Advanced Statistical Techniques Every Business Analyst Should Know

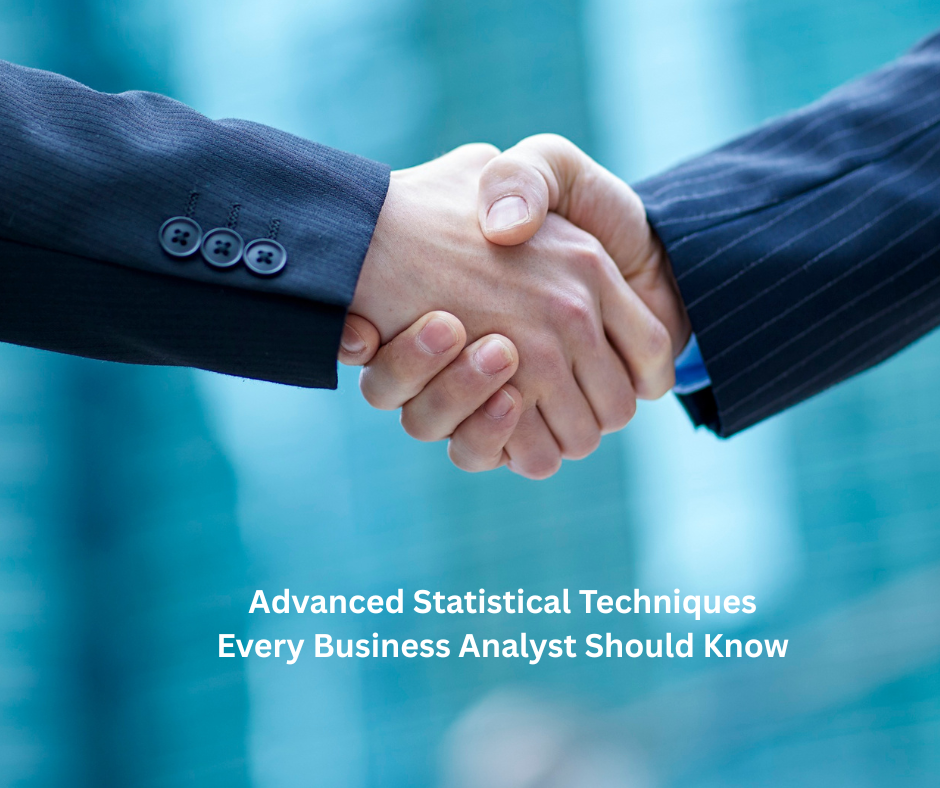
As a business analyst, understanding and leveraging advanced statistical techniques can significantly enhance your ability to make data-driven decisions and provide insights that drive business success. In this article, we will explore some of the most powerful statistical methods that can help business analysts better analyze data, forecast trends, and support business strategies.
Regression Analysis: Predicting Future Trends
What is Regression Analysis?
Regression analysis is one of the most widely used statistical techniques in business analysis. It helps business analysts understand the relationship between a dependent variable (the outcome you're trying to predict) and one or more independent variables (factors that influence the outcome).
Types of Regression
- Linear Regression: This method predicts the value of the dependent variable based on a linear relationship with the independent variable(s).
- Multiple Regression: In this technique, multiple independent variables are used to predict the outcome. It helps when there are several factors affecting the outcome.
Why is it Important?
Regression analysis is crucial for forecasting sales, understanding customer behavior, and predicting market trends. For example, a retail company might use regression analysis to predict future sales based on factors like advertising spend, seasonality, and customer demographics.
Time Series Analysis: Analyzing Trends Over Time
What is Time Series Analysis?
Time series analysis involves analyzing data points that are collected or recorded at specific time intervals. The goal is to identify underlying patterns, trends, and seasonal effects in the data, which can be used to make future predictions.
Common Time Series Techniques
- Moving Averages: A simple method of smoothing out short-term fluctuations to reveal long-term trends.
- Exponential Smoothing: A weighted moving average method that gives more weight to recent data points.
- ARIMA (AutoRegressive Integrated Moving Average): A sophisticated model that combines autoregressive and moving average techniques to model time series data.
Why is it Important?
