Cloud-Based AI for Real-Time Data Processing and Decision-Making

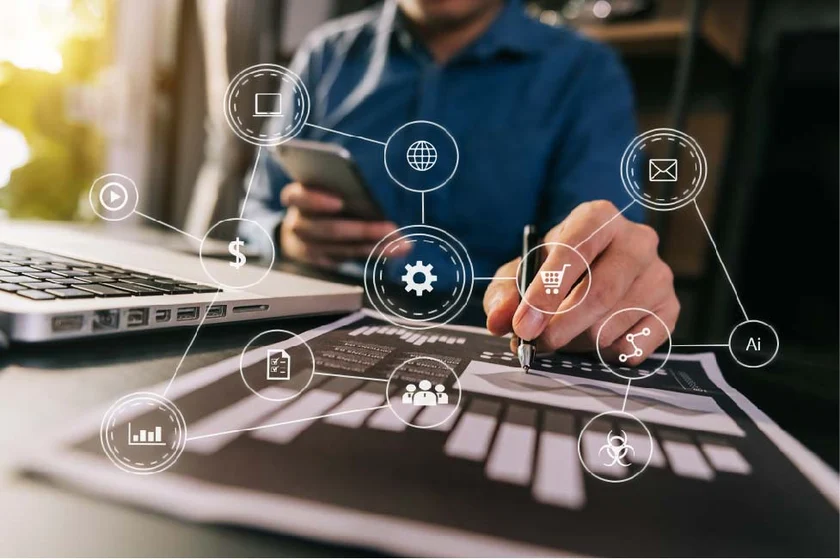
Introduction
The exponential growth of data generated by businesses, IoT devices, and digital applications has created a demand for real-time data processing and decision-making. Traditional computing architectures often struggle to handle vast amounts of data with low latency. Cloud-based artificial intelligence (AI) has emerged as a transformative solution, leveraging scalable computing resources, machine learning (ML), and automation to process and analyze data in real time. By integrating AI with cloud computing, organizations can enhance operational efficiency, improve decision-making, and drive innovation across industries.
Cloud-Based AI: An Overview
Cloud-based AI refers to the deployment of artificial intelligence models and applications on cloud infrastructure, enabling on-demand access to computing power, storage, and AI-driven analytics. This approach eliminates the need for expensive on-premise hardware while providing the flexibility to scale resources dynamically.
AI-powered cloud platforms utilize technologies such as deep learning, natural language processing (NLP), and predictive analytics to process real-time data streams from multiple sources. These capabilities make cloud-based AI essential for applications in healthcare, finance, manufacturing, logistics, and smart cities.
Key Components of Cloud-Based AI for Real-Time Data Processing
Edge Computing and AI Integration – AI models deployed on the cloud can work in conjunction with edge devices (e.g., IoT sensors, mobile devices) to process data closer to the source, reducing latency.
Machine Learning and Predictive Analytics – Cloud-based AI continuously learns from incoming data to improve decision-making and detect patterns in real time.
Big Data Analytics – AI-powered cloud solutions process large datasets efficiently, extracting valuable insights for businesses.
Streaming Data Processing – Technologies like Apache Kafka and AWS Kinesis allow AI to analyze real-time data streams from multiple sources, enabling instant decision-making.
Automation and AI-Driven Decision Support – AI automates complex workflows, reducing human intervention while enhancing accuracy in decision-making.
EQ.1.VMachine Learning for Real-Time Predictions
Applications of Cloud-Based AI in Real-Time Data Processing and Decision Making
1. Healthcare and Medical Diagnosis
Cloud-based AI plays a vital role in healthcare by enabling real-time analysis of medical images, patient vitals, and genomic data. AI-driven diagnostic tools assist doctors in identifying diseases, predicting patient deterioration, and personalizing treatment plans.
For instance, AI-powered cloud platforms analyze X-rays and MRI scans instantly, providing automated diagnoses and flagging abnormalities. Telemedicine applications leverage cloud AI to monitor patients remotely, alerting healthcare providers to critical conditions in real time.
2. Financial Fraud Detection and Risk Management
Financial institutions rely on cloud-based AI to detect fraudulent transactions, assess credit risk, and optimize trading strategies. By analyzing transaction patterns in real time, AI models identify anomalies and trigger alerts for suspicious activities.
