Event-Driven Data Pipelines: Kafka vs. RabbitMQ vs. Snowflake for Backend Data Flow:-

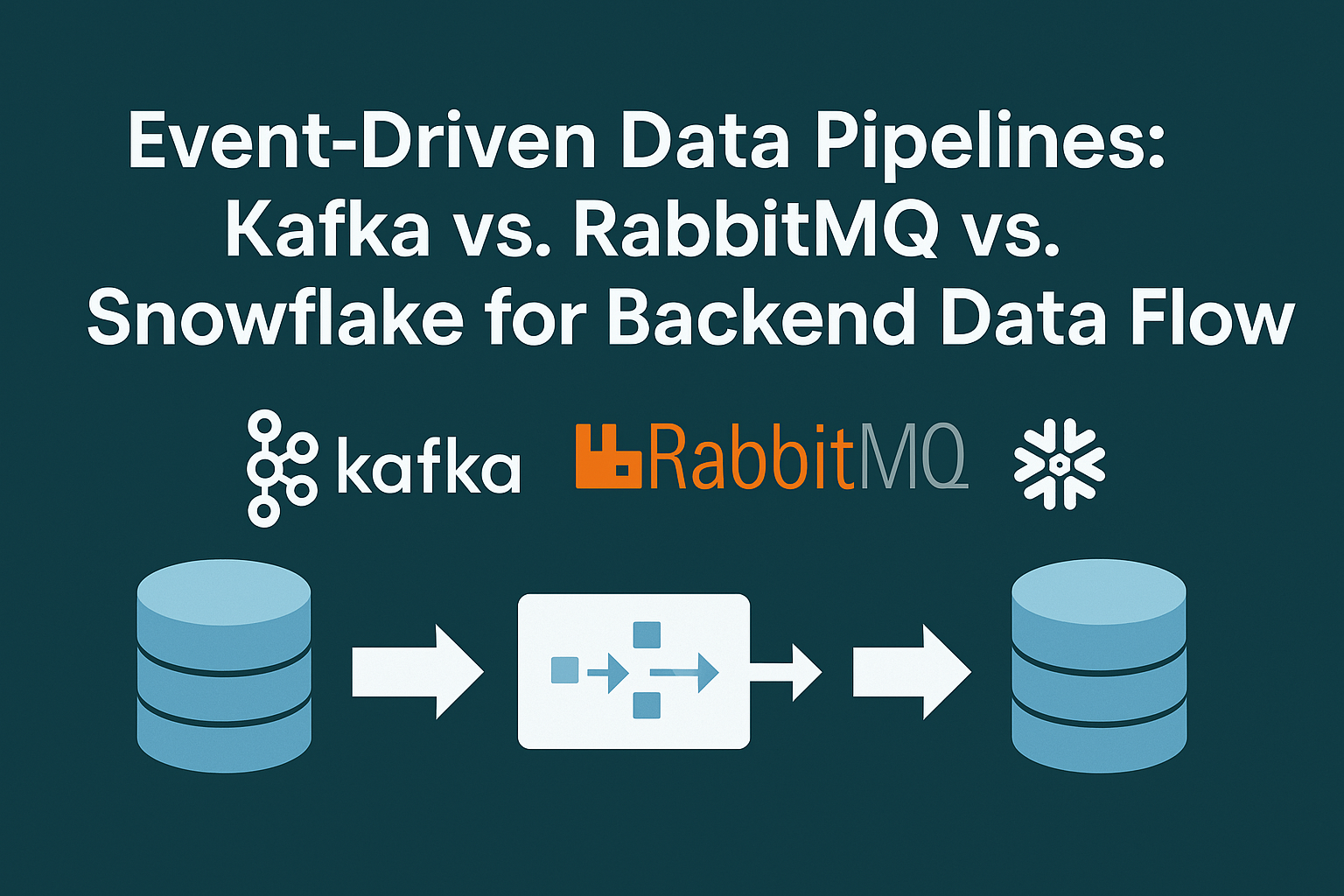
In modern backend architectures, data pipelines play a crucial role in processing and transmitting data efficiently. Event-driven architectures, powered by message brokers like Apache Kafka, RabbitMQ, and cloud data platforms like Snowflake, provide real-time data processing and scalability. This blog explores their use cases, comparisons, and how to choose the right one for your backend data pipeline.
What Are Event-Driven Data Pipelines?
Event-driven data pipelines enable real-time data flow by triggering actions in response to events. These pipelines are particularly useful in:
Real-time analytics (e.g., website activity tracking, IoT sensor data processing)
Fraud detection systems
Log aggregation and monitoring
Data ingestion into warehouses like Snowflake
Order processing in e-commerce applications
Unlike batch-based pipelines (ETL), event-driven pipelines (ELT) process data as it arrives, reducing latency and improving responsiveness.
Snowflake: The Cloud Data Platform for ELT
What is Snowflake?
Snowflake is a cloud-based data warehouse designed for scalable, real-time data processing. Unlike Kafka and RabbitMQ, which handle message passing, Snowflake provides a centralized data lake for analytics and storage.
How Snowflake Works in Event-Driven Pipelines?
Kafka Connect Snowflake Sink: Streams data from Kafka topics into Snowflake.
