
Developing a vaccine typically involves multiple stages, including antigen identification, preclinical testing, clinical trials, and regulatory approval. Each of these phases presents challenges:
Time-Consuming Research – Traditional vaccine development can take years due to lengthy trial-and-error methods in antigen selection and immune response evaluation.
High Costs – The financial burden of vaccine research and development can reach billions of dollars, particularly if a vaccine candidate fails during clinical trials.
Complex Immune System Interactions – The human immune system is intricate, and predicting its response to a new vaccine is difficult using traditional experimental methods alone.
Pathogen Evolution – Viruses and bacteria constantly evolve, leading to potential vaccine resistance and necessitating continuous research and updates.
AI offers a paradigm shift by addressing these challenges with predictive models, large-scale data analysis, and automation.
EQ.1: Epitope Binding Affinity Prediction (Machine Learning Model)
How AI is Optimizing Immune Response Prediction
AI-driven vaccine development primarily focuses on immune response prediction. This involves using algorithms and machine learning models to analyze vast amounts of biological and immunological data, providing insights into how a vaccine will interact with the immune system.
1. Machine Learning for Antigen Selection
The first step in vaccine design is identifying suitable antigens—molecules that trigger an immune response. AI can analyze genomic, proteomic, and structural data to predict which viral or bacterial components are most immunogenic (i.e., likely to elicit a strong immune response). Machine learning models can:
Analyze viral sequences to detect conserved regions less prone to mutation.
Predict epitope binding—epitopes are small portions of an antigen that the immune system recognizes. AI models can determine which epitopes will be effectively presented by major histocompatibility complex (MHC) molecules, crucial for immune activation.
Reduce reliance on trial-and-error by selecting the most promising vaccine candidates before laboratory testing begins.
2. Deep Learning for Immune System Simulation
Deep learning algorithms, such as convolutional neural networks (CNNs) and recurrent neural networks (RNNs), can simulate immune system interactions with vaccines. By training these models on past immunological data, researchers can predict:
Vaccine efficacy in different populations, considering genetic variations.
Potential adverse effects by identifying molecular structures similar to known allergens or autoantigens.
Long-term immunity by modeling how the immune system will respond over time.
For example, AI-driven simulations have played a crucial role in mRNA vaccine development, as seen with COVID-19 vaccines like those from Pfizer-BioNTech and Moderna.
AI Applications in Vaccine Development
1. Natural Language Processing (NLP) for Research Acceleration
AI-powered NLP tools help researchers process and analyze vast amounts of scientific literature, extracting relevant insights and identifying trends in vaccine development. This reduces redundancy and enhances collaboration by making previous research more accessible.
2. Generative AI for New Antigen Design
Generative models, such as generative adversarial networks (GANs) and transformer-based AI, can design new vaccine candidates by generating artificial proteins with enhanced immunogenicity. These AI-designed antigens can be tested in silico (via computer simulations) before being synthesized in a lab.
3. AI in Clinical Trials and Patient Selection
Machine learning assists in optimizing clinical trial design by:
Selecting diverse patient cohorts to ensure efficacy across populations.
Predicting adverse effects based on patient genetics and medical history.
Reducing trial duration by identifying biomarkers that indicate vaccine success early on.
These AI-driven approaches can help reduce clinical trial failures and bring vaccines to market faster.
Real-World Applications of AI in Vaccine Development
1. COVID-19 Vaccine Development
During the COVID-19 pandemic, AI played a significant role in vaccine development. Researchers used machine learning to analyze SARS-CoV-2 protein structures and predict the best targets for vaccines. AI-driven protein-folding algorithms, such as DeepMind’s AlphaFold, provided accurate 3D models of viral proteins, aiding in vaccine design.
2. Cancer Vaccines
AI is also being used to develop personalized cancer vaccines by predicting tumor-specific neoantigens—mutated proteins unique to a patient’s cancer cells. These vaccines stimulate an immune response specifically against the tumor while minimizing damage to healthy tissues.
3. Influenza and Future Pandemic Preparedness
AI is helping predict how influenza viruses evolve, allowing scientists to design more effective flu vaccines each year. Machine learning models analyze past mutations to forecast future strains, improving vaccine strain selection. This approach could also be applied to emerging pandemics, enabling rapid vaccine response.
EQ.2: Immune Response Prediction Using Ordinary Differential Equations (ODEs)
Ethical and Regulatory Considerations
Despite its potential, AI-driven vaccine development comes with ethical and regulatory challenges:
Data Privacy – AI requires vast amounts of genomic and medical data, raising concerns about patient privacy and data security.
Algorithm Bias – AI models may reflect biases present in training data, leading to disparities in vaccine efficacy among different populations.
Regulatory Approval – AI-generated vaccine candidates must undergo rigorous regulatory scrutiny to ensure safety and efficacy.
Transparency in AI Decision-Making – Black-box AI models must be interpretable so scientists and regulators can understand how decisions are made.
To address these challenges, researchers and policymakers must develop ethical frameworks for AI-driven vaccine development.
The Future of AI in Vaccine Development
AI’s role in vaccine development will continue to grow, leading to:
Faster vaccine discovery through real-time AI simulations.
Personalized vaccines tailored to individuals based on genetic and immune profiles.
Greater automation in vaccine manufacturing, reducing costs and improving scalability.
Enhanced surveillance of emerging pathogens using AI-powered monitoring systems.
As AI technologies advance, we can expect a new era of precision medicine, where vaccines are not only developed rapidly but also optimized for maximum effectiveness in diverse populations.
Conclusion
AI-driven vaccine development is revolutionizing the way scientists predict immune responses and design effective vaccines. By leveraging machine learning, deep learning, and computational modeling, AI significantly reduces the time and cost associated with traditional vaccine development. From COVID-19 to personalized cancer vaccines, AI is enabling breakthroughs that were previously unimaginable.
As this technology continues to evolve, collaboration between AI researchers, immunologists, and policymakers will be crucial in ensuring that AI-driven vaccines are safe, effective, and accessible to all. The integration of AI into immunology marks a turning point in global health, offering new hope for tackling infectious diseases and preparing for future pandemics.
Subscribe to my newsletter
Read articles from Sambasiva Rao Suura directly inside your inbox. Subscribe to the newsletter, and don't miss out.
Written by