AI-driven fraud detection systems use real-time behavioral analytics to flag unauthorized transactions, preventing financial losses. Additionally, AI-based predictive models help banks assess customer creditworthiness and minimize default risks.
3. Smart Manufacturing and Industry 4.0
In manufacturing, cloud-based AI enables predictive maintenance, quality control, and supply chain optimization. AI models analyze real-time sensor data from industrial machinery to predict equipment failures, reducing downtime and improving efficiency.
For example, factories use AI-powered vision systems to inspect products for defects in real time, ensuring high-quality manufacturing. AI also optimizes supply chain logistics by predicting demand fluctuations and automating inventory management.
4. Autonomous Vehicles and Transportation
Self-driving cars and smart transportation systems depend on cloud-based AI to process vast amounts of real-time sensor data. AI-powered cloud platforms analyze traffic patterns, pedestrian movements, and vehicle diagnostics to make instant driving decisions.
AI-driven traffic management systems optimize routes, reducing congestion and improving urban mobility. Logistics companies use cloud AI to track fleet movements and enhance delivery efficiency through predictive route planning.
5. Retail and Customer Experience Optimization
Retailers leverage cloud-based AI for real-time customer behavior analysis, personalized recommendations, and demand forecasting. AI-powered recommendation engines analyze browsing history and purchase patterns to suggest relevant products, enhancing customer engagement.
In physical stores, AI-driven analytics track foot traffic and inventory levels, helping retailers optimize product placement and manage stock efficiently. Chatbots powered by AI provide instant customer support, improving response times and service quality.
6. Cybersecurity and Threat Detection
Cloud-based AI enhances cybersecurity by detecting and preventing cyber threats in real time. AI models analyze network traffic, user behavior, and system logs to identify potential breaches and mitigate risks automatically.
For example, AI-driven security systems detect unusual login attempts, phishing emails, and malware infections by comparing current behavior against historical patterns. Automated incident response mechanisms take immediate action, reducing security vulnerabilities.
Eq.2.Streaming Data Processing and Anomaly Detection
Challenges and Limitations of Cloud-Based AI
Despite its advantages, cloud-based AI faces several challenges that organizations must address:
Latency and Bandwidth Constraints – Real-time data processing requires low-latency networks, but cloud-based AI may experience delays due to data transmission over the internet. Edge computing solutions help mitigate this issue.
Data Privacy and Security Risks – Processing sensitive data in the cloud raises concerns about data breaches and compliance with regulations such as GDPR and HIPAA. Organizations must implement strong encryption and access controls.
Cost and Resource Management – Cloud AI services can be expensive, especially when handling large volumes of real-time data. Businesses must optimize resource usage to control costs.
Model Interpretability and Bias – AI decision-making models must be transparent and unbiased to ensure fairness and accountability in real-time applications. Ongoing model audits and bias detection techniques are essential.
Future of Cloud-Based AI for Real-Time Decision Making
The future of cloud-based AI will be shaped by advancements in edge AI, federated learning, and quantum computing.
Edge AI for Ultra-Low Latency – AI models running on edge devices will enable near-instantaneous decision-making without relying on cloud servers.
Federated Learning for Privacy-Preserving AI – AI models will be trained across decentralized data sources without exposing sensitive information, enhancing security and privacy.
Quantum Computing for Advanced AI Processing – Quantum computing will accelerate AI algorithms, enabling faster and more complex decision-making in real-time applications.
Conclusion
Cloud-based AI is revolutionizing real-time data processing and decision-making across industries, from healthcare and finance to manufacturing and transportation. By leveraging scalable cloud infrastructure and AI-driven analytics, organizations can enhance efficiency, reduce risks, and drive innovation. However, challenges related to latency, security, and cost must be addressed to maximize AI’s potential. As emerging technologies like edge AI and federated learning continue to evolve, cloud-based AI will play an increasingly critical role in shaping the future of real-time decision-making.
4o
Subscribe to my newsletter
Read articles from Ravi Kumar Vankayalpati directly inside your inbox. Subscribe to the newsletter, and don't miss out.
Written by