RabbitMQ to Snowflake: Uses ETL tools like Apache Nifi or AWS Glue to move data.
Snowpipe: A Snowflake service that ingests streaming data in near real-time.
✅ Large-scale data warehousing (structured & semi-structured data) ✅ Real-time analytics (e.g., BI tools, dashboards) ✅ ELT transformation (using Snowflake’s SQL-based processing) ✅ AI/ML workloads (storing and processing training data)
Pros of Snowflake
✔️ Scalable, cloud-native architecture ✔️ Low-maintenance, automatic scaling ✔️ Supports semi-structured data (JSON, Parquet, Avro) ✔️ Integration with Kafka, RabbitMQ, and other ETL tools
Cons of Snowflake
❌ Not a real-time event-streaming system (needs ingestion services) ❌ Cost considerations for high-volume data ingestion ❌ Requires proper indexing and optimization for large datasets
Apache Kafka: The Real-Time Streaming Giant
What is Kafka?
Apache Kafka is a distributed event streaming platform designed for high-throughput, fault-tolerant, and real-time data processing.
How Kafka Works?
Kafka consists of:
Producers: Send events (messages) to topics.
Topics: Logical channels where data is published.
Brokers: Distribute messages among consumers.
Consumers: Read messages from topics.
Zookeeper: Manages Kafka cluster metadata.
Use Cases of Kafka in Data Pipelines
✅ Log collection and monitoring (e.g., ELK stack integration) ✅ Streaming data processing (e.g., real-time user activity tracking) ✅ Data ingestion into Snowflake via Kafka Connect ✅ Distributed event-driven microservices communication
Pros of Kafka
✔️ High throughput and scalability (handles millions of events per second) ✔️ Distributed and fault-tolerant ✔️ Strong ecosystem (Kafka Connect, Schema Registry, Kafka Streams) ✔️ Ideal for large-scale event-driven architectures
Cons of Kafka
❌ Complex to set up and manage (requires Zookeeper, tuning, etc.) ❌ Higher storage and memory usage ❌ Not ideal for low-latency transactional messaging
RabbitMQ: The Reliable Message Broker
What is RabbitMQ?
RabbitMQ is a message broker that follows the Advanced Message Queuing Protocol (AMQP) and is widely used for event-driven microservices and backend queues.
How RabbitMQ Works?
Producers send messages to exchanges.
Exchanges route messages to queues based on rules (direct, fanout, topic, headers).
Queues store messages until they are processed.
Consumers retrieve messages and process them.
Use Cases of RabbitMQ in Data Pipelines
✅ Asynchronous job execution (e.g., background tasks, notifications) ✅ Message-driven microservices (e.g., processing orders in e-commerce) ✅ Work queue management (e.g., task distribution among multiple workers) ✅ Integration with Snowflake through RabbitMQ adapters
Pros of RabbitMQ
✔️ Simple to deploy and use ✔️ Low latency for small messages ✔️ Supports multiple messaging patterns (pub/sub, work queues, RPC, etc.) ✔️ Strong support for transactions and message acknowledgment
Cons of RabbitMQ
❌ Lower throughput than Kafka (limited message size and speed) ❌ Not designed for high-volume streaming data ❌ Requires additional persistence mechanisms for long-term storage
Kafka vs. RabbitMQ vs. Snowflake: Which One to Choose?
Feature | Kafka | RabbitMQ | Snowflake |
Best For | Large-scale event streaming | Message queuing & transactional messaging | Data warehousing & analytics |
Throughput | High | Medium | High |
Latency | Medium | Low | Medium |
Persistence | Log-based storage | Queue-based storage | Cloud storage |
Scalability | Very high | Moderate | High |
Ease of Setup | Moderate (Zookeeper needed) | Easy (AMQP protocol) | Easy (Managed cloud service) |
Use Cases | Real-time analytics, IoT, log processing | Asynchronous job execution, microservices | Big data storage, ELT, BI reporting |
When to Choose Kafka?
If you need high-throughput streaming data processing.
When integrating real-time analytics or IoT applications.
For event-driven microservices that handle large-scale messages.
When to Choose RabbitMQ?
If you need low-latency, reliable messaging for microservices.
When handling task queues and background jobs.
For synchronous message acknowledgment in applications.
When to Choose Snowflake?
If you need long-term storage and ELT transformations.
When working with BI tools and analytics dashboards.
For integrating Kafka or RabbitMQ data into a data warehouse.
Conclusion
Choosing between Kafka, RabbitMQ, and Snowflake depends on your backend’s requirements:
Use Kafka for high-volume, real-time event streaming.
Use RabbitMQ for reliable message delivery and task queues.
Use Snowflake for data warehousing and analytical processing.
For many applications, a combination of Kafka → Snowflake or RabbitMQ → Snowflake can provide both real-time processing and data storage benefits. 🚀
Let us know how you're using event-driven architectures in your projects!
Thank You!
Subscribe if you like it!
Subscribe to my newsletter
Read articles from Mayank agrawal directly inside your inbox. Subscribe to the newsletter, and don't miss out.
Written by

Mayank agrawal
Mayank agrawal
I am a Software Engineer and Freelancer with a strong passion for learning new technologies and exploring innovative solutions. Alongside my technical expertise, I have a keen interest in management and enjoy taking on leadership roles to drive projects to successful completion.