For business analysts, time series analysis is vital in forecasting demand, inventory, and sales, among other business metrics. It helps businesses plan for the future and make more informed decisions based on past performance.
Hypothesis Testing: Making Data-Driven Decisions
What is Hypothesis Testing?
Hypothesis testing allows business analysts to make decisions based on sample data. It involves setting up two competing hypotheses—null and alternative—and using statistical methods to determine which hypothesis is more likely based on the data.
Steps in Hypothesis Testing
- Formulate Hypotheses: Start by stating the null hypothesis (H0) and the alternative hypothesis (H1).
- Choose a Significance Level (Alpha): This is usually set at 0.05, meaning a 5% chance of incorrectly rejecting the null hypothesis.
- Calculate the Test Statistic: Using your data, calculate the test statistic (e.g., t-test, chi-square test).
- Make a Decision: Based on the calculated p-value, determine whether to reject or fail to reject the null hypothesis.
Why is it Important?
Hypothesis testing is valuable for business analysts when evaluating changes in business practices, products, or services. For instance, a company might want to test if a new marketing campaign leads to increased sales, and hypothesis testing can help determine the effectiveness of such campaigns.
Cluster Analysis: Grouping Similar Data Points
What is Cluster Analysis?
Cluster analysis is an unsupervised machine learning technique used to group similar data points together based on shared characteristics. The goal is to identify natural groupings within the data, which can help businesses segment customers, products, or other relevant groups.
Popular Clustering Algorithms
- K-Means Clustering: This algorithm divides the data into 'K' clusters by minimizing the variance within each cluster.
- Hierarchical Clustering: This method builds a hierarchy of clusters, which can be useful for understanding nested relationships.
Why is it Important?
Cluster analysis is often used in market segmentation. For example, a business might use clustering to identify different types of customers, allowing them to tailor marketing strategies and improve customer targeting.
Principal Component Analysis (PCA): Reducing Data Dimensionality
What is Principal Component Analysis (PCA)?
PCA is a statistical technique used to reduce the dimensionality of large datasets while preserving as much variance as possible. By transforming the data into a new set of variables (principal components), PCA simplifies complex datasets without losing critical information.
Why is it Important?
Business analysts often deal with large datasets that include many variables. PCA helps in reducing the complexity of such datasets, making it easier to visualize and analyze data. For instance, PCA can help in identifying key features in customer data that drive purchasing decisions.
Bayesian Analysis: Updating Beliefs with New Data
What is Bayesian Analysis?
Bayesian analysis is a statistical method that allows business analysts to update the probability for a hypothesis as more evidence or data becomes available. Unlike traditional frequentist methods, Bayesian analysis incorporates prior knowledge and beliefs in the analysis.
Why is it Important?
In business, decisions are often based on uncertain information. Bayesian analysis helps analysts incorporate prior knowledge and continuously update their models as new data comes in. It’s particularly useful in forecasting and decision-making under uncertainty.
Decision Trees: Visualizing Decision-Making
What is a Decision Tree?
A decision tree is a flowchart-like structure used to represent decisions and their possible consequences, including outcomes, costs, and probabilities. Each branch of the tree represents a decision or action, and the leaves represent the outcomes.
Why is it Important?
Decision trees help business analysts visualize complex decision-making processes and outcomes. They are particularly useful in risk analysis, customer segmentation, and marketing strategies. For instance, a business could use decision trees to determine the best course of action for customer retention strategies.
Conclusion
Advanced statistical techniques empower business analysts to extract meaningful insights from data, predict future trends, and make more informed decisions. Whether you're using regression analysis to forecast sales, time series analysis to identify trends, or hypothesis testing to evaluate strategies, these techniques can greatly enhance the value of your work. To master these skills, you can enroll in Business Analyst classes in Delhi, Noida, Pune, Bangalore, and other parts of India, where you will receive expert guidance and hands-on experience in applying these advanced methods.
Subscribe to my newsletter
Read articles from Ruhi Parveen directly inside your inbox. Subscribe to the newsletter, and don't miss out.
Written by

Ruhi Parveen
Ruhi Parveen
I am a Digital Marketer and Content Marketing Specialist, I enjoy technical and non-technical writing. I enjoy learning something new.